Topology Uncertainty Modeling For Imbalanced Node Classification on Graphs
ICASSP 2023 - 2023 IEEE International Conference on Acoustics, Speech and Signal Processing (ICASSP)(2023)
摘要
Most existing graph neural networks work under a class-balanced assumption, while ignoring class-imbalanced scenarios that widely exist in real-world graphs. Although there are many methods in other fields that can alleviate this issue, they do not consider the special topology of the non-Euclidean graph. Hence, we propose Graph Topology Uncertainty (GraphTU), a novel probabilistic class-imbalanced solution specifically for graphs. Firstly, an invisible "uncertain gap" between under-represented minorities in training set and authentic minorities in unseen set is modeled by estimating statistical variances in topology. We extend the training distribution for minorities by sampling in this gap through a non-parametric way. Moreover, a gradient-guided mask is introduced to prevent biased statistics. Extensive experiments demonstrate the superior performance of GraphTU.
更多查看译文
关键词
Class Imbalance,Node Classification
AI 理解论文
溯源树
样例
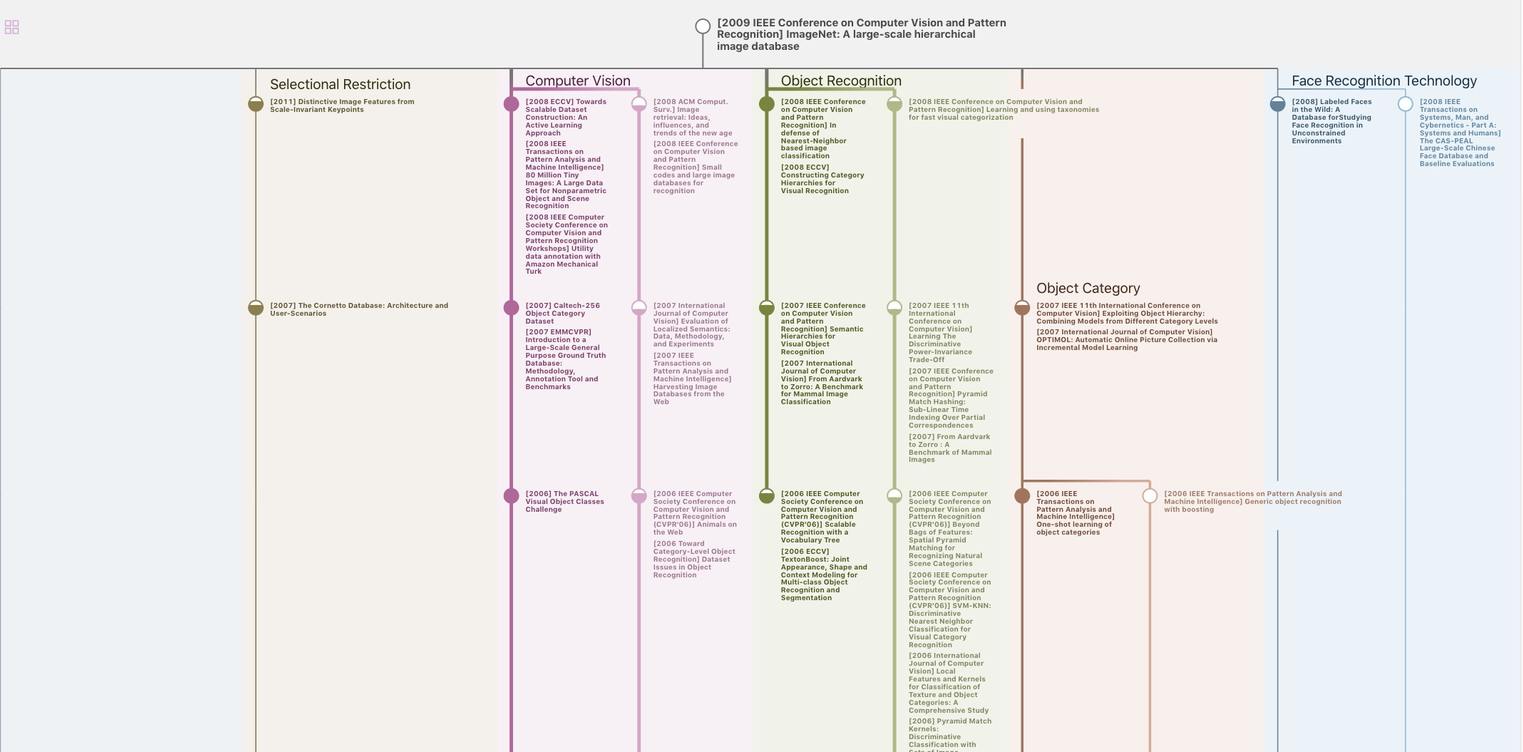
生成溯源树,研究论文发展脉络
Chat Paper
正在生成论文摘要