Multi-Level Fusion for Burst Super-Resolution with Deep Permutation-Invariant Conditioning
ICASSP 2023 - 2023 IEEE International Conference on Acoustics, Speech and Signal Processing (ICASSP)(2023)
摘要
Developing deep learning techniques for super-resolving bursts of images acquired by mobile cameras is a topic that has recently gained significant interest. This topic fits the general problem of learning-based multi-image super-resolution (SR), which, contrary to its sibling single-image SR, has so far received little attention despite its potential. In this work, we introduce a neural network architecture for burst SR, called MLB-FuseNet (Multi-Level Burst Fusion Network), that is capable of extracting features in a manner that is invariant to permutations in the burst and to progressively condition features extracted from a reference image. Permutation invariance is desirable as it is known that the order of images in a burst does not matter in this problem, but its study has so far been neglected. Moreover, we also introduce a module exploiting a polyphase decomposition to improve feature extraction from mosaiced raw images. Results show an improvement over the state of the art on the BurstSR dataset – a recent and popular benchmark for this problem.
更多查看译文
关键词
burst super-resolution,convolutional neural networks,multi-level fusion,self-attention,demosaicing
AI 理解论文
溯源树
样例
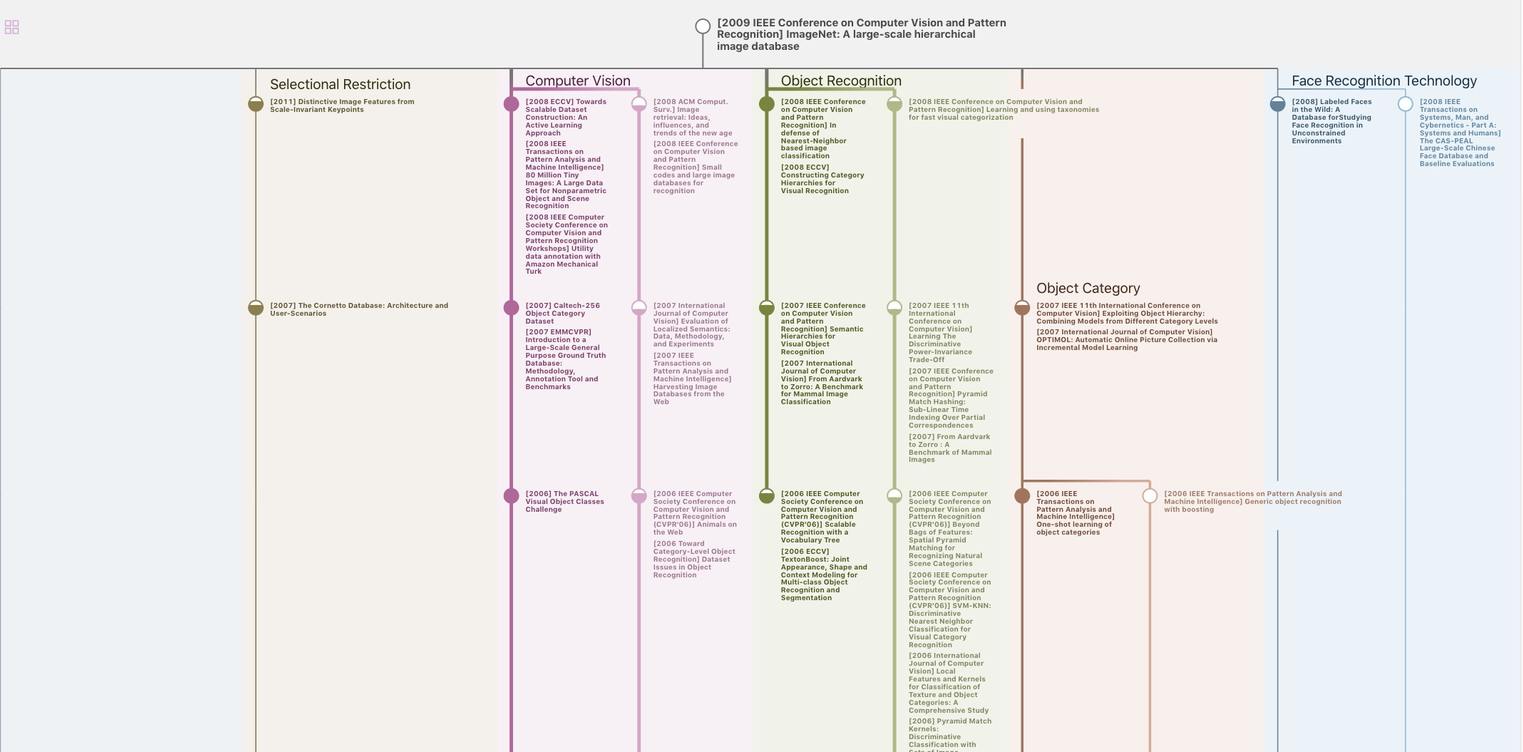
生成溯源树,研究论文发展脉络
Chat Paper
正在生成论文摘要