Long-Tailed Recognition with Causal Invariant Transformation
ICASSP 2023 - 2023 IEEE International Conference on Acoustics, Speech and Signal Processing (ICASSP)(2023)
摘要
Standard classification models rely on the assumption that all the classes of interest are equally represented in training datasets. However, visual phenomena exhibit a long-tailed distribution, such that many standard approaches fail to properly model and result in a considerable degeneration on accuracy. The recent methods have produced encouraging results, but their efforts only seek to simulate the statistical relationship between data and labels and compensate for imbalanced data-related issues, without addressing the underlying causal mechanisms. In this paper, a comprehensive structural causal model is developed to excavate the intrinsic causal mechanism between data and labels. Specifically, we assume that each input is constructed from a mix of causal factors and non-causal factors, and only the causal factors cause the classification judgments. In order to extract such causal factors from inputs and then reconstruct the invariant causal mechanisms, we propose a Causal Invariant Transformation algorithm for Long-tailed recognition (CITL), which generates diverse data to avoid the over-fitting on the tail classes and enforces the learnt representations to maintain the causal factors and eliminate the non-causal factors. Our extensive experimental results on several widely used datasets have demonstrated the effectiveness of our proposed CITL approach.
更多查看译文
关键词
Long-tailed Recognition,Deep Learning,Structural Causal Model,Causal Invariant Transformation
AI 理解论文
溯源树
样例
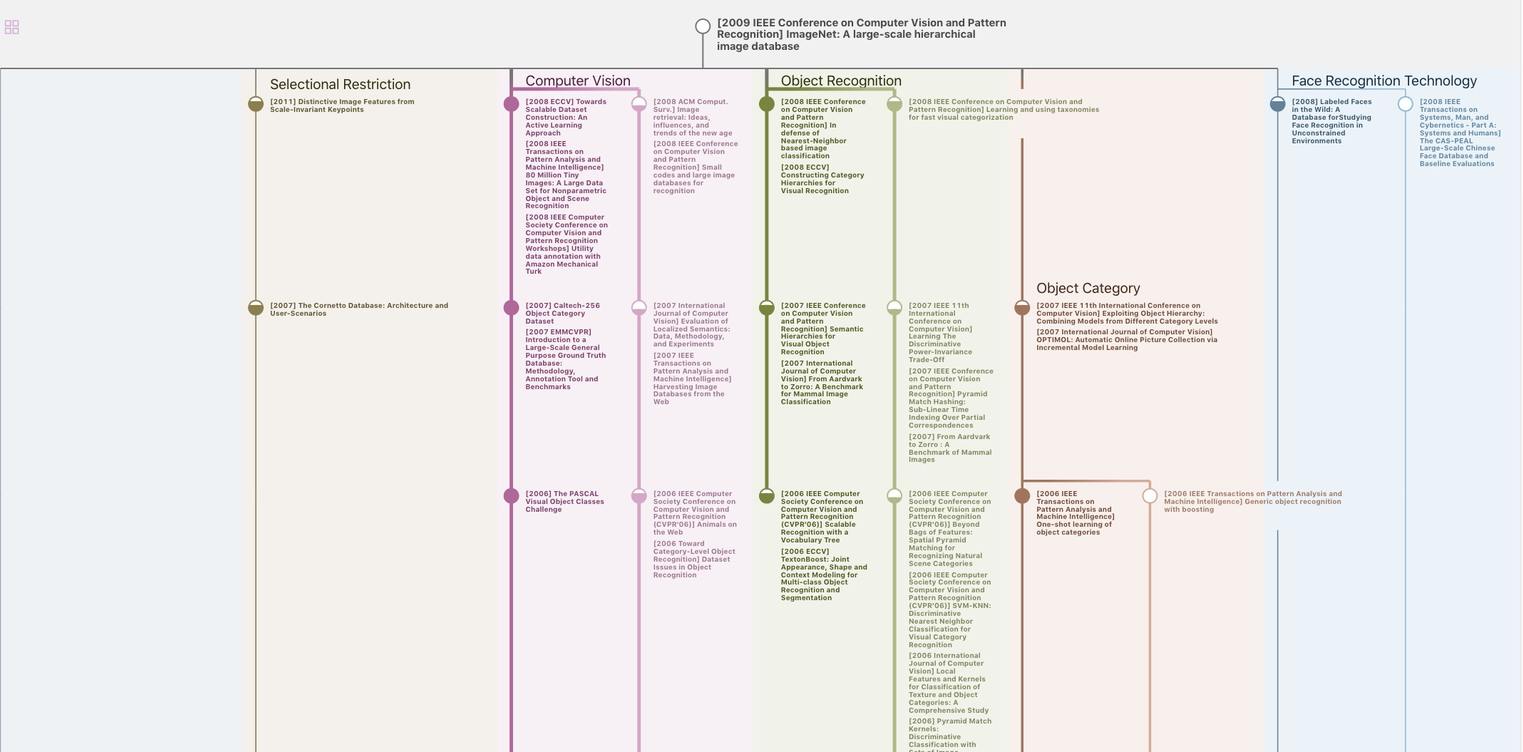
生成溯源树,研究论文发展脉络
Chat Paper
正在生成论文摘要