FedRPO: Federated Relaxed Pareto Optimization for Acoustic Event Classification
ICASSP 2023 - 2023 IEEE International Conference on Acoustics, Speech and Signal Processing (ICASSP)(2023)
摘要
Performance and robustness of real-world Acoustic Event Classification (AEC) solutions depend on ability to train on diverse data from wide range of end-point devices and acoustic environments. Federated Learning (FL) provides a framework to leverage annotated and non-annotated AEC data from servers and client devices in a privacy preserving manner. In this work we propose a novel Federated Relaxed Pareto Optimization (FedRPO) method for semi-supervised FL with heterogeneous client data. In contrast to federated averaging class of FL algorithms (fedAvg) that perform unconstrained weighted aggregation across all data sources, FedRPO enables special treatment of data with high quality annotations vs. data with pseudo-labels of unknown, varying qualities. In particular, FedRPO computes the updates to the global model solving a constrained linear program, with explicit Pareto constraints to prevent performance degradation on annotated data, and controlled relaxation of the Pareto constraints on pseudo-labeled data to prevent learning of patterns in conflict with the annotated data. We show FedRPO significantly outperforms FedAvg on Amazon internal de-identified dataset on AEC tasks. On supervised learning, FedRPO improved precision by 32.5% over FedAvg when maintaining recall at 90%. Combined with FixMatch [1] for semi-supervised learning, FedRPO outperformed FedAvg on precision by 50.5% at 90% recall.
更多查看译文
关键词
Acoustic Event Classification,Federated Learning,Pareto Optimization,Semi-supervised Learning
AI 理解论文
溯源树
样例
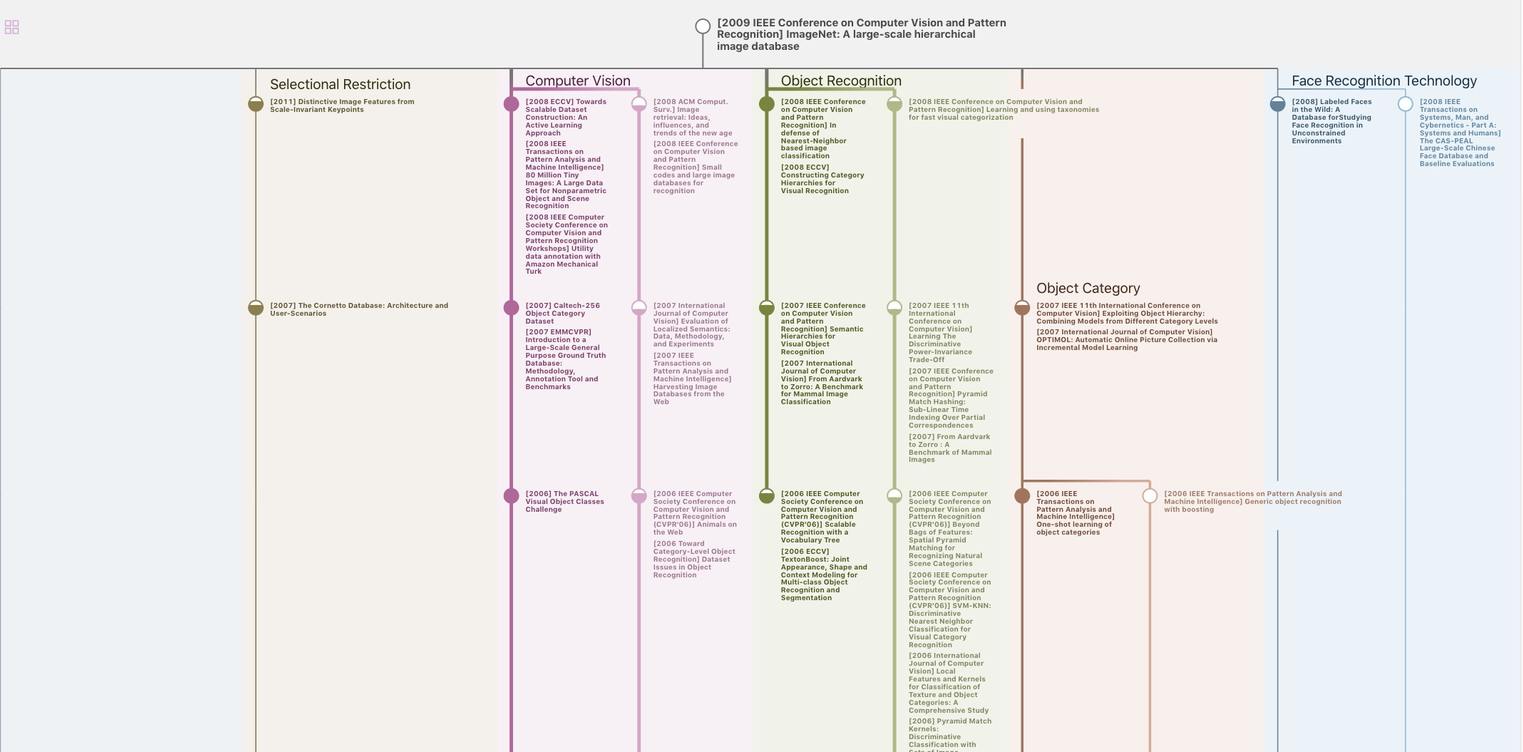
生成溯源树,研究论文发展脉络
Chat Paper
正在生成论文摘要