Transformer-based image restoration with divergent self-attentive mechanism
2023 CAA Symposium on Fault Detection, Supervision and Safety for Technical Processes (SAFEPROCESS)(2023)
摘要
When convolution is used to process corrupted inputs, each filter of the convolution shares convolution kernel parameters spatially. For a single image containing both normal and abnormal regions, assigning the same kernel to operations on features that are valid, invalid, or a mix of both can easily lead to structural distortion, texture blurring and artifacts. This is especially true when the pattern is complex or the corrupted region is too large. Secondly, convolution is inefficient at modeling long-range image dependencies in CNNs with only local receptive fields. Accordingly, this paper proposes a novel Transformer-based split-flow multi-headed self-attentive mechanism image restoration method. The main components of the restoration network include a generator and a discriminator, alongside a divergent self-attentive mechanism and a detailed feedforward network that aim to extract contextual information in hierarchical features to generate appropriate feature maps for reconstructing images. The method presented in this paper effectively utilizes hierarchical features to extract relevant information from corrupted images. Experiments on the CelebA and Places datasets show that our proposed method have excellent performance.
更多查看译文
关键词
multi-headself-attention mechanism,Transformer,Convolutional neural network,detail feedforward network
AI 理解论文
溯源树
样例
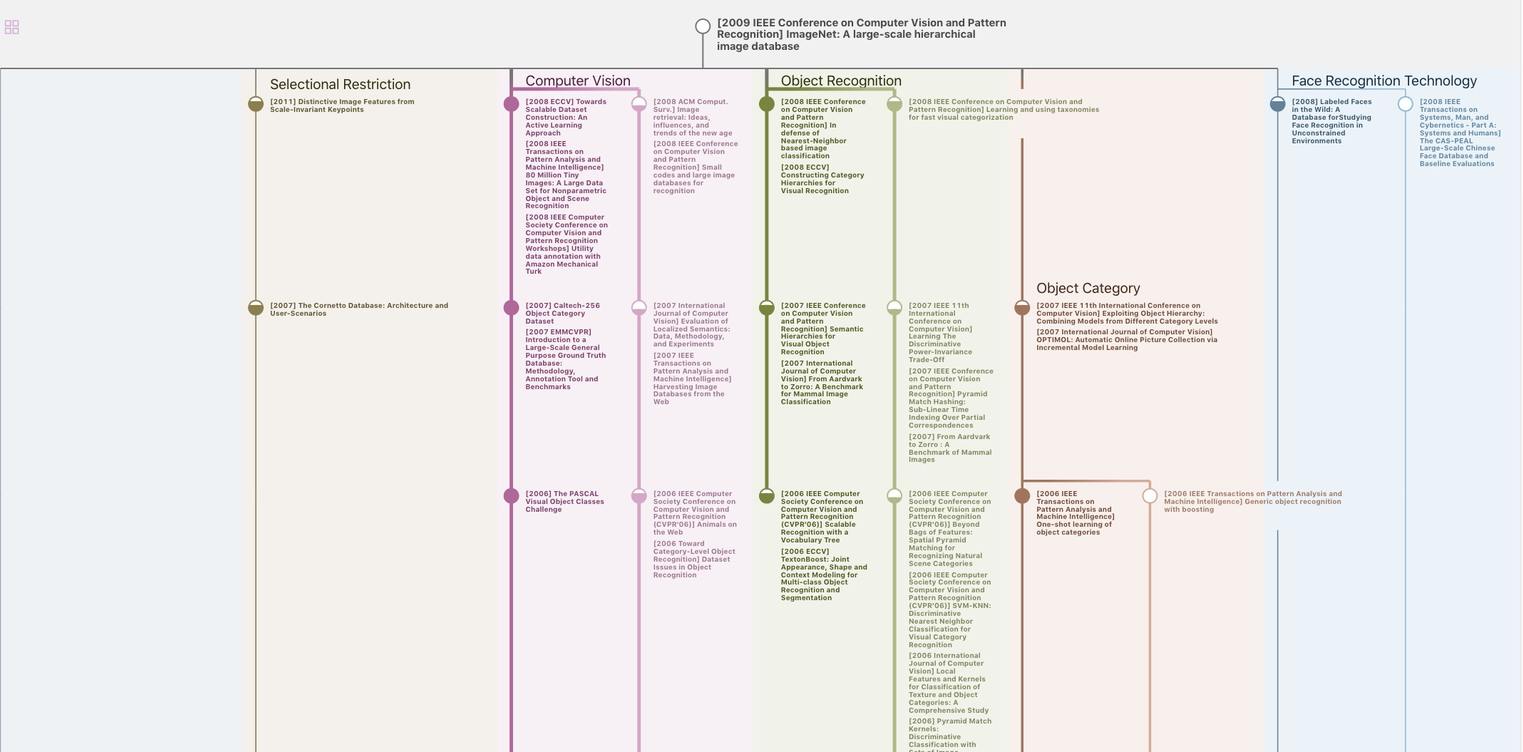
生成溯源树,研究论文发展脉络
Chat Paper
正在生成论文摘要