CPA: Compressed Private Aggregation for Scalable Federated Learning Over Massive Networks
ICASSP 2023 - 2023 IEEE International Conference on Acoustics, Speech and Signal Processing (ICASSP)(2023)
摘要
Federated learning (FL) allows a central server to train a model using remote users’ data. FL faces challenges in preserving the local datasets privacy and in its communication overhead; which is considerably dominant in large-scale networks. These limitations are often mitigated individually by local differential privacy (LDP) mechanisms, compression, and user-selection techniques, which often come at the cost of accuracy. In this work we present compressed private aggregation (CPA), which allows massive deployments to simultaneously communicate at extremely low bit-rates while achieving privacy, anonymity, and resilience to malicious users. CPA randomizes a code-book for compressing the data into a few bits, ensuring anonymity and robustness, with a subsequent perturbation to hold LDP. We provide both a theoretical analysis and a numerical study, demonstrating the performance gains of CPA compared with separate mechanisms for compression and privacy.
更多查看译文
关键词
FL,LDP,anonymity,compression
AI 理解论文
溯源树
样例
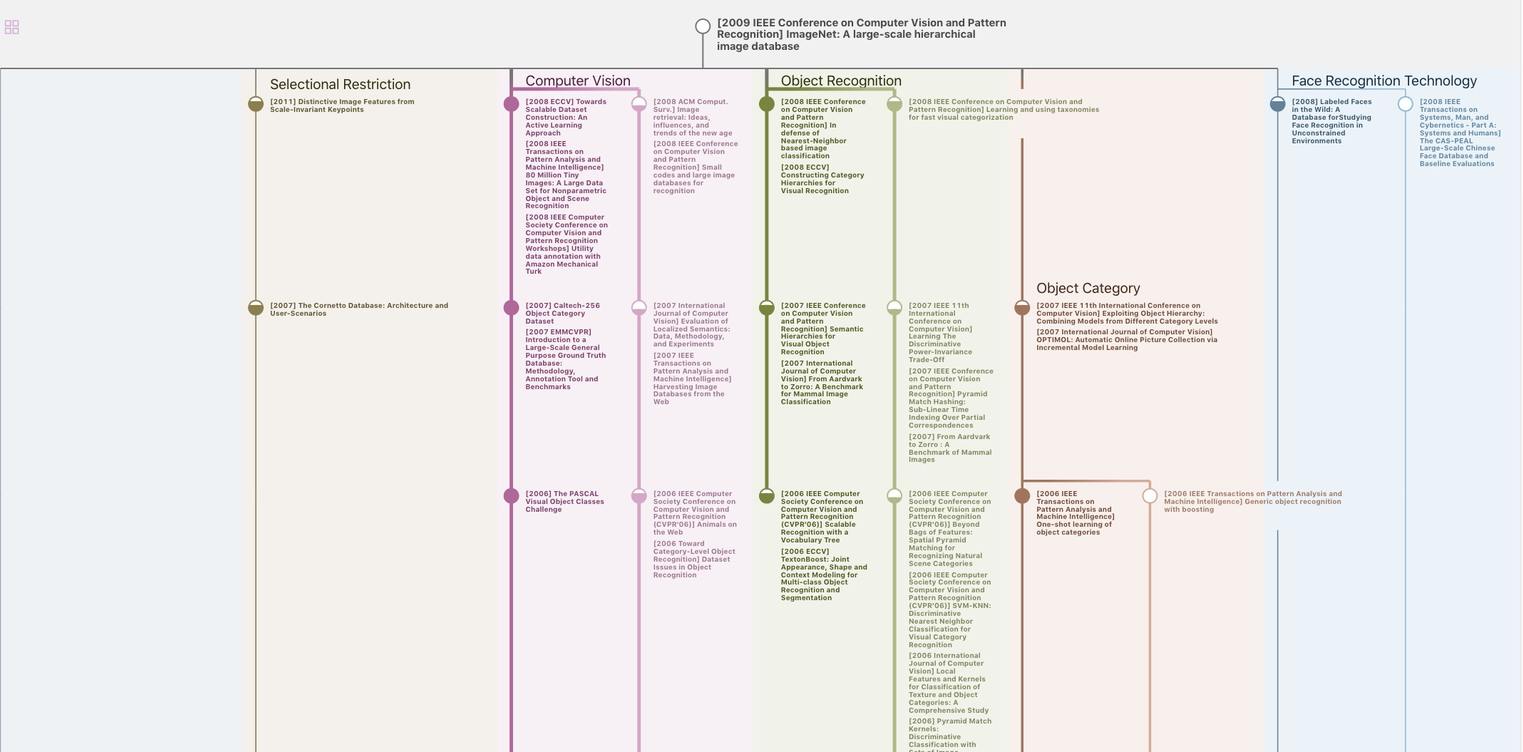
生成溯源树,研究论文发展脉络
Chat Paper
正在生成论文摘要