Probabilistic Forecasting of Electric Vehicle Charging Load using Composite Quantile Regression LSTM
2023 IEEE/IAS INDUSTRIAL AND COMMERCIAL POWER SYSTEM ASIA, I&CPS ASIA(2023)
Abstract
With the explosive development of electric vehicles (EVs), the impact on the operation and planning of low voltage distribution network has become more and more significant. Therefore, ordered charging control strategies of EVs are proposed, which require accurate EV charging load forecasting. Under this background, this paper carries out a probabilistic forecasting model combining composite quantile regression and LSTM neural network for EV charging load forecasting. Firstly, a composite long-short term memory (LSTM) neural network is built to obtain synchronously quantile forecasting. Secondly, kernel density estimation method is used to estimate probability density function. Finally, the performance of the proposed model is verified on real residential EV charging load data, with 97.92% predicting interval coverage probability (PICP) at 90% confidence level, better than the comparison model.
MoreTranslated text
Key words
Electric Vehicle,Charging Load Forecasting,Probabilistic Forecasting,Composite Quantile Regression,LSTM
AI Read Science
Must-Reading Tree
Example
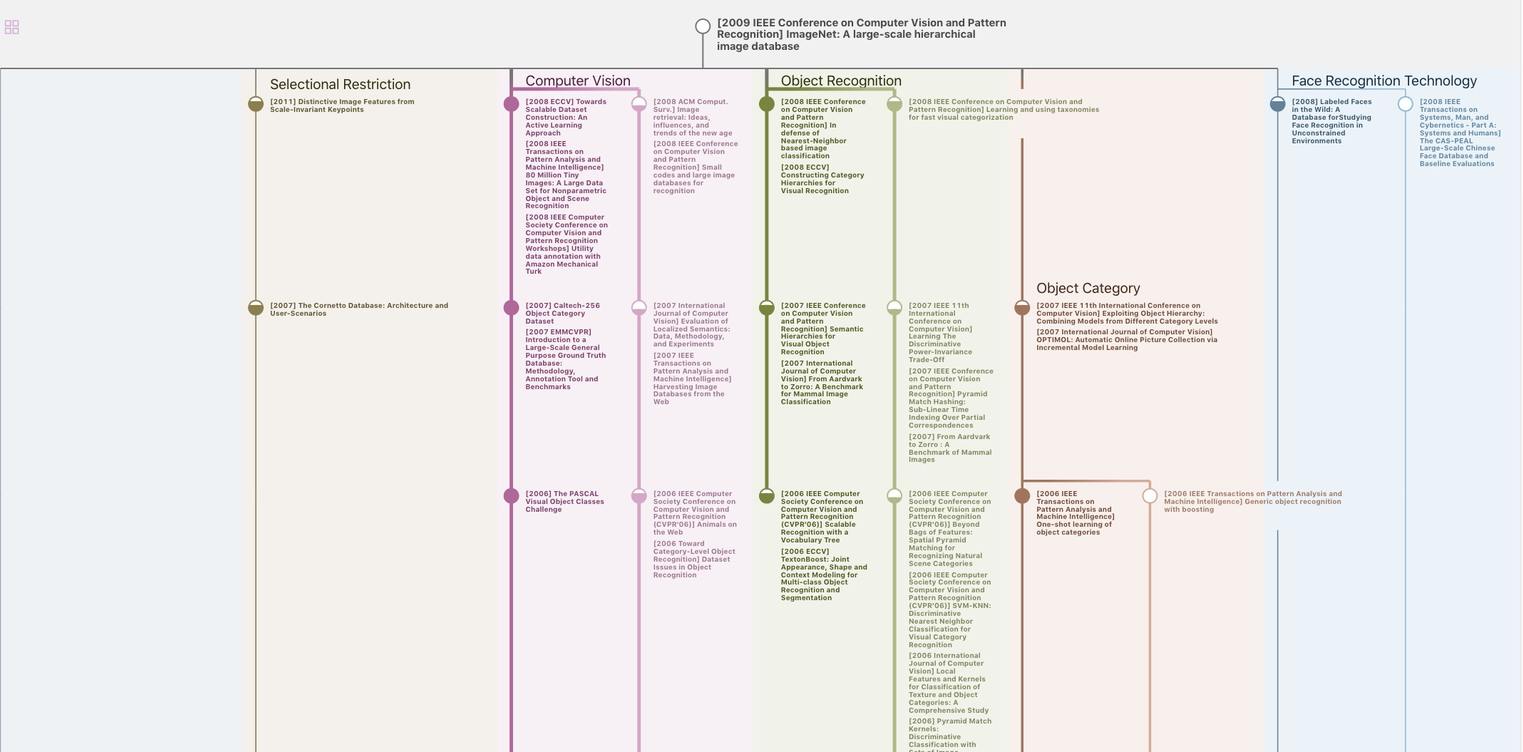
Generate MRT to find the research sequence of this paper
Chat Paper
Summary is being generated by the instructions you defined