Augmentation of Various Speed Data by Controlling Frame Overlap for Acoustic Traffic Monitoring
2023 ASIA PACIFIC SIGNAL AND INFORMATION PROCESSING ASSOCIATION ANNUAL SUMMIT AND CONFERENCE, APSIPA ASC(2023)
摘要
In this study, we present a data augmentation method for machine-learning-based acoustic traffic monitoring that estimates traffic speed from the running-vehicle sound observed by microphones. Acoustic traffic monitoring is superior to existing traffic detectors using loop coils in terms of installation and maintenance costs, and machine-learning-based acoustic traffic monitoring has demonstrated high performance with sufficient training data. However, it is often difficult or burdensome to collect a large amount of running-vehicle sound with the corresponding labels of traffic speed over many days as training data. In addition, the tendency of the traffic speed changes significantly from day to day, depending highly on the traffic situation, such as congested or free flowing. This may result in biased traffic speed in the training data, particularly the absence of low-speed data. To overcome this problem, we propose a data augmentation method for artificially generating training data with a sufficient range of traffic speed from data with a limited range of traffic speed by controlling frame overlap in the time-frequency domain. In the experimental evaluation, a deep neural network was trained using only high-speed real data with and without the proposed data augmentation method, and the estimation performance characteristics for high-speed and low-speed test data were compared. The results showed that the estimation accuracy for low-speed data was greatly improved with the proposed data augmentation method, while that for high-speed data was kept almost the same, compared with the condition without data augmentation.
更多查看译文
AI 理解论文
溯源树
样例
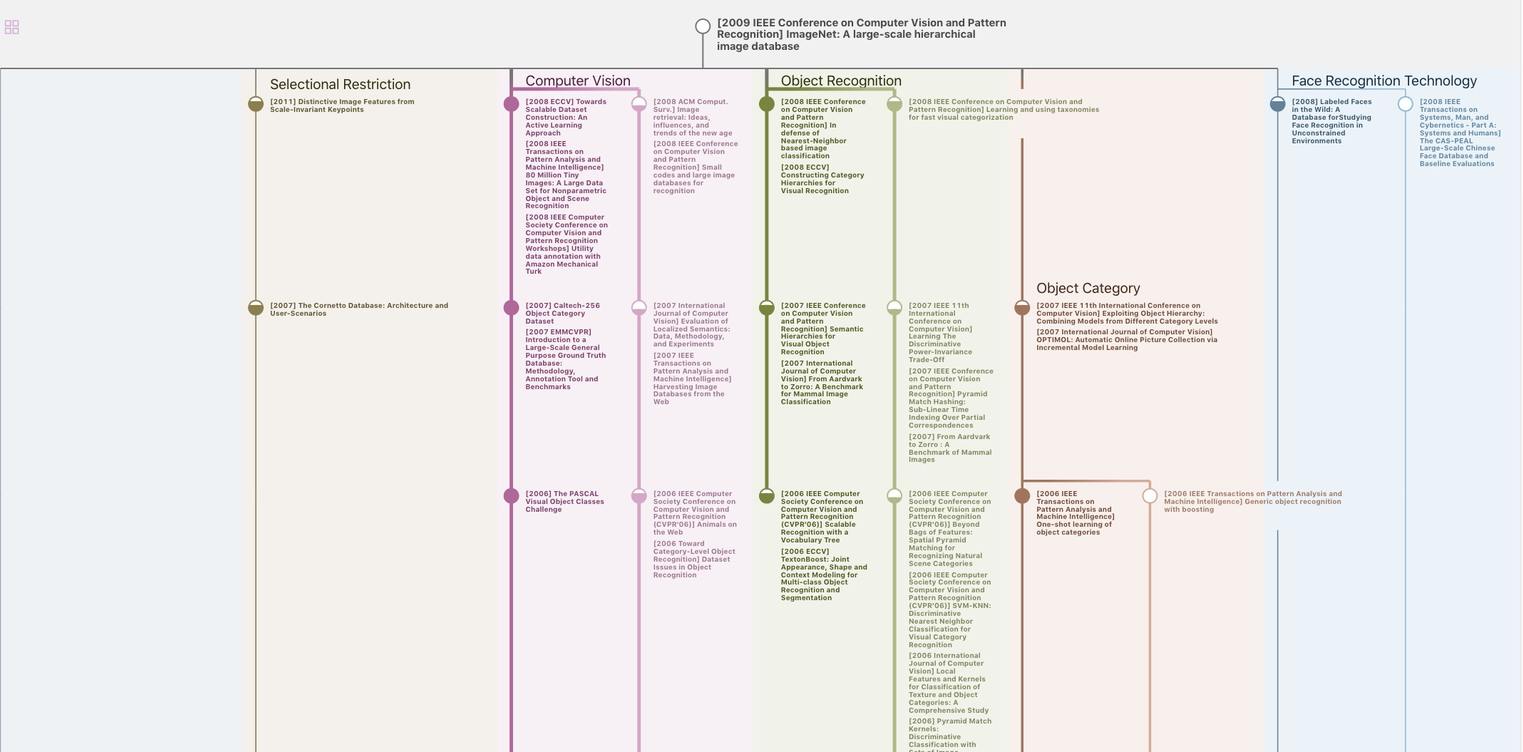
生成溯源树,研究论文发展脉络
Chat Paper
正在生成论文摘要