3D-GFE: a Three-Dimensional Geometric-Feature Extractor for Point Cloud Data
2021 Asia-Pacific Signal and Information Processing Association Annual Summit and Conference (APSIPA ASC)(2021)
摘要
In this paper, we propose a novel framework that extracts rotation-invariant features relative to the centroid and the reference point in a local point set. Furthermore, we search different scales of nearest neighbors simultaneously to acquire more rotation-invariant information around certain specific point cloud data. We evaluate our architecture with two point cloud tasks, object classification and part segmentation. Experiment results show that our method generates consistent results on randomly rotated data and achieves state-of-the-art performance without any rotational data augmentation. For classification, when training and testing with arbitrary rotations, our model is able to reach averagely 89.0% and 73.5% accuracy on the ModelNet40 and ScanObjectNN datasets, respectively. On the ShapeNet dataset, which is a part segmentation task, our model can achieve 77.7% mIOU.
更多查看译文
关键词
Point Cloud,Classification,Segmentation,Rotation-Invariant,Geometric-Feature Descriptor
AI 理解论文
溯源树
样例
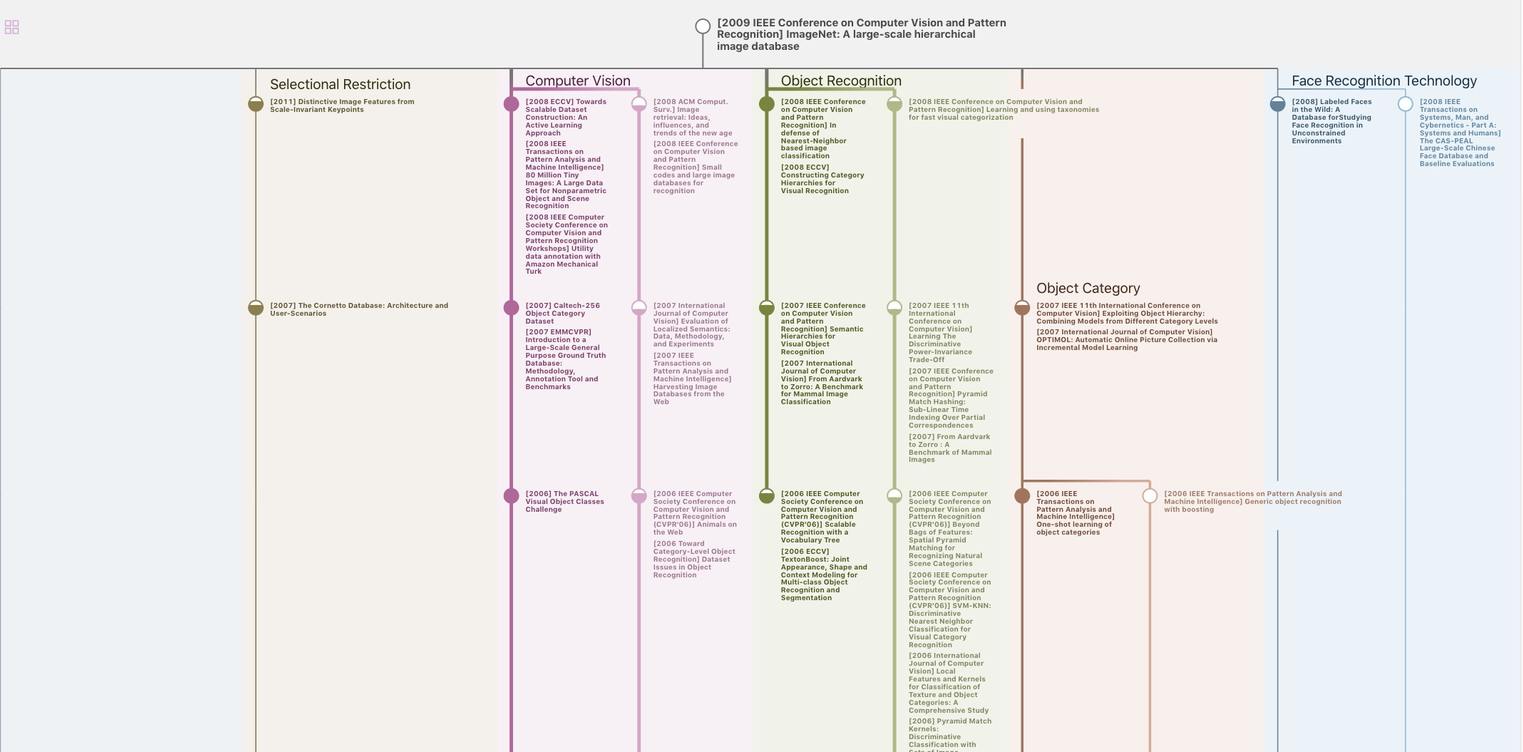
生成溯源树,研究论文发展脉络
Chat Paper
正在生成论文摘要