Dual-Uncertainty Guided Curriculum Learning and Part-Aware Feature Refinement for Domain Adaptive Person Re-Identification
ICASSP 2023 - 2023 IEEE International Conference on Acoustics, Speech and Signal Processing (ICASSP)(2023)
摘要
Unsupervised Domain Adaptative person re-identification (UDA ReID) aims to transfer the knowledge of pre-trained model from labeled source domain to unlabeled target domain. Although the current clustering-based methods have achieved promising success, they neglect the tolerance of the model to cope with different-level noise, which may cause the model to memorize some incorrect patterns caused by label noise and overfit on them rapidly in the early stages. In this paper, we introduce a novel Dual Uncertainty guided Curriculum Learning (DUCL) method to tackle the above problems. Specifically, the reliability-based curriculum allocation is proposed to enforce the sample adaptation in an easy-to-hard manner, which is further assisted by a novel dual-uncertainty re-weighting strategy to alleviate the influence of label noise. In addition, we design Part-aware Feature Refinement (PAFR) to enhance the discrimination of model and thereby acquiring more reliable pseudo-labels. Specifically, the part-aware attention maps are exploited in the PAFR to integrate fine-grained semantics into holistic representation. Extensive experiments have validated the superiority of the proposed method.
更多查看译文
关键词
Label noise,Curriculum learning,Dual-uncertainty,Feature refinement
AI 理解论文
溯源树
样例
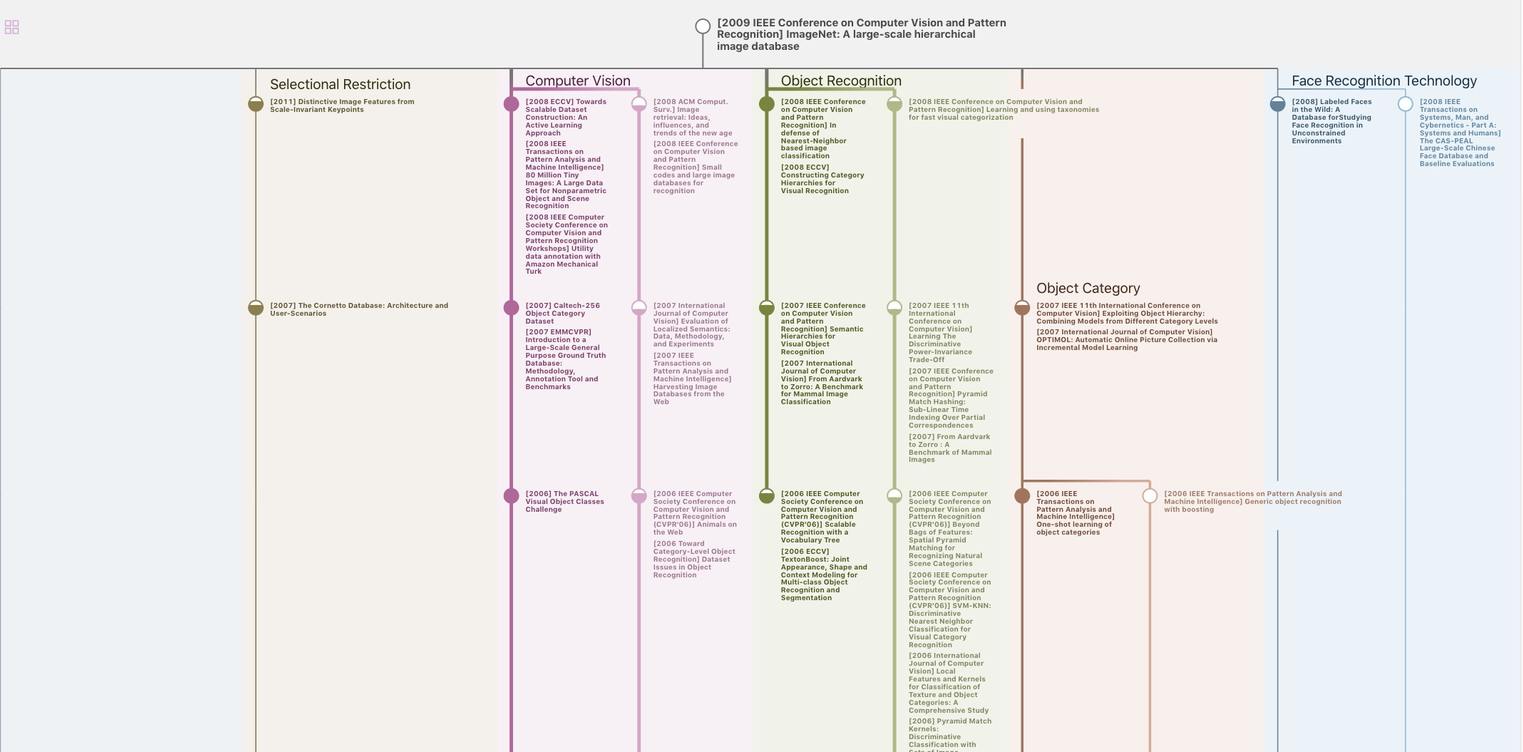
生成溯源树,研究论文发展脉络
Chat Paper
正在生成论文摘要