A Knowledge-driven Cross-modal Memory Network for Radiology Report Generation
2023 CAA Symposium on Fault Detection, Supervision and Safety for Technical Processes (SAFEPROCESS)(2023)
摘要
In previous studies, most work focused on generating high-quality reports by smoothing the alignment between cross-modal mappings. However, a common problem with current radiology report generation methods is that they ignore the interactions and connections between various medical findings that are critical to medical discovery. To overcome this problem, we propose a knowledge-driven cross-modal memory network. The network is built by data mining clinical reports and constructing knowledge graphs and their corresponding adjacency matrices based on the mined a prior knowledge. Combining information after graphical interaction through a cross-modal memory network with a knowledge graph adjacency matrix. Finally the Transformer generates a full report. Experiments have demonstrated that reports generated from cross-modal memory network models that incorporate prior knowledge are more clinically informative.
更多查看译文
关键词
radiology report,knowledge graph,cross-modal,deep learning,prior knowledge
AI 理解论文
溯源树
样例
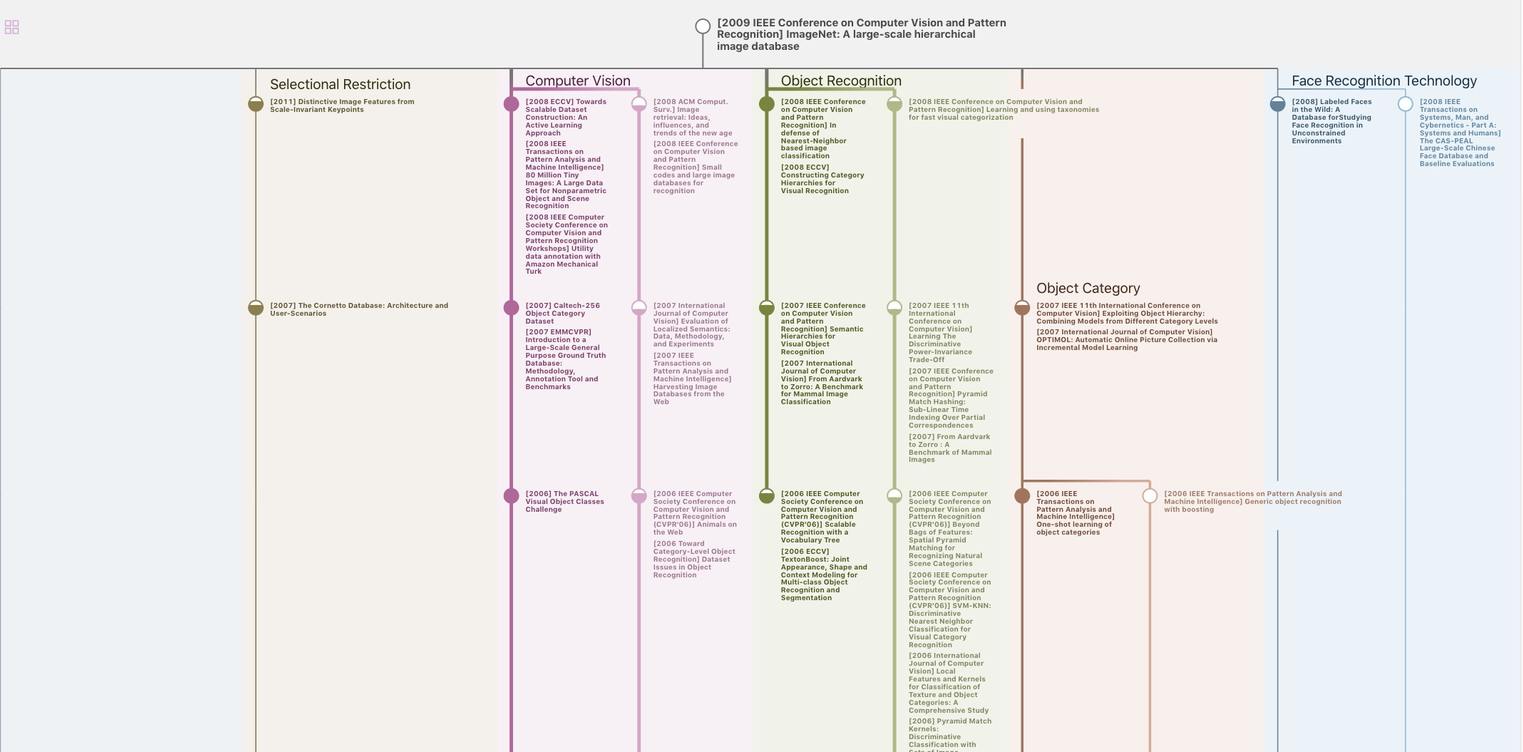
生成溯源树,研究论文发展脉络
Chat Paper
正在生成论文摘要