ProPML: Probability Partial Multi-label Learning
2023 IEEE 10th International Conference on Data Science and Advanced Analytics (DSAA)(2024)
摘要
Partial Multi-label Learning (PML) is a type of weakly supervised learning
where each training instance corresponds to a set of candidate labels, among
which only some are true. In this paper, we introduce , a novel
probabilistic approach to this problem that extends the binary cross entropy to
the PML setup. In contrast to existing methods, it does not require suboptimal
disambiguation and, as such, can be applied to any deep architecture.
Furthermore, experiments conducted on artificial and real-world datasets
indicate that outperforms existing approaches, especially for high noise
in a candidate set.
更多查看译文
AI 理解论文
溯源树
样例
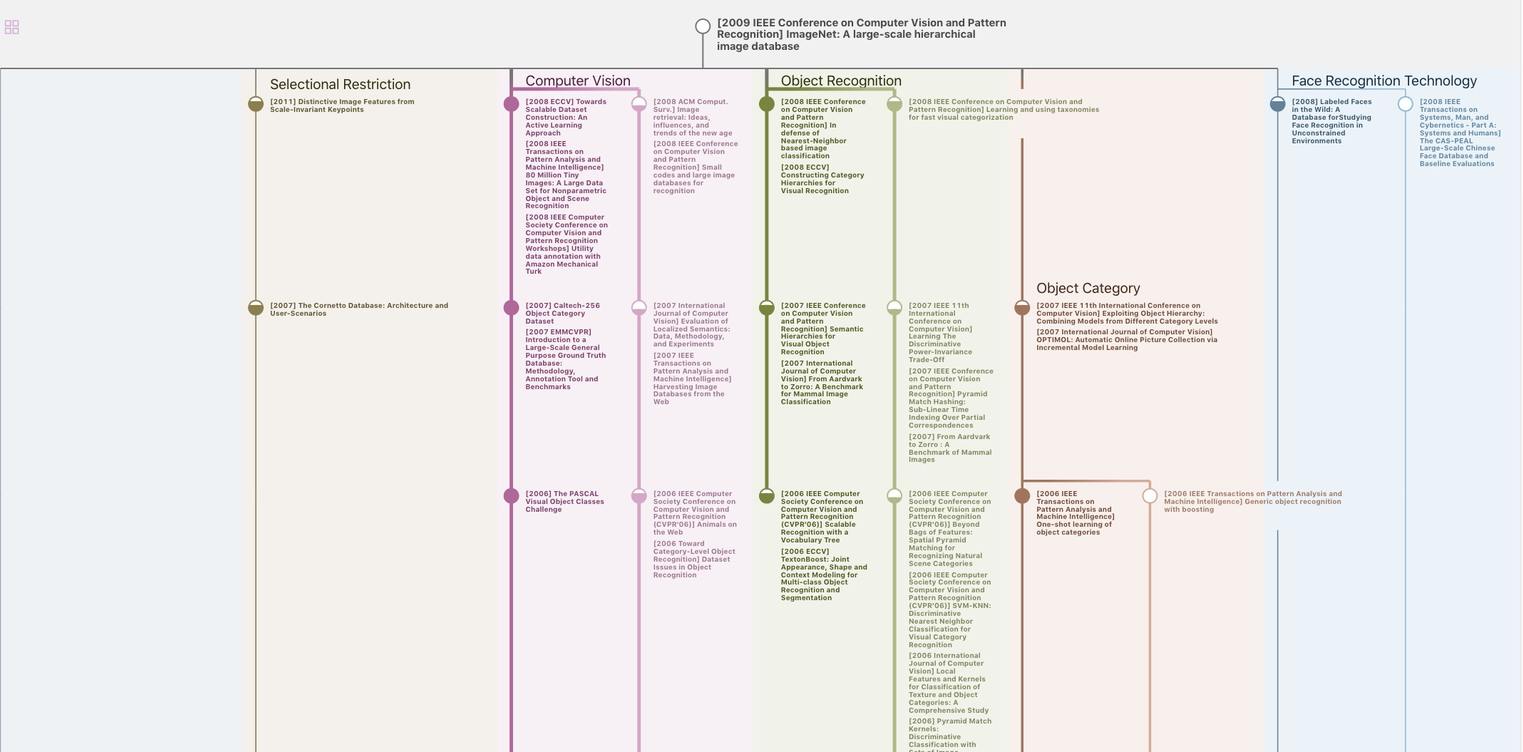
生成溯源树,研究论文发展脉络
Chat Paper
正在生成论文摘要