Individual-Driven Spiking-Mixer Deep Learning Model for IRS-Assisted mmWave Systems Beam Training
2023 IEEE 12th Global Conference on Consumer Electronics (GCCE)(2023)
摘要
The impacts of noise and channel variation are critical challenges for beam training in millimeter wave (mmWave) intelligent reflecting surface (IRS) systems. This paper proposes a novel hybrid deep learning (DL) scheme containing individual spiking encoders and a feature mixer network named the individual-driven spiking-mixer (IDSM) DL model. The proposed spiking encoder converts channel intensities into binary sequences by the leaky integrate-and-fire (LIF) mechanism for a robust channel representation. Moreover, the feature mixer network obtains the optimal beam through two views of firing tokens quantified from binary sequences. Experimental results show that our proposal can achieve reliable beam prediction and a high channel capacity with low complexity.
更多查看译文
关键词
mmWave,intelligent reflecting surface,hybrid deep learning,spiking neural network
AI 理解论文
溯源树
样例
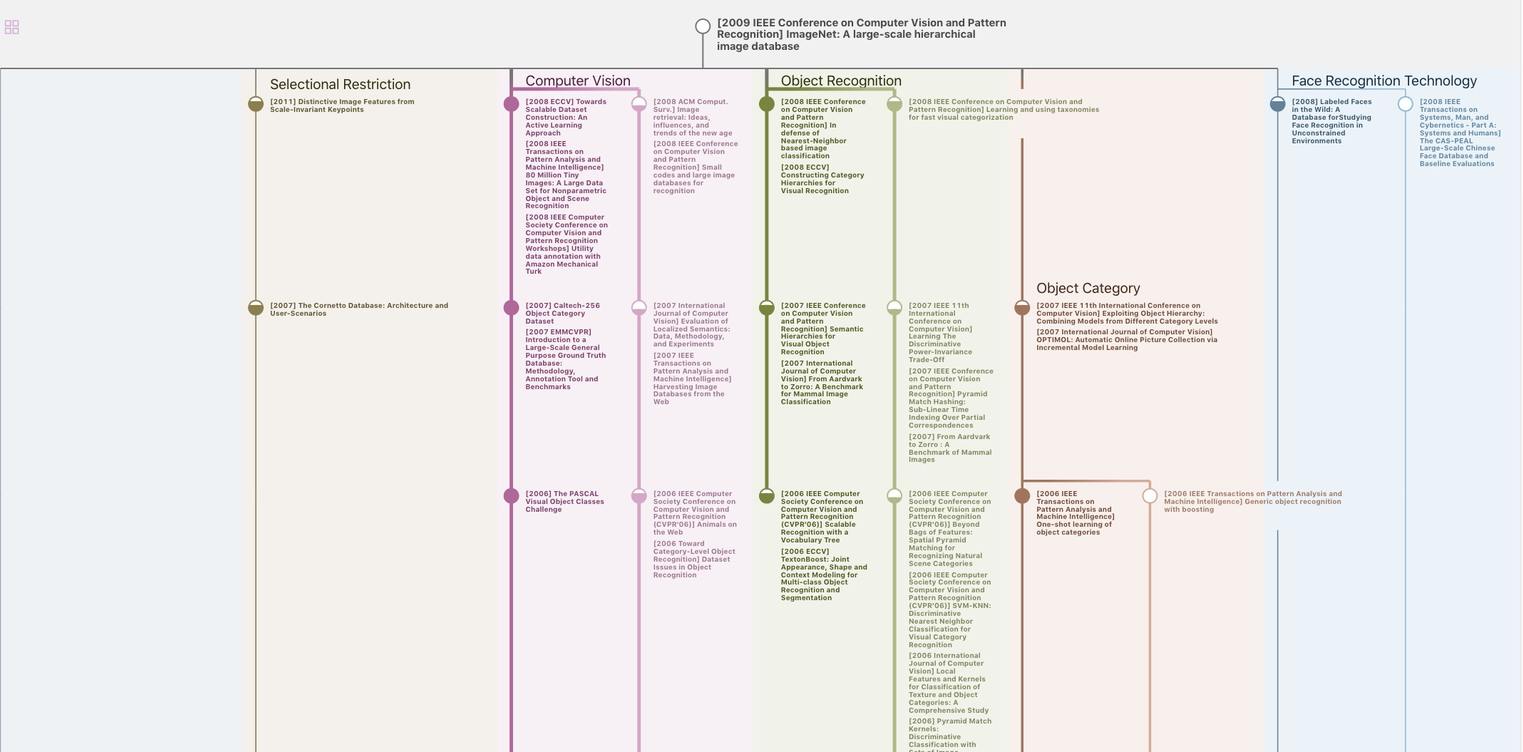
生成溯源树,研究论文发展脉络
Chat Paper
正在生成论文摘要