Exploiting Sparse Recovery Algorithms for Semi-Supervised Training of Deep Neural Networks for Direction-of-Arrival Estimation
ICASSP 2023 - 2023 IEEE International Conference on Acoustics, Speech and Signal Processing (ICASSP)(2023)
摘要
This paper proposes a semi-supervised training approach for a direction-of-arrival (DoA) estimation based on a convolutional neural network (CNN). We apply a sparse recovery algorithm called optMGD-ℓ
1
-SVD on the training dataset consisting of only unlabeled observed data to obtain binarized pseudo-spectra regarded as the CNN training targets (labels). The estimated DoAs are obtained at test time by performing peak picking on the CNN outputs. optMGD-ℓ
1
-SVD has been shown to perform well with a few sensors under low signal-to-noise ratio (SNR) conditions (up to −6 dB) by optimally reweighting the pseudo-spectra of ℓ
1
-SVD based on the application of group delay function on the pseudo-spectra of MUSIC. Since its hyperparameters are noise-sensitive, we assume that the SNR levels of the training dataset are known such that we can use the optimal ones. We also consider multi-condition training using data of multiple SNR levels to improve the robustness towards different noisy environments. We evaluated the trained networks, named optMGD-ℓ
1
-SVD-CNN and MGD-ℓ
1
-SVD-CNN, in terms of the average root-mean-square error and the resolution probability under low SNR conditions (up to −20 dB). We demonstrated that it performed well with a few sensors and snapshots, including at SNR levels unseen in the training data.
更多查看译文
关键词
direction-of-arrival estimation,convolutional neural network,semi-supervised training,MUSIC,optMGD-ℓ1-SVD
AI 理解论文
溯源树
样例
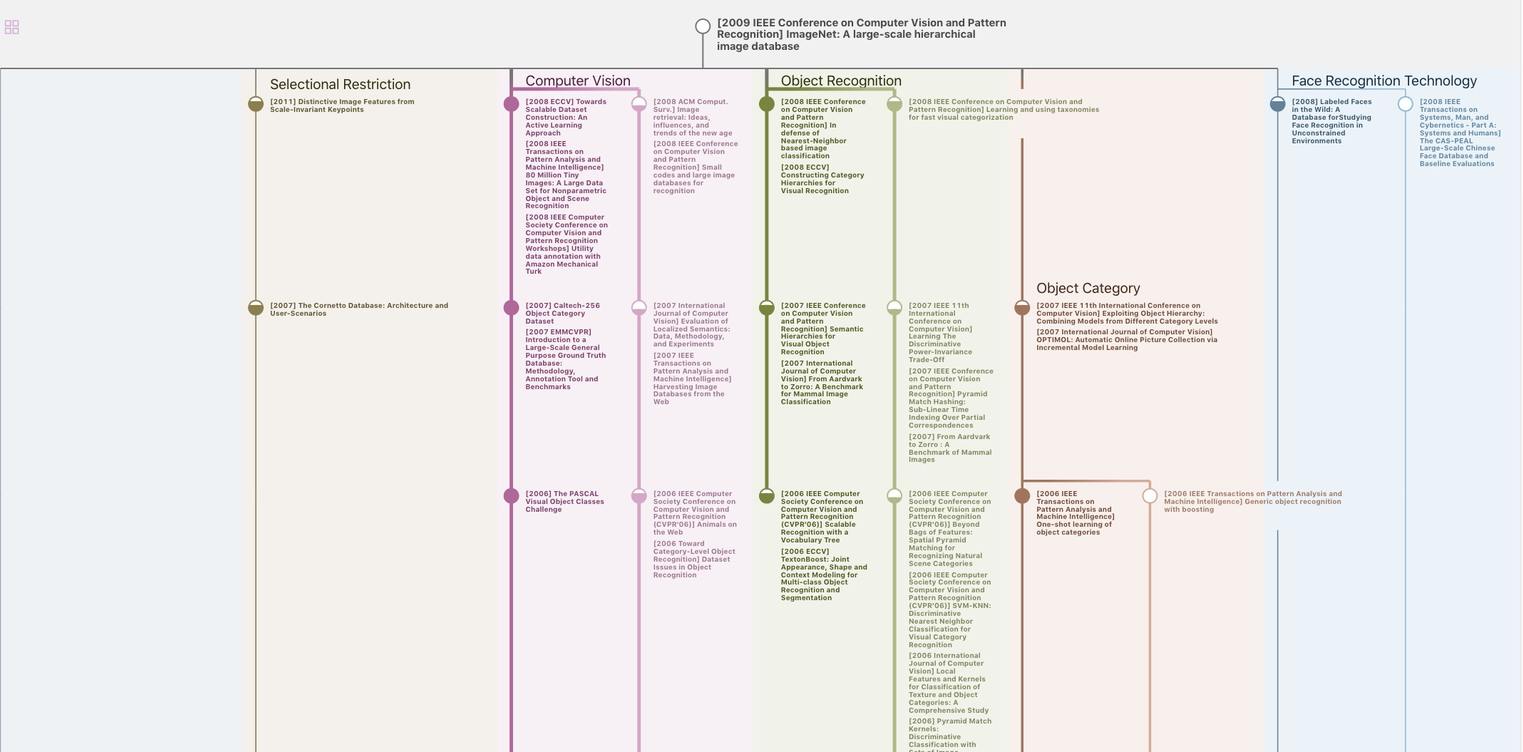
生成溯源树,研究论文发展脉络
Chat Paper
正在生成论文摘要