Universal Sampling Denoising (USD) for noise mapping and noise removal of non-Cartesian MRI.
ArXiv(2023)
摘要
Random matrix theory (RMT) combined with principal component analysis has resulted in a widely used MPPCA noise mapping and denoising algorithm, that utilizes the redundancy in multiple acquisitions and in local image patches. RMT-based denoising relies on the uncorrelated identically distributed noise. This assumption breaks down after regridding of non-Cartesian sampling. Here we propose a Universal Sampling Denoising (USD) pipeline to homogenize the noise level and decorrelate the noise in non-Cartesian sampled k-space data after resampling to a Cartesian grid. In this way, the RMT approaches become applicable to MRI of any non-Cartesian k-space sampling. We demonstrate the denoising pipeline on MRI data acquired using radial trajectories, including diffusion MRI of a numerical phantom and ex vivo mouse brains, as well as in vivo $T_2$ MRI of a healthy subject. The proposed pipeline robustly estimates noise level, performs noise removal, and corrects bias in parametric maps, such as diffusivity and kurtosis metrics, and $T_2$ relaxation time. USD stabilizes the variance, decorrelates the noise, and thereby enables the application of RMT-based denoising approaches to MR images reconstructed from any non-Cartesian data. In addition to MRI, USD may also apply to other medical imaging techniques involving non-Cartesian acquisition, such as PET, CT, and SPECT.
更多查看译文
AI 理解论文
溯源树
样例
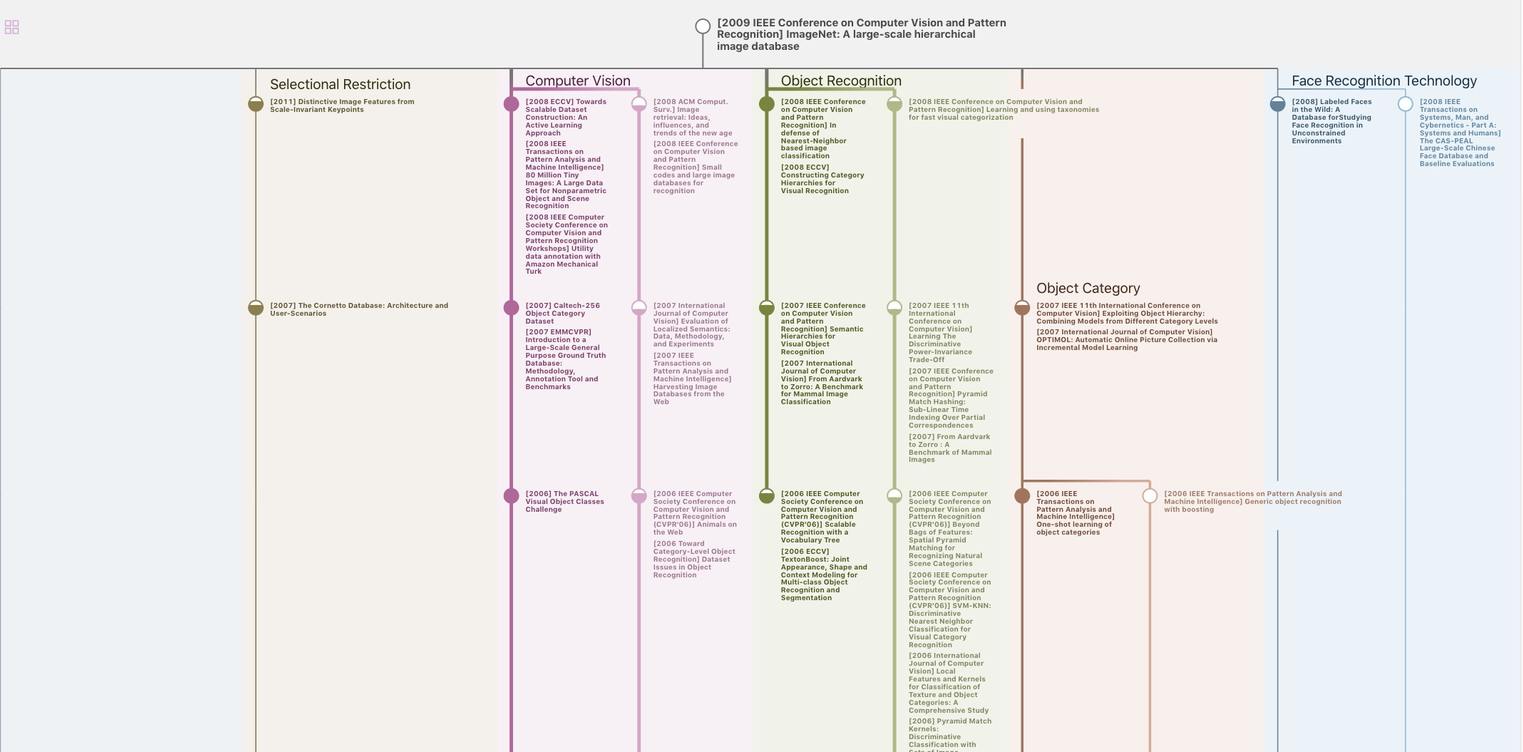
生成溯源树,研究论文发展脉络
Chat Paper
正在生成论文摘要