Contrastive Learning of Sentence Embeddings in Product Search
IEEE International Conference on Acoustics, Speech, and Signal Processing(2023)
关键词
Weak Supervision,Contrastive Learning,Graph Neural Network,Domain-specific Pretraining
AI 理解论文
溯源树
样例
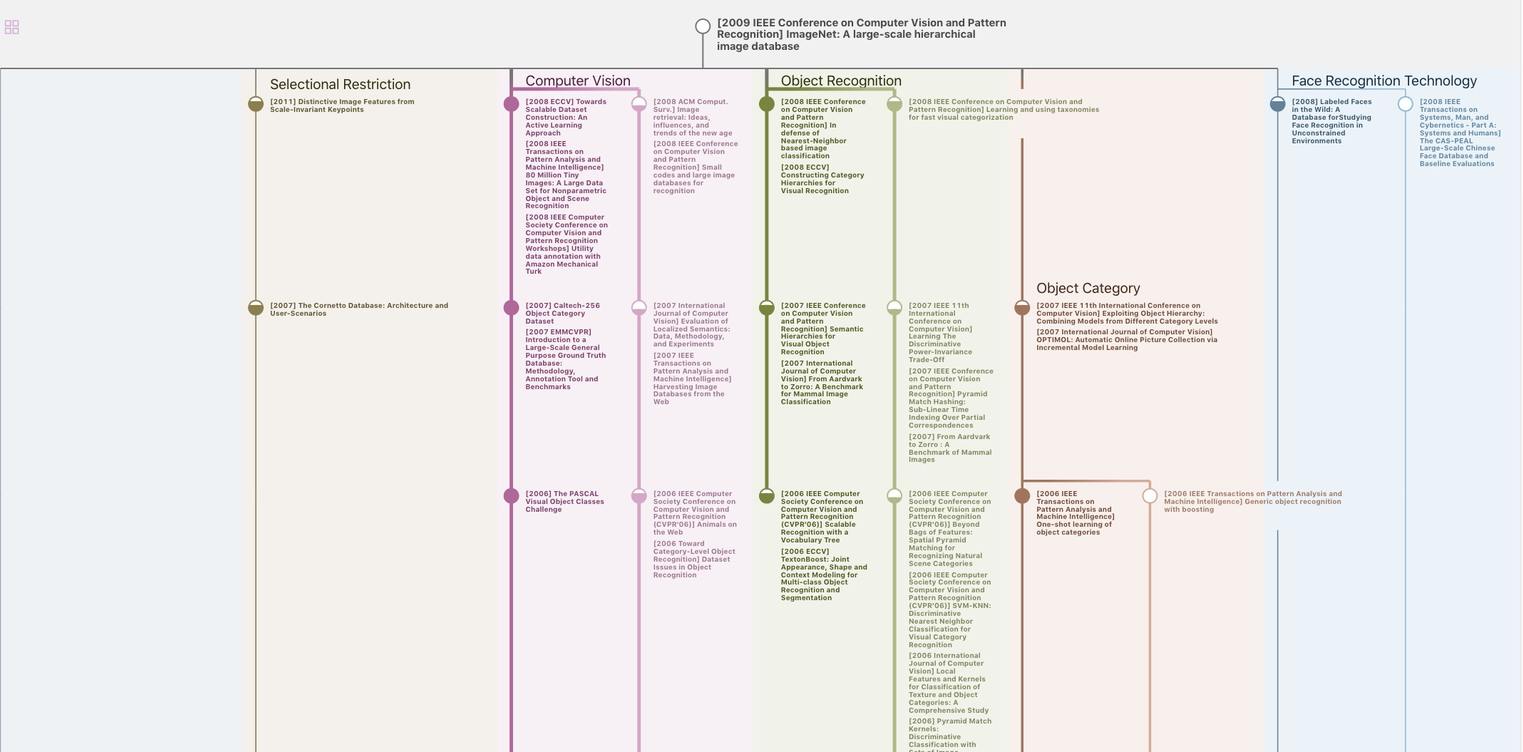
生成溯源树,研究论文发展脉络
Chat Paper
正在生成论文摘要