Vocal Tract Length Perturbation-based Pseudo-Speaker Augmentation for Speaker Embedding Learning
2023 ASIA PACIFIC SIGNAL AND INFORMATION PROCESSING ASSOCIATION ANNUAL SUMMIT AND CONFERENCE, APSIPA ASC(2023)
摘要
Data augmentation is essential for constructing reliable automatic speaker verification (ASV) systems. It is well known that data augmentation increases the number of utterances by adding noise and is effective in most methods. However, the number of speakers in the training data also plays an important role in enhancing ASV system performance. The robustness of speaker embedding networks, which are used in ASV systems, relies on the number of speakers present in the training data. To address this, we propose a method called pseudo-speaker augmentation, which utilizes a technique called vocal tract length (VTL) warping. By changing a parameter, the VTL warping technique alters speaker characteristics, allowing us to easily increase the number of speakers. Since the speaker embedding network aims to classify speakers, having a larger number of speakers enhances its robustness. In our experiments, the pseudo-speaker augmentation method improved the performance of the speaker embedding-based ASV system, achieving an equal error rate of 4.058% on the JTubeSpeech database.
更多查看译文
AI 理解论文
溯源树
样例
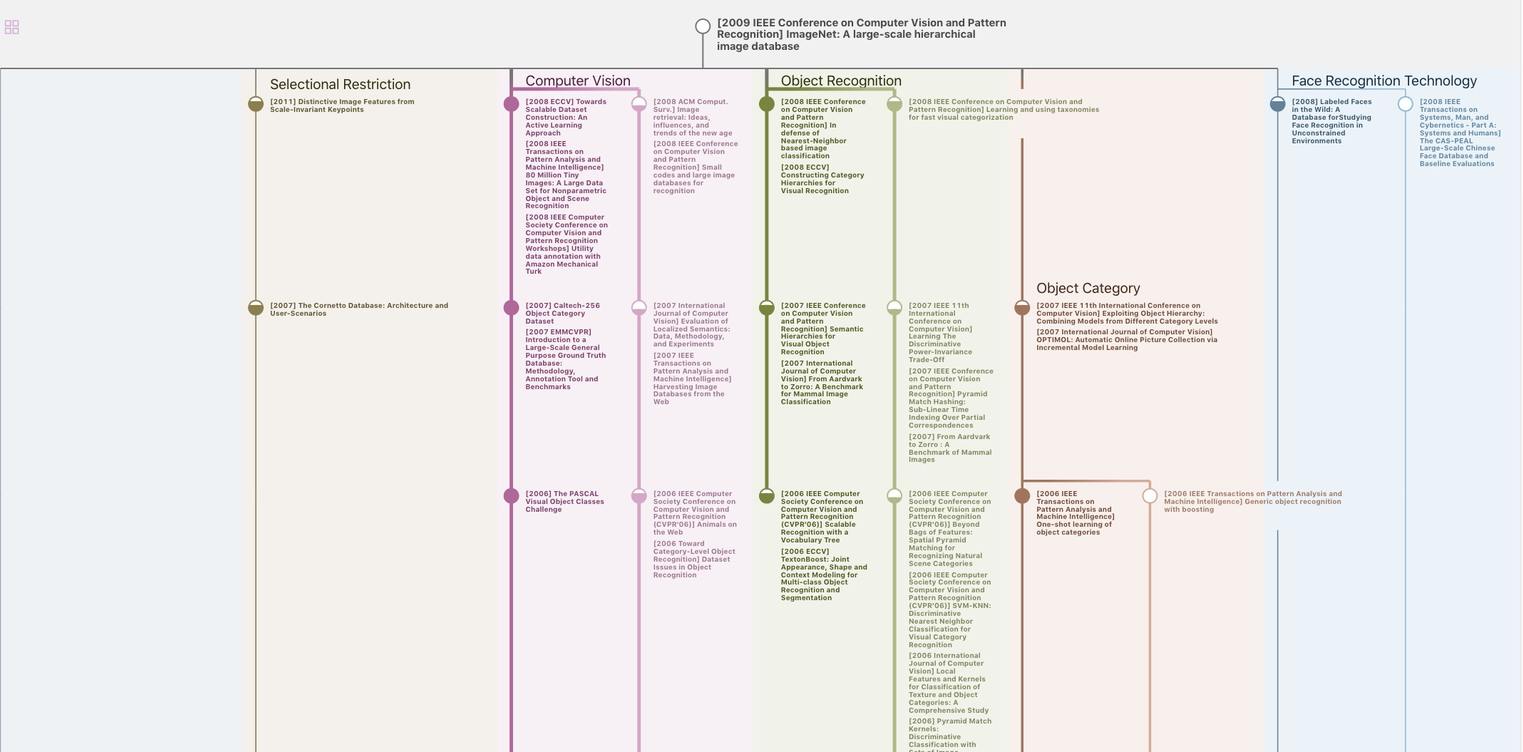
生成溯源树,研究论文发展脉络
Chat Paper
正在生成论文摘要