Prediction of Post-COVID-19 Pandemic Learning Mode Preferences Using Machine Learning
2023 14th International Conference on Computing Communication and Networking Technologies (ICCCNT)(2023)
Abstract
The COVID-19 epidemic has had a huge impact on the educational landscape, prompting the adoption of online and remote learning as viable alternatives to conventional in-person instruction. In order to create effective educational methods as institutions prepare for the post-pandemic period, it is essential to understand students' preferences for various learning modes. With the aid of machine learning techniques, this study seeks to forecast preferences for learning styles in post-pandemic higher education. The study made use of a dataset compiled from a wide range of college students, which included information on the institution type (public or private), program type (bachelor's or diploma), academic discipline (science or humanities), gender, year of study, internet use, communication costs, comorbidity status, vaccination history, gadget and laptop ownership, and preferred learning mode (online, in-person, or both). For the aim of prediction, a Support Vector Machine (SVM) was used. Its kernel functions included linear, polynomial, radial basis function (RBF), and sigmoid. Performance criteria like accuracy, precision, recall, and F1 score were used to assess the models after the dataset was randomly partitioned into training and testing sets. The findings showed that for identifying the preferred mode of learning, SVM with the RBF kernel had the highest prediction accuracy (91.67%). Further proving the RBF kernel's efficacy, the confusion matrix analysis showed that high values in the diagonal represented accurate predictions for each of the three classes (both online and onsite). The results imply that machine learning approaches, in particular SVM with the RBF kernel, may accurately forecast students' preferences for learning modes in the post-pandemic situation. In a post-pandemic society, the findings provide insightful information that may be used to build adaptable and inclusive learning models and put them into practice.
MoreTranslated text
Key words
post-pandemic,learning preferences,higher education,mode of learning,predictive modeling
AI Read Science
Must-Reading Tree
Example
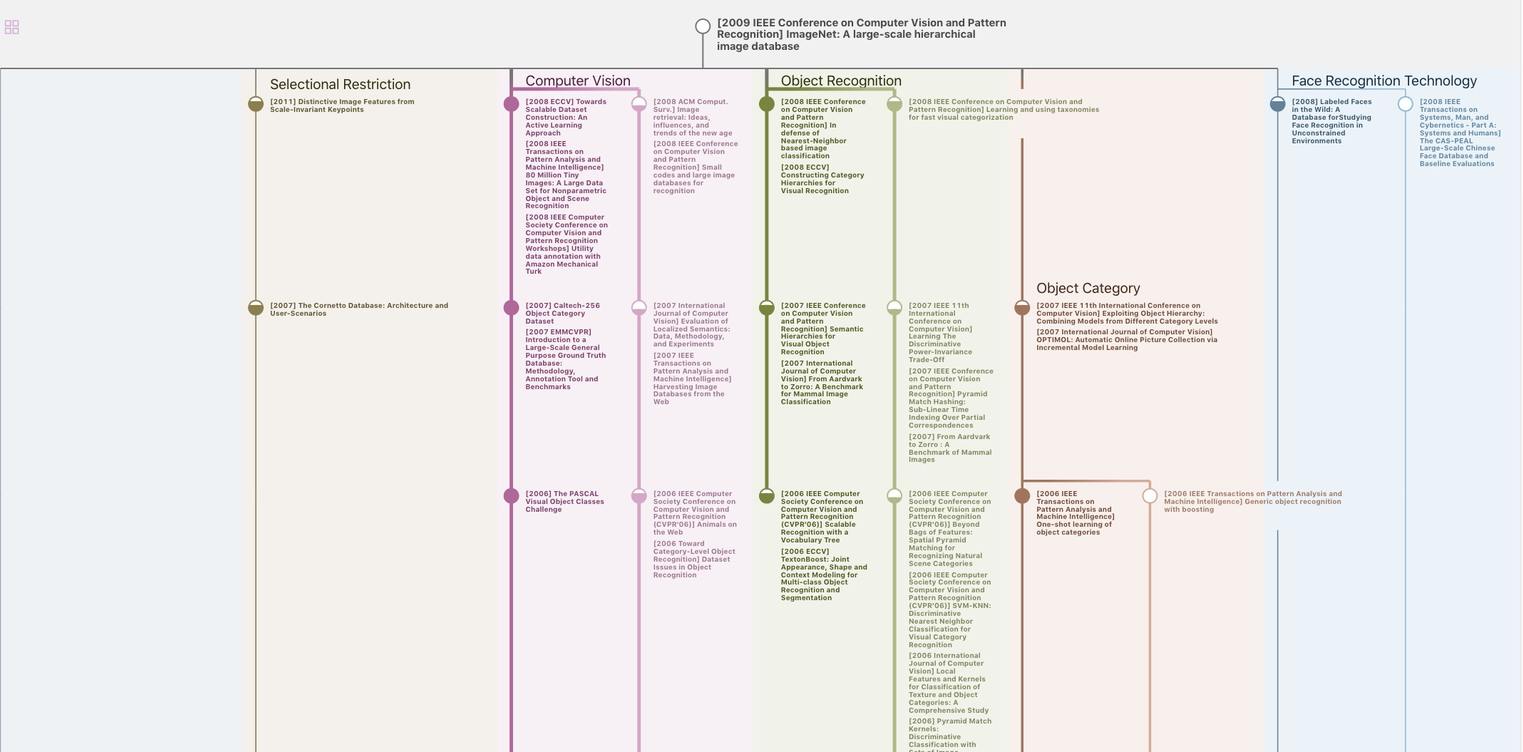
Generate MRT to find the research sequence of this paper
Chat Paper
Summary is being generated by the instructions you defined