Mendam: Multi-Expert Network with Distribution-Aware Momentum for Long-Tailed Recognition
ICASSP 2023 - 2023 IEEE International Conference on Acoustics, Speech and Signal Processing (ICASSP)(2023)
摘要
Long-tailed data distribution (i.e., minority classes occupy most of the data, while most classes have very few samples) is a common problem in image classification. The existing deferred re-balancing methods suffer from low accuracy for tail classes due to their bias towards head classes. In this paper, we find that properly adjusting the momentum can significantly improve the performance for class-imbalanced tasks, which provides a novel perspective to solve this thorny problem. Based on this finding, we propose a new way to calculate a distribution-aware momentum (DAM) based on the data distribution to avoid bias towards head classes. Furthermore, we design a multi-expert network (MEN) to designate each expert to focus on different tail classes. We achieve new state-of-the-art on CIFAR-10-LT, CIFAR-100-LT, ImageNet-LT and iNaturalist2018 for image classification.
更多查看译文
关键词
Long-tailed learning,Distribution-aware momentum,Multi-expert network
AI 理解论文
溯源树
样例
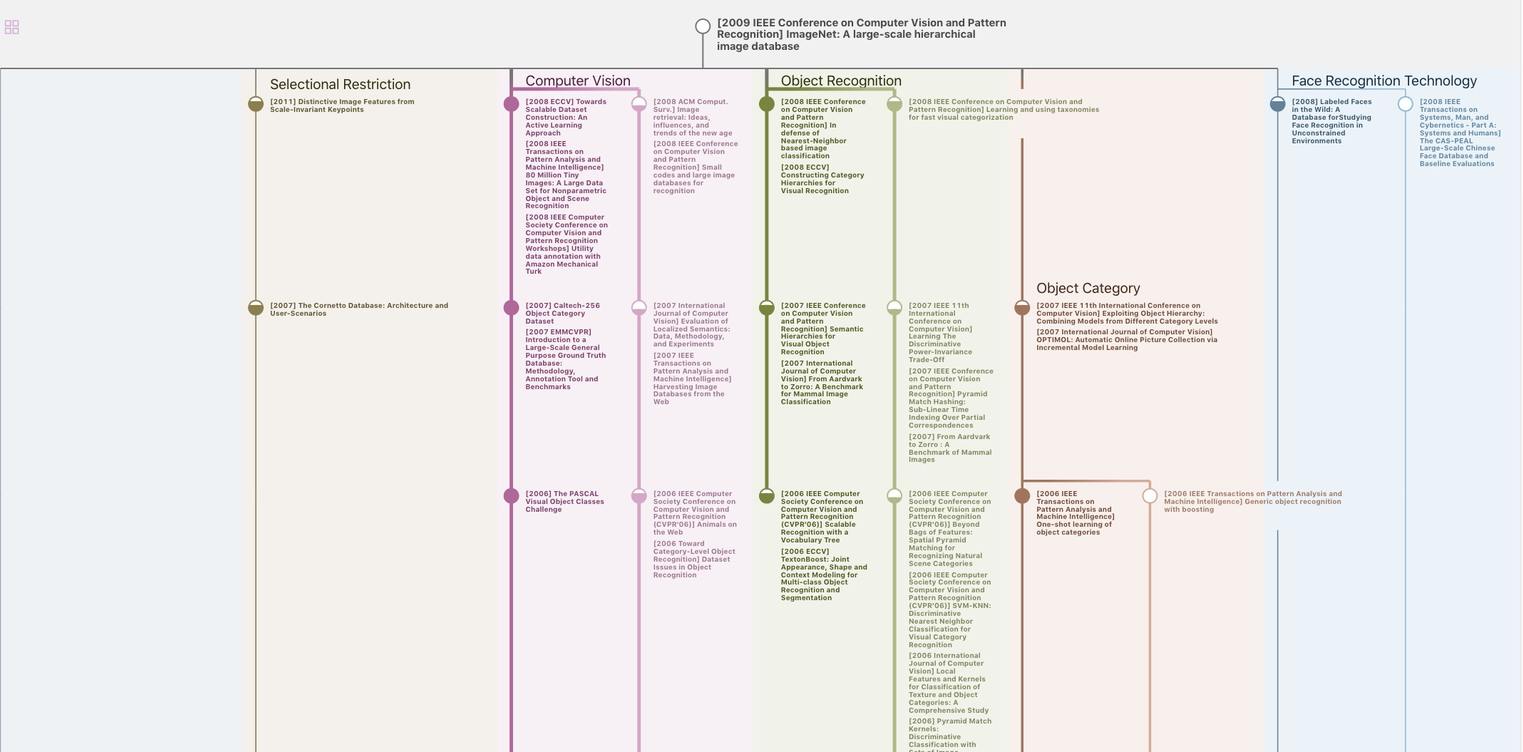
生成溯源树,研究论文发展脉络
Chat Paper
正在生成论文摘要