Effectiveness of Model and Data Scale Contrastive Learning in Non-IID Federated Learning
2023 International Conference on Advanced Technologies for Communications (ATC)(2023)
摘要
All involved clients are guaranteed data privacy in a collaborative machine learning environment via Federated Learning. The lack of generalization in local client models brought on by data heterogeneity, however, is one of Federated Learning ’s major challenges that lead to slow model convergence and communication latency. In this study, we use contrastive learning techniques that have been shown effective in both centralized and federated learning environments. For local models to have a stronger capacity for generalization, we suggest adopting contrastive loss at the model and data scales. Utilizing the CIFAR10 and CIFAR-100 datasets, we assess and contrast our suggested strategy with other industry standard approaches.
更多查看译文
关键词
Federated learning,federated optimization,non-IID,contrastive learning
AI 理解论文
溯源树
样例
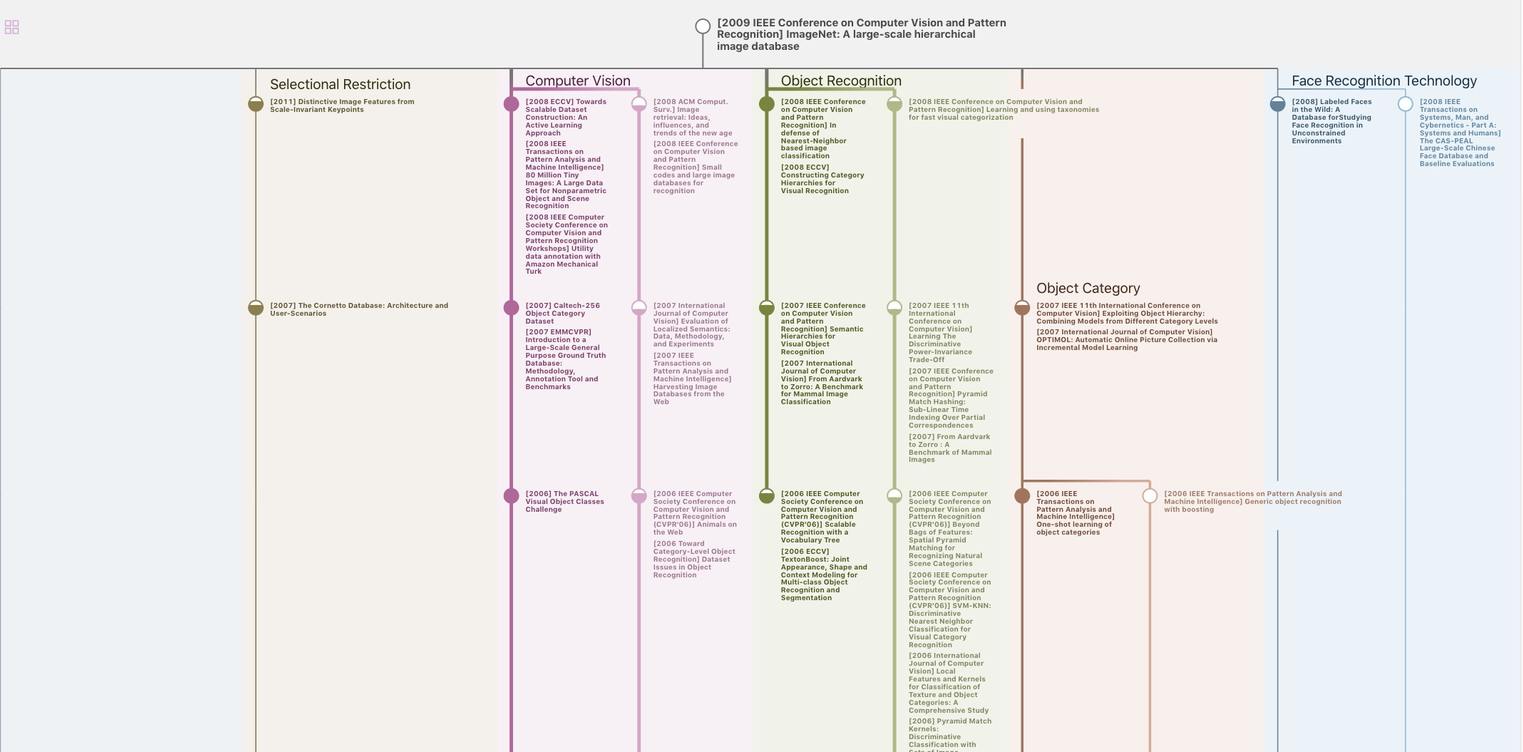
生成溯源树,研究论文发展脉络
Chat Paper
正在生成论文摘要