Leveraging Sparsity with Spiking Recurrent Neural Networks for Energy-Efficient Keyword Spotting
ICASSP 2023 - 2023 IEEE International Conference on Acoustics, Speech and Signal Processing (ICASSP)(2023)
Abstract
Bio-inspired Spiking Neural Networks (SNNs) are promising candidates to replace standard Artificial Neural Networks (ANNs) for energy-efficient keyword spotting (KWS) systems. In this work, we compare the trade-off between accuracy and energy-efficiency of a gated recurrent SNN (Spik-GRU) with a standard Gated Recurrent Unit (GRU) on the Google Speech Command Dataset (GSCD) v2. We show that, by taking advantage of the sparse spiking activity of the SNN, both accuracy and energy-efficiency can be increased. Lever-aging data sparsity by using spiking inputs, such as those produced by spiking audio feature extractors or dynamic sensors, can further improve energy-efficiency. We demonstrate state-of-the-art results for SNNs on GSCD v2 with up to 95.9% accuracy. Moreover, SpikGRU can achieve similar accuracy than GRU while reducing the number of operations by up to 82%.
MoreTranslated text
Key words
Spiking neural networks,keyword spotting,speech commands,energy-efficiency,sparsity
AI Read Science
Must-Reading Tree
Example
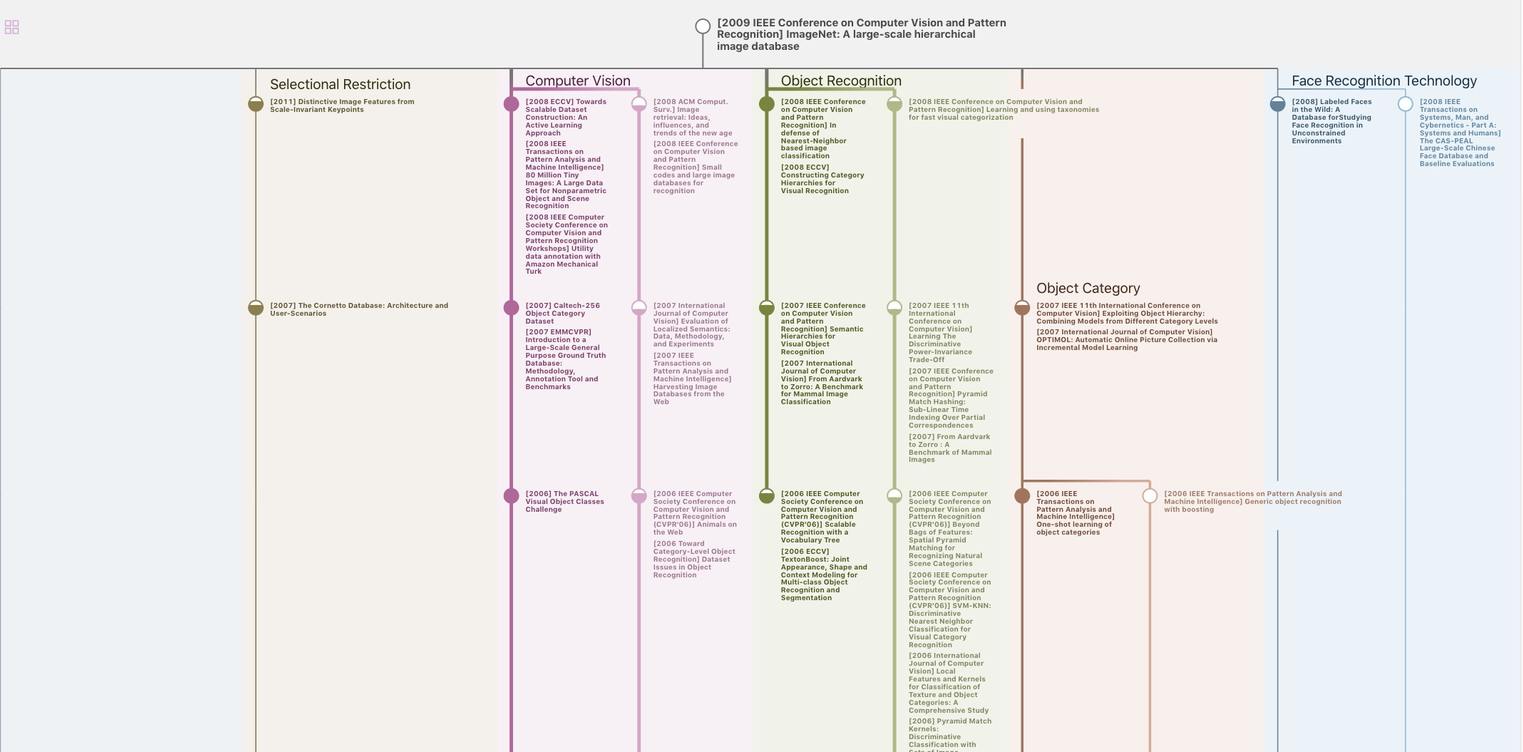
Generate MRT to find the research sequence of this paper
Chat Paper
Summary is being generated by the instructions you defined