Boosting No-Reference Super-Resolution Image Quality Assessment with Knowledge Distillation and Extension
ICASSP 2023 - 2023 IEEE International Conference on Acoustics, Speech and Signal Processing (ICASSP)(2023)
摘要
Deep learning (DL) based image super-resolution (SR) tech-niques have been well investigated for recent years. However, studies dedicated to SR image quality assessment (SR-IQA) have not been fully developed, which is even more difficult if pristine high-resolution (HR) images are lacking as a reference. Due to the challenge, existing widely used no-reference (NR) SR-IQA metrics (e.g., PI, NIQE, and Ma) are still far from meeting the practical requirements of providing accurate estimations which align well with human mean opinion scores (MOS). To this end, we propose a novel Knowledge Extension Super-Resolution Image Quality Assessment (KE-SR-IQA) framework to predict SR image quality by leveraging a semi-supervised knowledge distillation (KD) strategy. Concretely, we first employ a well-trained full-reference (FR) SR-IQA model as the teacher, then we perform knowledge extension (KE) by additional pseudo-labeled data to further distill a NR-student for promoting the prediction accuracy. Extensive experiments on several benchmarks validate the ef-fectiveness of our approach.
更多查看译文
关键词
Super-resolution image quality assessment (SR-IQA),no-reference (NR),knowledge distillation (KD),knowledge extension (KE)
AI 理解论文
溯源树
样例
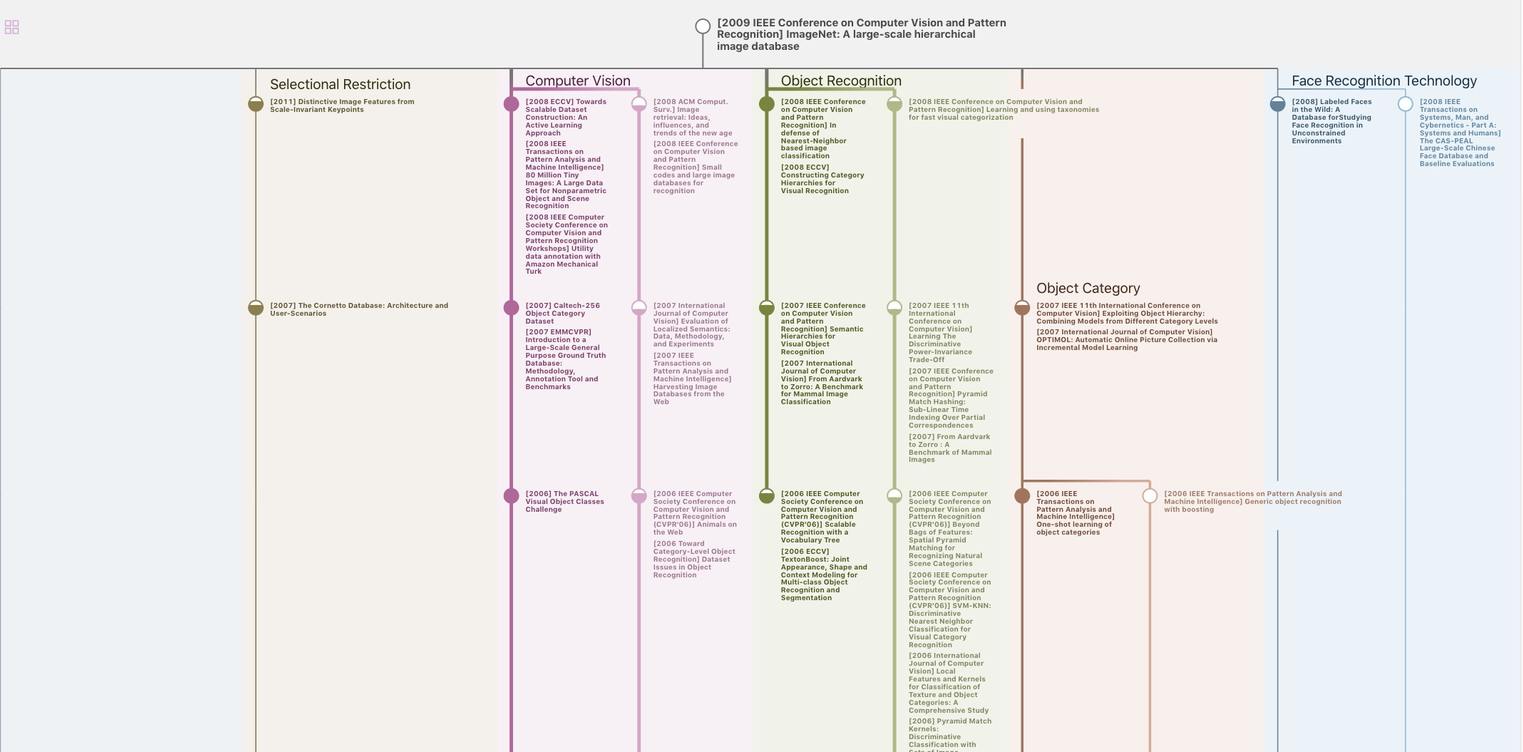
生成溯源树,研究论文发展脉络
Chat Paper
正在生成论文摘要