MFAT: A Multi-Level Feature Aggregated Transformer for Person Re-Identification
ICASSP 2023 - 2023 IEEE International Conference on Acoustics, Speech and Signal Processing (ICASSP)(2023)
摘要
Recently, with the development of the Transformer, re-identification (ReID) has great success in various applications. Existing works prefer to utilize the Transformer’s highest-level information as its discriminative feature, which focuses on a few concentrated parts or areas. However, in ReID filed, under such various scenes and camera views, only using a few concentrated parts to distinguish the query person is insufficient. Meanwhile, we find that Transformer’s lower-level information is also helpful for the recognition accuracy of the query person, especially, when the scene changes greatly. Therefore, we propose a Multi-level Feature Aggregated Transformer for person re-identification (MFAT) with high performance. To aggregate multi-level information, two novel modules are carefully designed. (i) The Global Content and Structure Aggregation (GCSA) module is proposed to aggregate multi-level information in a global manner. (ii) The Local Convolution Aggregation (LCA) module which consists of a series of convolutional blocks, is introduced to aggregate multi-level features with local operations. To the best of our knowledge, this is the first work to aggregate multi-level features with a Transformer backbone for person ReID task. Experiment results show that our method has achieved state-of-the-art on three person ReID benchmarks, with both Pyramid Vision Transformer (PVT) and Vision Transformer (ViT) backbones.
更多查看译文
关键词
Person ReIdentification,Transformer,Multi-level
AI 理解论文
溯源树
样例
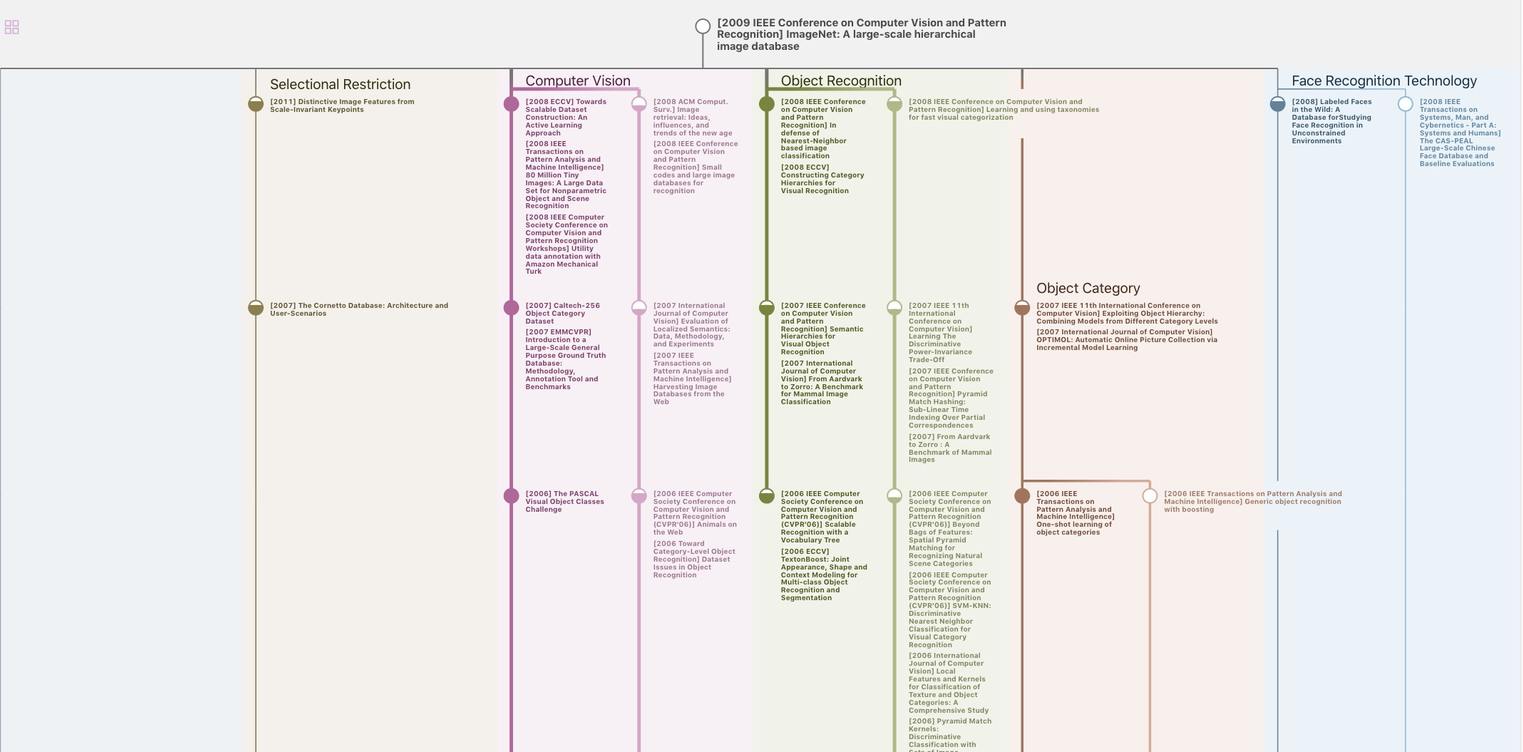
生成溯源树,研究论文发展脉络
Chat Paper
正在生成论文摘要