Distill-Quantize-Tune - Leveraging Large Teachers for Low-Footprint Efficient Multilingual NLU on Edge
ICASSP 2023 - 2023 IEEE International Conference on Acoustics, Speech and Signal Processing (ICASSP)(2023)
摘要
This paper describes Distill-Quantize-Tune (DQT), a pipeline to create viable small-footprint multilingual models that can perform NLU on extremely resource-constrained Edge devices. We distill semantic knowledge from a large-sized teacher (transformer-based), that has been trained on huge amount of public and private data, into our Edge candidate (student) model (Bi-LSTM based) and further compress the student model using a lossy quantization method. We show that unlike monolingual models, in a multilingual scenario, post-compression finetuning on downstream tasks is not enough to recover the performance loss caused by compression. We design a fine-tuning pipeline to recover the lost performance using a compounded loss function consisting of NLU, distillation and compression losses. We show that pre-biasing the encoder with semantics learned on a language modeling task can further improve the performance when used in conjunction with DQT pipeline. Our best performing multilingual model achieves a size reduction of 85% and 99.2% when compared to uncompressed student and teacher models respectively. It outperforms the uncompressed monolingual models (by >30% on average) across all languages on our in-house data. We further validate our approach and see similar trends on the public MultiATIS++ dataset.
更多查看译文
关键词
Bi-LSTM based,compounded loss function,Distill-Quantize-Tune - leveraging,distillation,downstream tasks,DQT pipeline,Edge candidate model,extremely resource-constrained Edge devices,fine-tuning pipeline,language modeling task,large-sized teacher,lossy quantization method,lost performance,low-footprint efficient multilingual NLU,multilingual scenario,performing multilingual model,post-compression finetuning,private data,public data,semantic knowledge,semantics,size reduction,student model,teacher models,uncompressed monolingual models,uncompressed student,viable small-footprint multilingual models
AI 理解论文
溯源树
样例
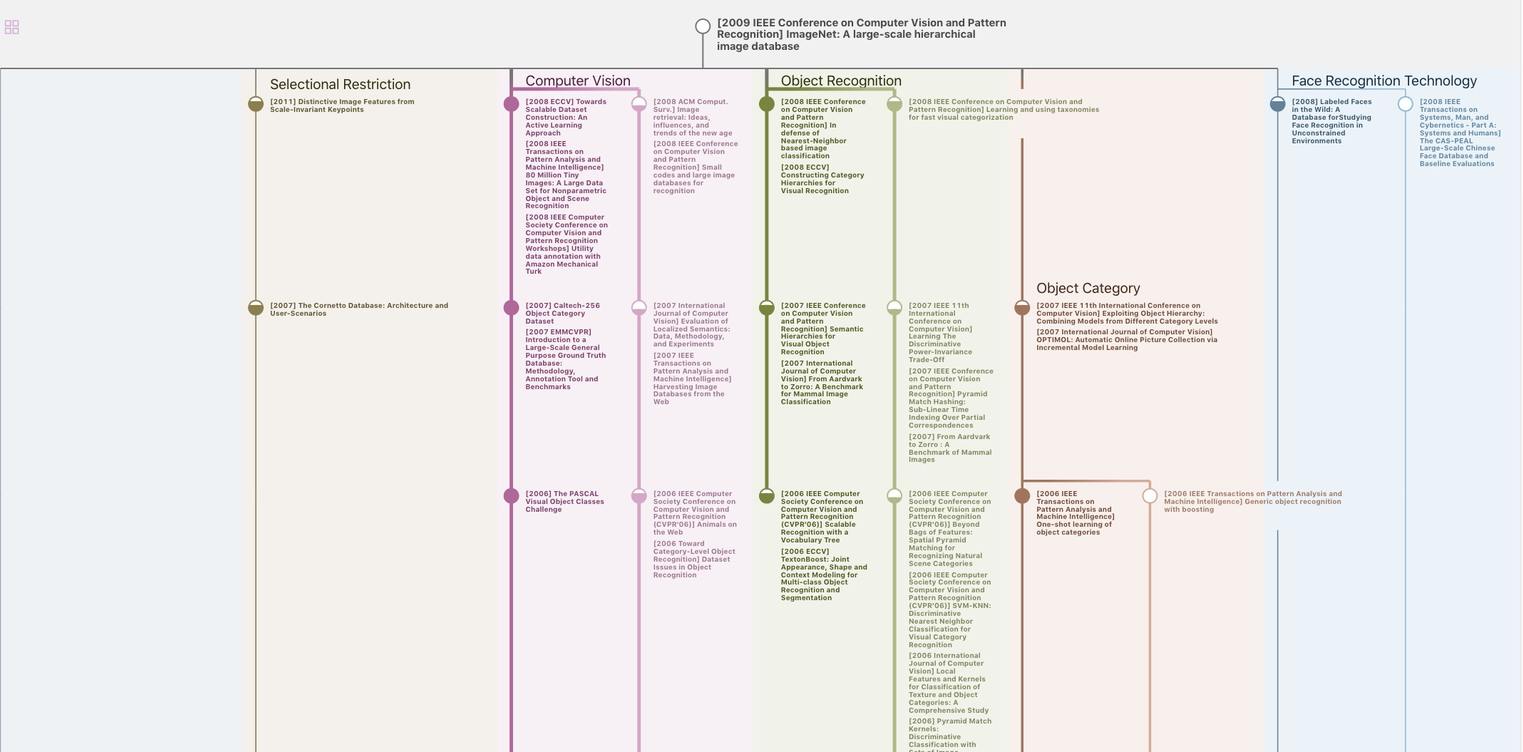
生成溯源树,研究论文发展脉络
Chat Paper
正在生成论文摘要