A Graph Neural Network Multi-Task Learning-Based Approach for Detection and Localization of Cyberattacks in Smart Grids
ICASSP 2023 - 2023 IEEE International Conference on Acoustics, Speech and Signal Processing (ICASSP)(2023)
Abstract
False data injection attacks (FDIAs) on smart power grids’ measurement data present a threat to system stability. When malicious entities launch cyberattacks to manipulate the measurement data, different grid components will be affected, which leads to failures. For effective attack mitigation, two tasks are required: determining the status of the system (normal operation/under attack) and localizing the attacked bus/power substation. Existing mitigation techniques carry out these tasks separately and offer limited detection performance. In this paper, we propose a multi-task learning-based approach that performs both tasks simultaneously using a graph neural network (GNN) with stacked convolutional Chebyshev graph layers. Our results show that the proposed model presents superior system status identification and attack localization abilities with detection rates of 98.5−100% and 99 − 100%, respectively, presenting improvements of 5 − 30% compared to benchmarks.
MoreTranslated text
Key words
Multi-task learning,localization,false data injection attacks,cyberattacks,smart grids
AI Read Science
Must-Reading Tree
Example
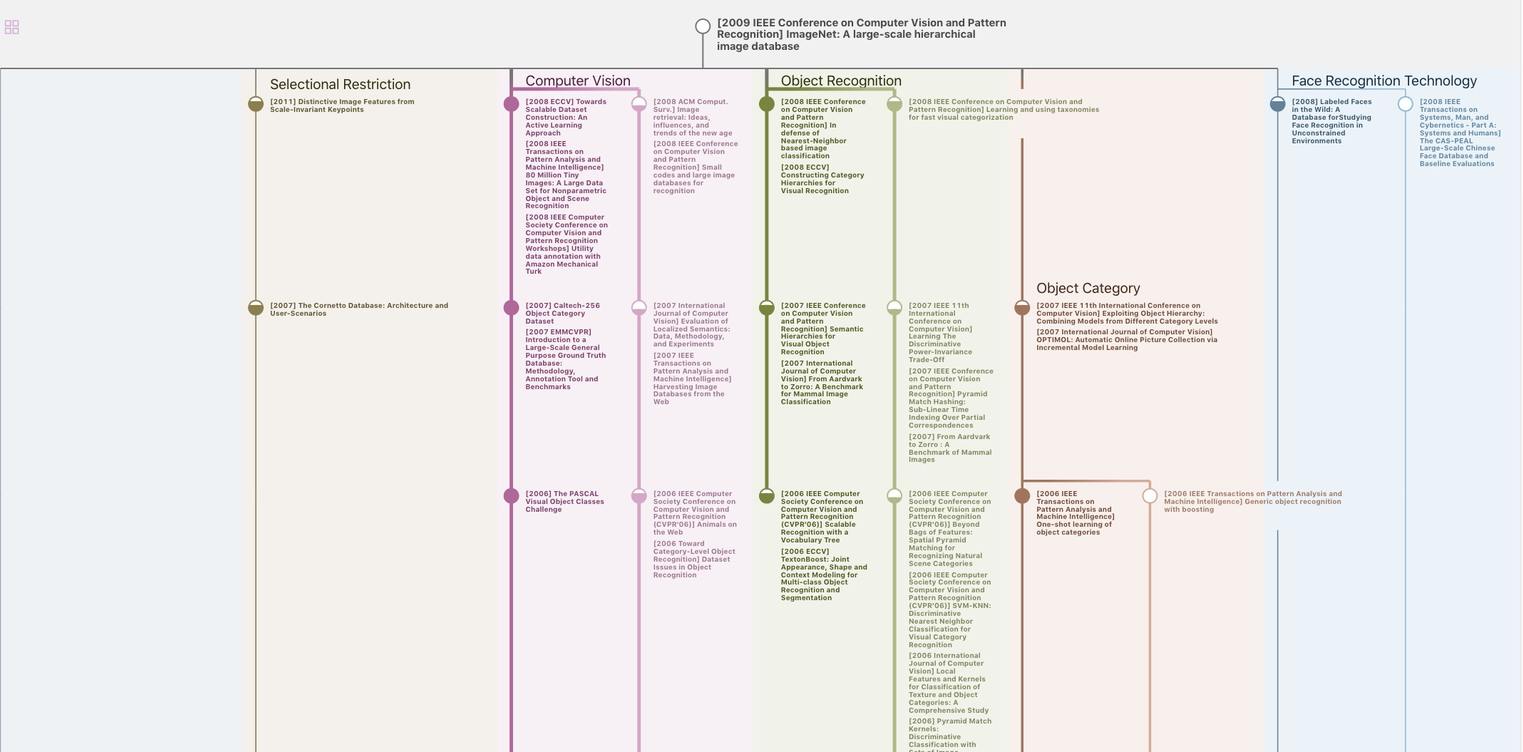
Generate MRT to find the research sequence of this paper
Chat Paper
Summary is being generated by the instructions you defined