Inverse Reinforcement Learning with Graph Neural Networks for IoT Resource Allocation
ICASSP 2023 - 2023 IEEE International Conference on Acoustics, Speech and Signal Processing (ICASSP)(2023)
摘要
The rapid development of Internet of Things (IoT) applications requires efficient computing and communication resource allocation strategies to streamline the existing network operations. These strategies could be formulated as mixed-integer nonlinear programming (MINLP) problems, where the optimal branch-and-bound (B&B) with the full strong branching (FSB) variable selection policy features an extremely high complexity. We propose inverse reinforcement learning with graph neural networks (GNNIRL) to generate a new variable selection policy that closely matches the FSB variable selection. Without sacrificing the optimality, the GNNIRL can directly infer the variable selection with a significantly lower complexity, which is also verified by simulation.
更多查看译文
关键词
communication resource allocation strategies,FSB variable selection,full strong branching,GNNIRL,graph neural networks,Internet of Things applications,inverse reinforcement learning,IoT resource allocation,MINLP,mixed-integer nonlinear programming problems,optimal branch- branch-and-bound
AI 理解论文
溯源树
样例
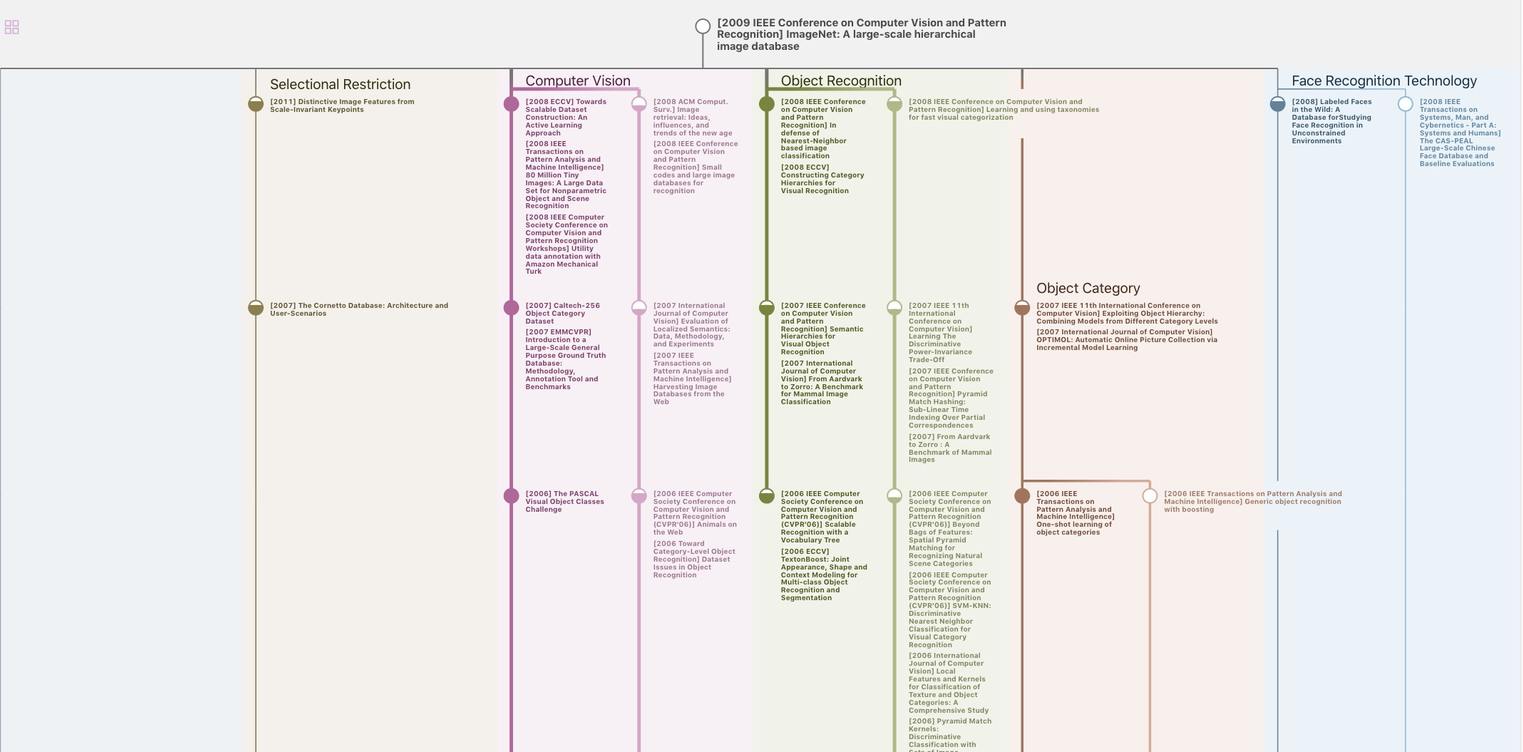
生成溯源树,研究论文发展脉络
Chat Paper
正在生成论文摘要