Audio-to-Score Singing Transcription Based on Joint Estimation of Pitches, Onsets, and Metrical Positions With Tatum-Level CTC Loss
2023 ASIA PACIFIC SIGNAL AND INFORMATION PROCESSING ASSOCIATION ANNUAL SUMMIT AND CONFERENCE, APSIPA ASC(2023)
摘要
This paper describes an end-to-end singing transcription method that directly estimates a musical score (a sequence of sung notes with metrical positions) from a music audio signal. The monotonicity of audio-to-score mapping naturally calls for the use of connectionist temporal classification (CTC). Inspired by the success of character-level automatic speech recognition, previous studies on CTC-based music transcription represent a musical score as a sequence of various kinds of symbols (e.g., note pitches and values and barlines) defined in some music notation. Such a naive notation-respecting representation, however, does not fit the non-overlapping monotonic audio-to-symbol alignment and the positions of barlines and the durations of beats tend to be incoherent in the estimated score. To solve this problem, we propose a tatum-level singing transcription method that jointly estimates the pitch (including rest), onset flag, and metrical position at each tatum. Our approach enables the tatums to be monotonically aligned with regularly-spanned intervals of the music signal and the estimated notes are located on the estimated metrical positions that are encouraged to be periodic. Experimental results clearly showed that our proposed model reached comparable accuracy on score-level singing transcription with only unaligned training data, and the proposed tatum-level representation significantly improved the stability of the metrical structures in the estimated scores.
更多查看译文
AI 理解论文
溯源树
样例
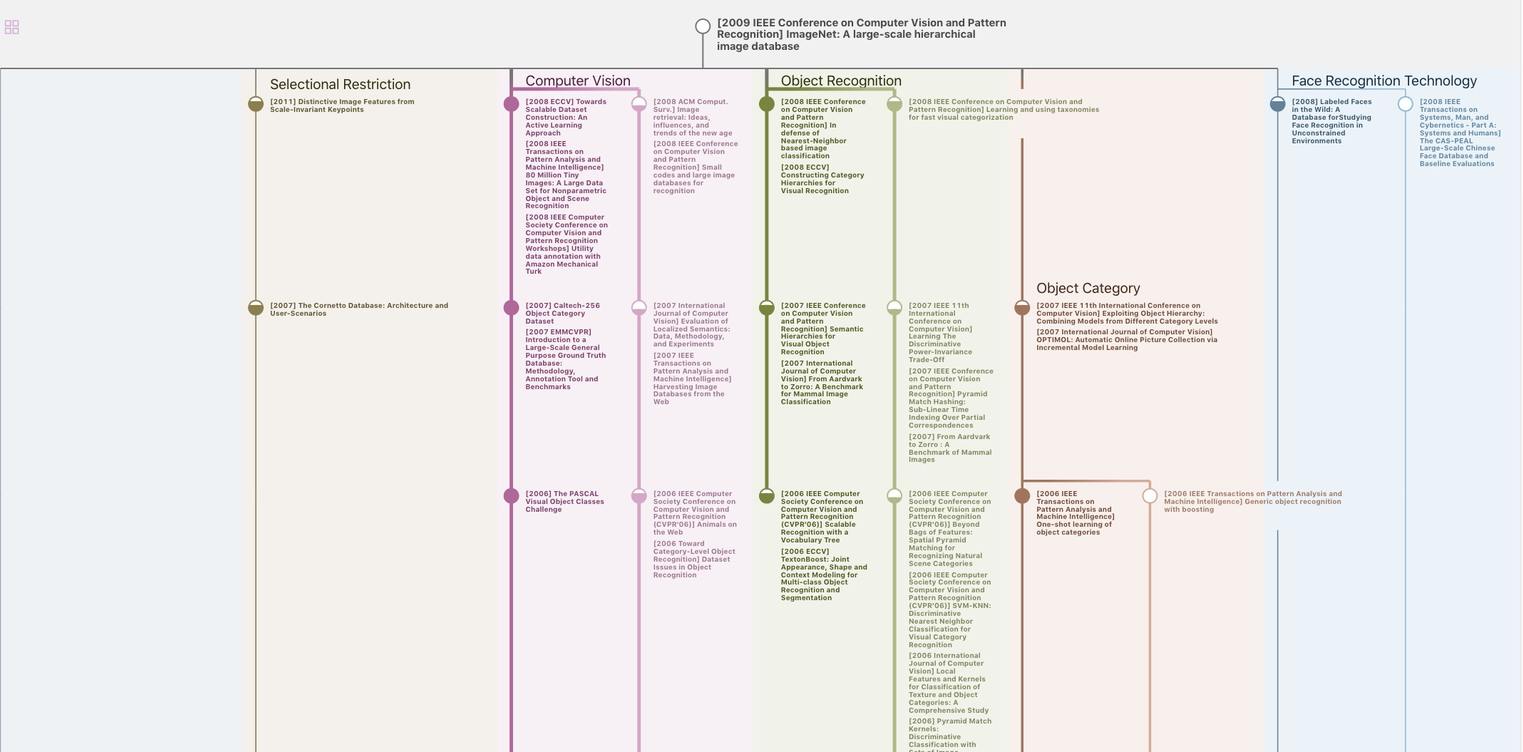
生成溯源树,研究论文发展脉络
Chat Paper
正在生成论文摘要