A Multiple Branch Fusion Network for Feature Learning and Hyperspectral Image Classification
2023 14th International Conference on Computing Communication and Networking Technologies (ICCCNT)(2023)
Abstract
Hyperspectral image (HSI) classification has made extensive use of deep learning approaches, specifically Convolutional Neural Network (CNN). CNN's ability to fully capture local contextual aspects of HSI makes it an excellent HSI classifier. Although, a significant factor in the poor classification performance is the scarcity of training data. Conventional CNN-based methods don't use the integrate-band relationships of HSI as much as they could because they typically employ 2D-CNNs to extract features. On the other hand, 3D-CNNs use a similar procedure to get both spectral and spatial data. This gets around the problem with 2D-CNNs, but it could mean that not enough features are extracted. As a solution, we offer a multi-scale 3D-CNN with multi branch feature fusion, which we call multi branch fusion CNN (MBF-CNN), for HSI classification. We begin by applying 3D-CNN on multiple scales to extract HSI features. After that, the multiple distinct characteristics are streamlined and combined together. The fused features then go through several fully connected layers to get the classification outcomes. The experiments are executed on both the new data sets and the publicly accessible benchmark data sets to expose the efficiency and durability of the proposed model. Empirically, it has been demonstrated that the presented model succeeds over the remaining state-of-the-art approaches in terms of classification accuracy.
MoreTranslated text
Key words
Hyperspectral image,Deep learning,Convolutional Neural Networks,Classification,Feature Fusion
AI Read Science
Must-Reading Tree
Example
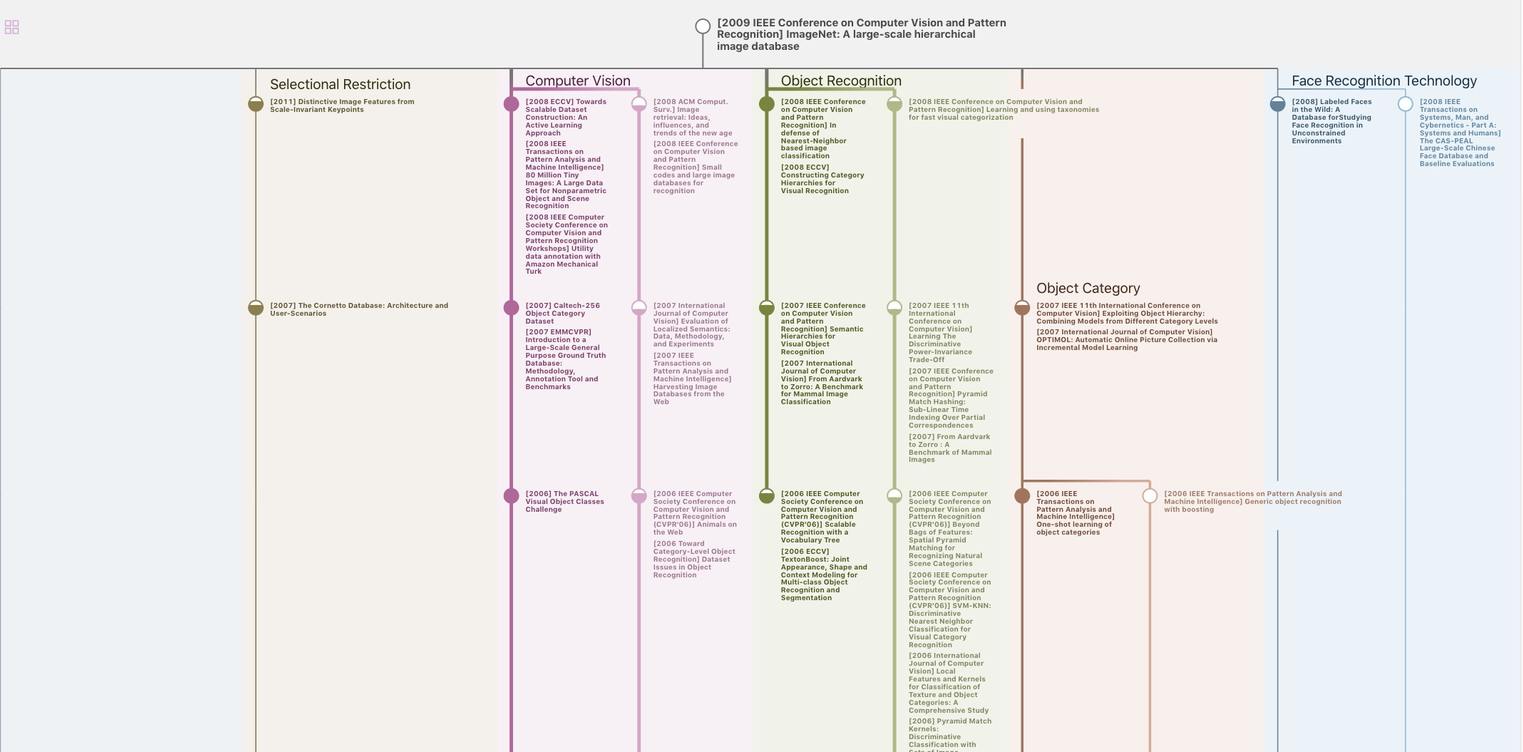
Generate MRT to find the research sequence of this paper
Chat Paper
Summary is being generated by the instructions you defined