Fault detection based on multifractal analysis and variational autoencoder for complex industrial process
2023 CAA Symposium on Fault Detection, Supervision and Safety for Technical Processes (SAFEPROCESS)(2023)
摘要
Deep learning is an effective tool to learn high level feature for process monitoring in complicated industry production. However, most traditional deep learning networks do not take the geometric characters and singularities of the original data into consideration. Meanwhile, owing to the learned deep features usually can not obey the normal distribution, this may lead to the inaccurate modeling and it is not beneficial for monitoring statistics establishing. Thus, in this paper, we proposes a deep feature learning method integrating multifractal analysis with variational autoencoder (MF-VAE) for process monitoring in complicated chemical processes. Through the unsupervised training, MF-VAE can extract more informative multifractal representation from the original data. First, the multi-scale self-similar features of process data are extracted by the multifractal analysis. Considering inherent data distribution of generated feature from deep learning network, VAE is then utilized to learn key feature from the obtained multifractal feature. The learned encoder feature is provided to construct the monitoring statistic. Finally, the Tennessee Eastman (TE) process is used to evaluate the proposed method.
更多查看译文
关键词
deep learning,fault detection,multifractal analysis,variational autoencoder,TE process
AI 理解论文
溯源树
样例
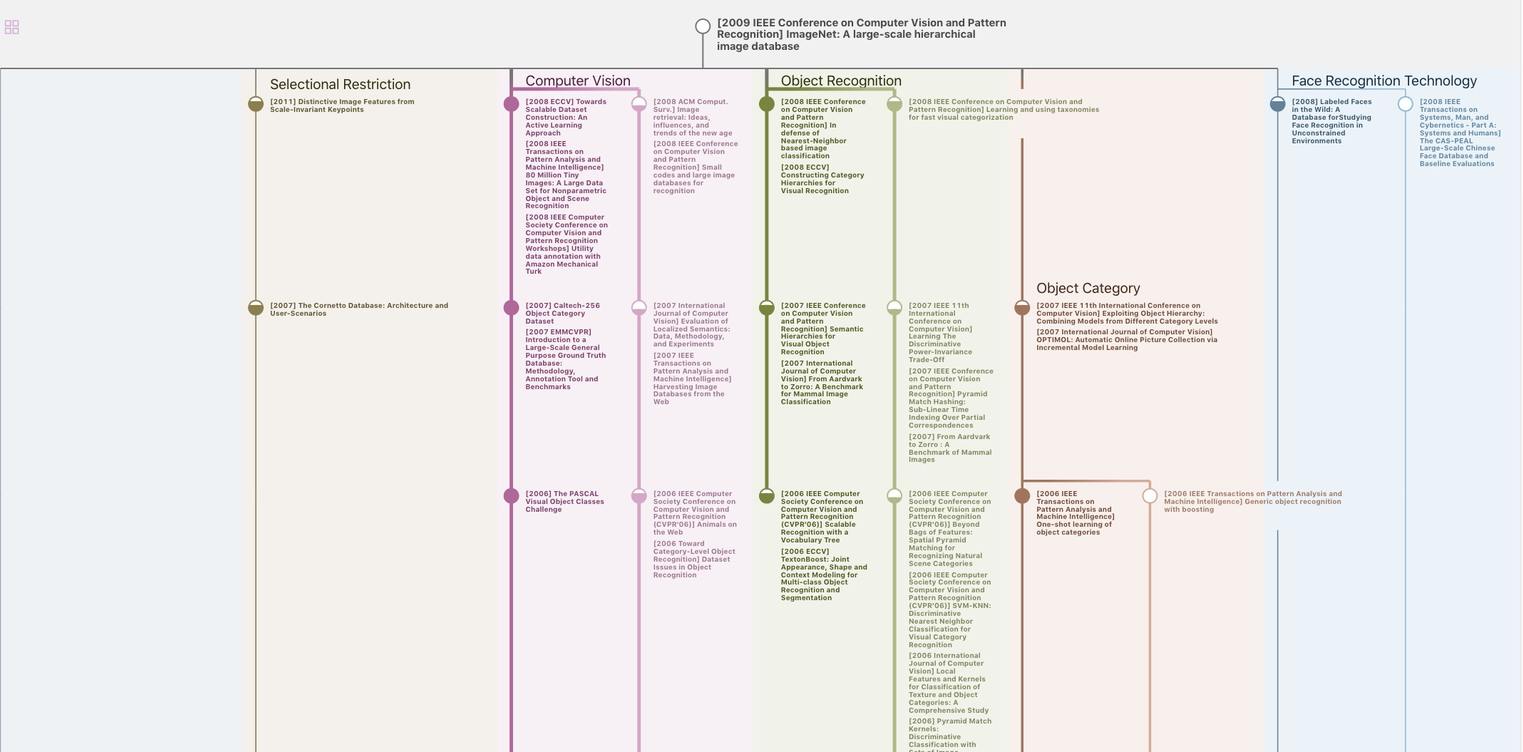
生成溯源树,研究论文发展脉络
Chat Paper
正在生成论文摘要