Data Augmentation with Automatically Generated Images for Character Classifier Model Training
2023 IEEE 12th Global Conference on Consumer Electronics (GCCE)(2023)
摘要
This paper presents a novel data augmentation technique crucial for training AI-OCR systems for handwritten character classification. Using a Y-autoencoder (Y-AE) enhanced with Adaptive Instance Normalization, diverse handwriting styles are generated to improve the breadth of handwriting representations. A filtering mechanism is introduced to include only valid character images for training. The method was tested on a subset of the ETL Character Database, featuring 92 unique Japanese Hiragana and Katakana characters. The baseline classifier achieved an accuracy of 0.9061. However, when using the augmented dataset, which included Y-AE model-generated and filtered images, the accuracy improved to a maximum 0.9555 with data augmentation technique. These results showcase the potential of this data augmentation technique in consumer electronics, particularly in AI-OCR software. Despite needing some noise removal, the approach significantly boosts classifier accuracy, suggesting an efficient way forward for document processing in various sectors.
更多查看译文
关键词
Data augmentation,Handwritten character recognition,Image generation,Y-autoencoder
AI 理解论文
溯源树
样例
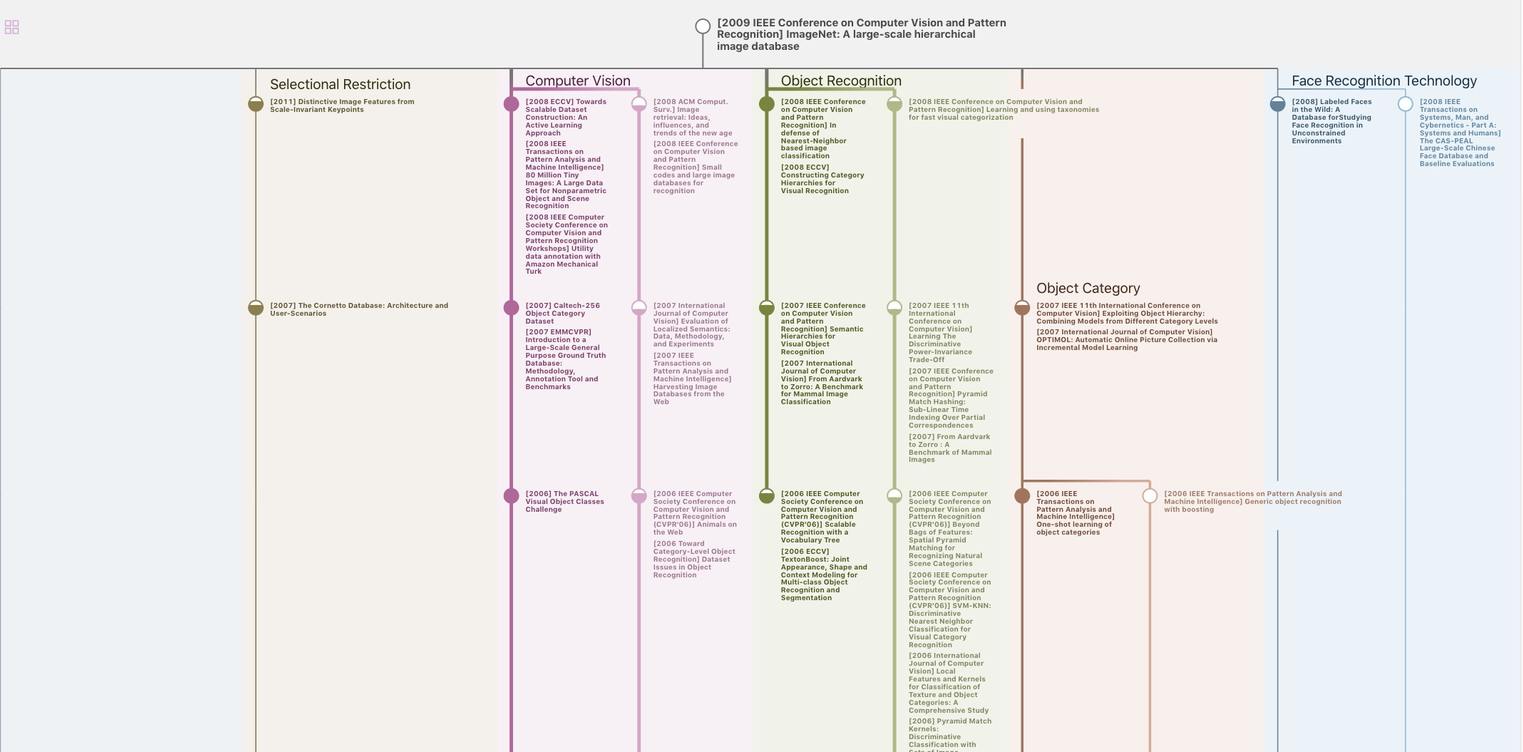
生成溯源树,研究论文发展脉络
Chat Paper
正在生成论文摘要