Super-Resolution for Macro X-Ray Fluorescence Data Collected from Old Master Paintings
ICASSP 2023 - 2023 IEEE International Conference on Acoustics, Speech and Signal Processing (ICASSP)(2023)
摘要
Macro X-ray fluorescence (MA-XRF) scanning is commonly used to non-invasively analyse Old Master paintings by mapping the distribution of the chemical elements present in the artworks. The visual quality of the element distribution maps is very important for characterising the materials and understanding the execution and condition of the painting. However, this quality is limited by the acquisition time for the XRF datacube, resulting in a trade-off between signal-to-noise ratio (SNR) and spatial resolution. To solve this problem we propose to enhance the spatial resolution of the XRF datacube of a painting leveraging a corresponding high-resolution (HR) RGB image. We achieve that by introducing a method based on coupled dictionary learning along with a similarity constraint based on mutual information. In particular, we divide the RGB image and the XRF datacube into a common part and a unique part based on whether the information is shared or not, and then transfer the HR information between the two common parts, resulting in high-quality reconstructions. Numerical results show that our XRF super-resolution method outperforms the other state-of-the-art approaches.
更多查看译文
关键词
X-ray fluorescence,super-resolution,dictionary learning,sparse representation,mutual information
AI 理解论文
溯源树
样例
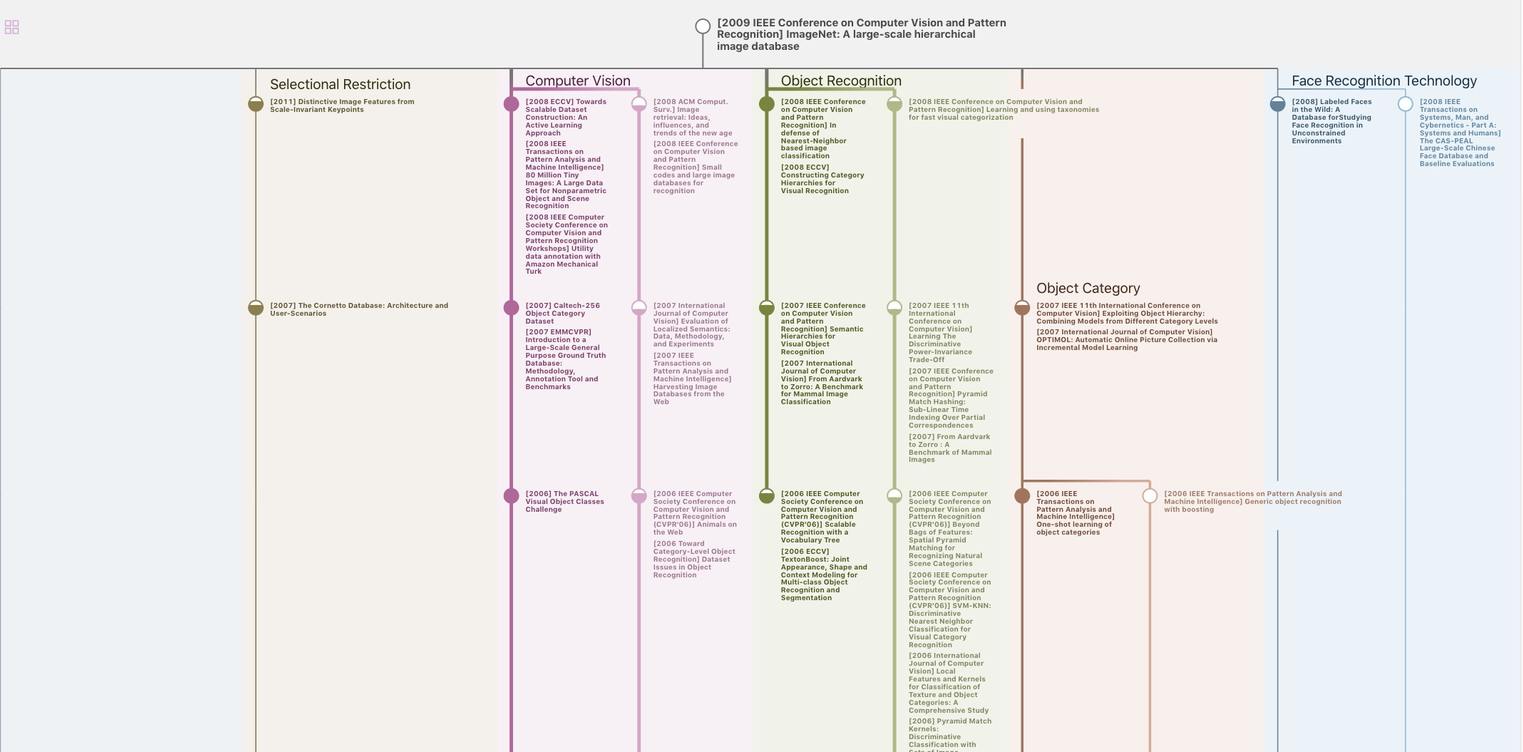
生成溯源树,研究论文发展脉络
Chat Paper
正在生成论文摘要