Interpretable Multi-Scale Neural Network for Granger Causality Discovery
ICASSP 2023 - 2023 IEEE International Conference on Acoustics, Speech and Signal Processing (ICASSP)(2023)
摘要
We propose a novel multi-scale neural network for Granger causality discovery (MSNGC) in multivariate time series. Compared with existing counterparts, our model avoids the explicit data segmentation between series and between time lags for the first time. By extracting diverse causal information from the data with different delay ranges and then integrating them effectively via the learned attention weights, it can capture the complete causal relationships between all the series and provide accurate weighted adjacency matrix estimation. Thus, further interpretable inference can be better supported. Specifically, we propose a consistency-based thresholding algorithm for binary causal structure inference, an effect sign detection method to distinguish the positive causal effects from the negative ones, and a self-adaptive lag discovery algorithm to identify the lagged time points. Experiments on multiple benchmarks demonstrate that our model significantly outperforms the state-of-the-art methods.
更多查看译文
关键词
Multi-scale neural network,Granger causality,interpretability,causal effect sign,lag discovery
AI 理解论文
溯源树
样例
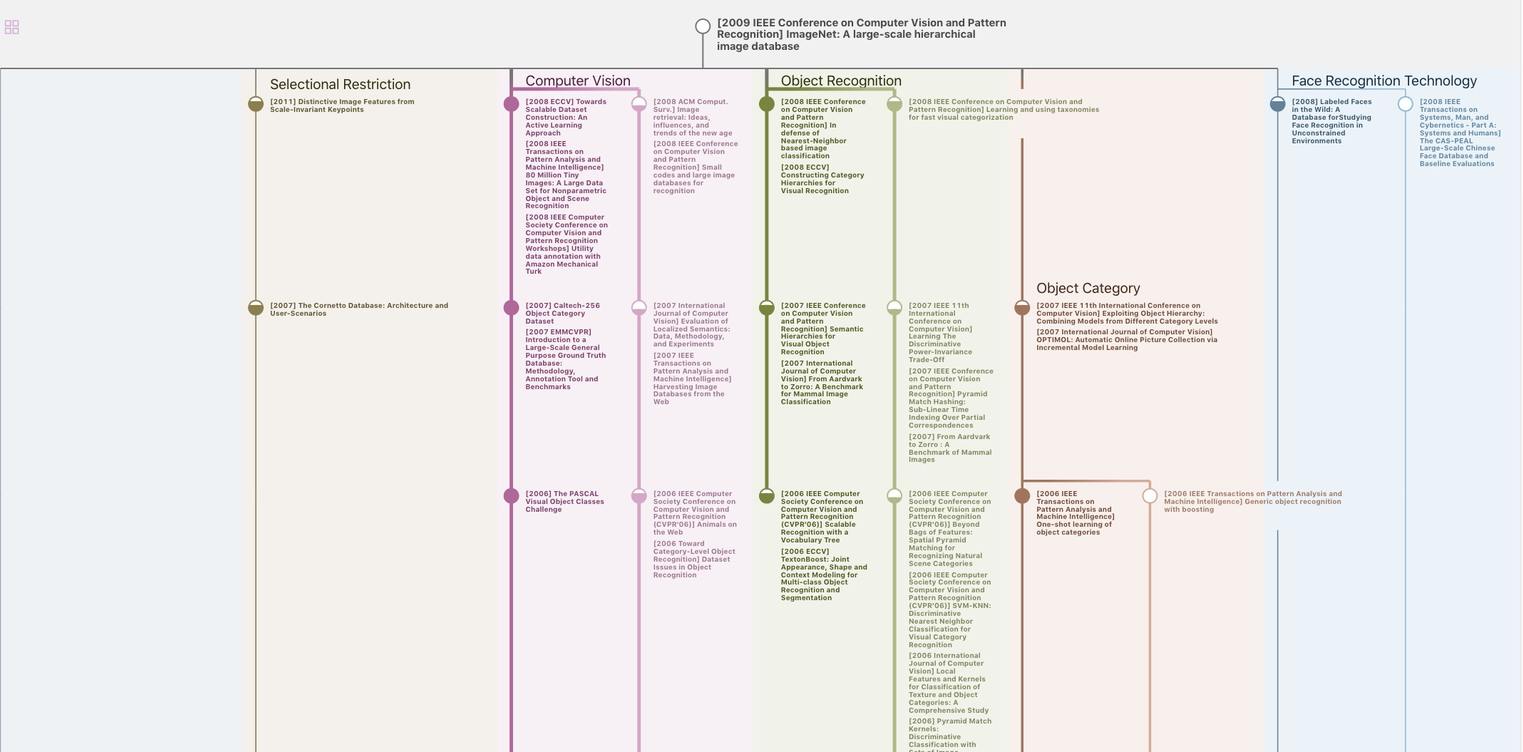
生成溯源树,研究论文发展脉络
Chat Paper
正在生成论文摘要