Sparse Convolution Based Octree Feature Propagation for Lidar Point Cloud Compression
ICASSP 2023 - 2023 IEEE International Conference on Acoustics, Speech and Signal Processing (ICASSP)(2023)
摘要
With the advent of new 3D scanning technologies, point clouds have become a crucial way to depict real and virtual objects/scenes. Point clouds represent the continuous sur-faces of underlying object/scene through a collection (usually millions) of discrete, irregular, and often sparsely distributed 3D samples on the surface of the objects, e.g. LiDAR scans. This nature of point cloud data presents a considerable challenge to not only store but also understand and extract the topology of object(s) from the point cloud data. In this regard, our work presents a point cloud compression procedure that leverages sparse 3D convolutions to extract features at various octree scales for lossless compression of octree representation of point clouds. For hierarchical flow of information between octree levels, our proposed method named SparseContextNet (SCN) also propagates features from a lower resolution scale to higher resolution scale via 3D upsampling convolutions. Our experiments with LiDAR datasets reveal competitive performance of our proposal compared to the state-of-the-art.
更多查看译文
关键词
Point cloud compression,point cloud geometry,deep learning,octree compression
AI 理解论文
溯源树
样例
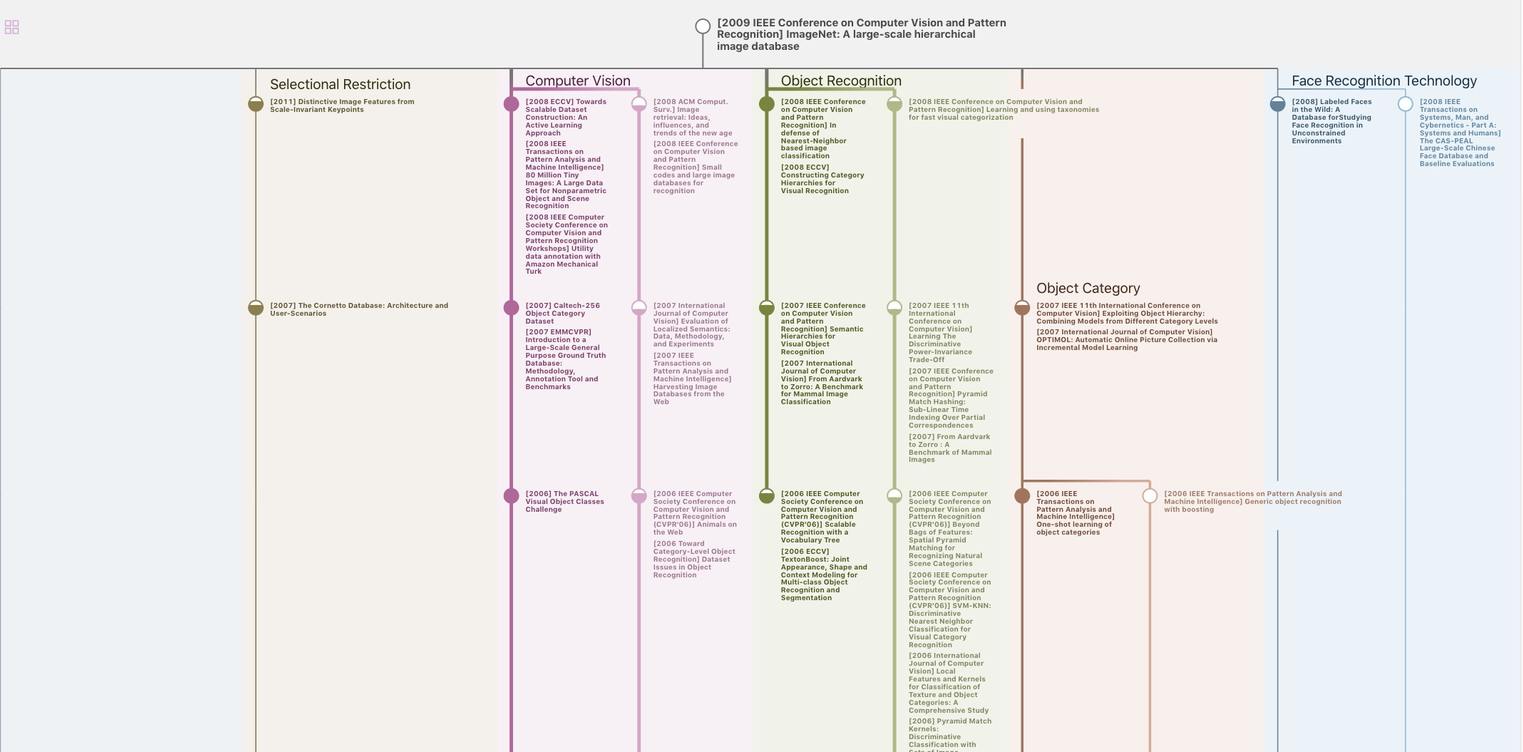
生成溯源树,研究论文发展脉络
Chat Paper
正在生成论文摘要