A Residual Attention-based EfficientNet Homography Estimation Model for Sports Field Registration
IECON 2023- 49th Annual Conference of the IEEE Industrial Electronics Society(2023)
摘要
Accurate sports field registration plays a crucial role in computer vision for effective team sports analysis, as it provides the ability to track and recognize the positions, movements, and interactions of players from image space to real-world field space. In this paper, we propose a two-stage deep learning framework for sports field registration. In the first-stage network, we utilize a Residual EfficientNet-Attention U-Net architecture to estimate the initial homography matrix using predefined keypoints to register sports fields. Subsequently, our second-stage network refines the initial homography for improved accuracy. We evaluate our proposed method on the public sports datasets to show its outperform results against the state-of-the-art methods in some evaluation metrics.
更多查看译文
关键词
sports field registration,homography estimation,keypoints detection
AI 理解论文
溯源树
样例
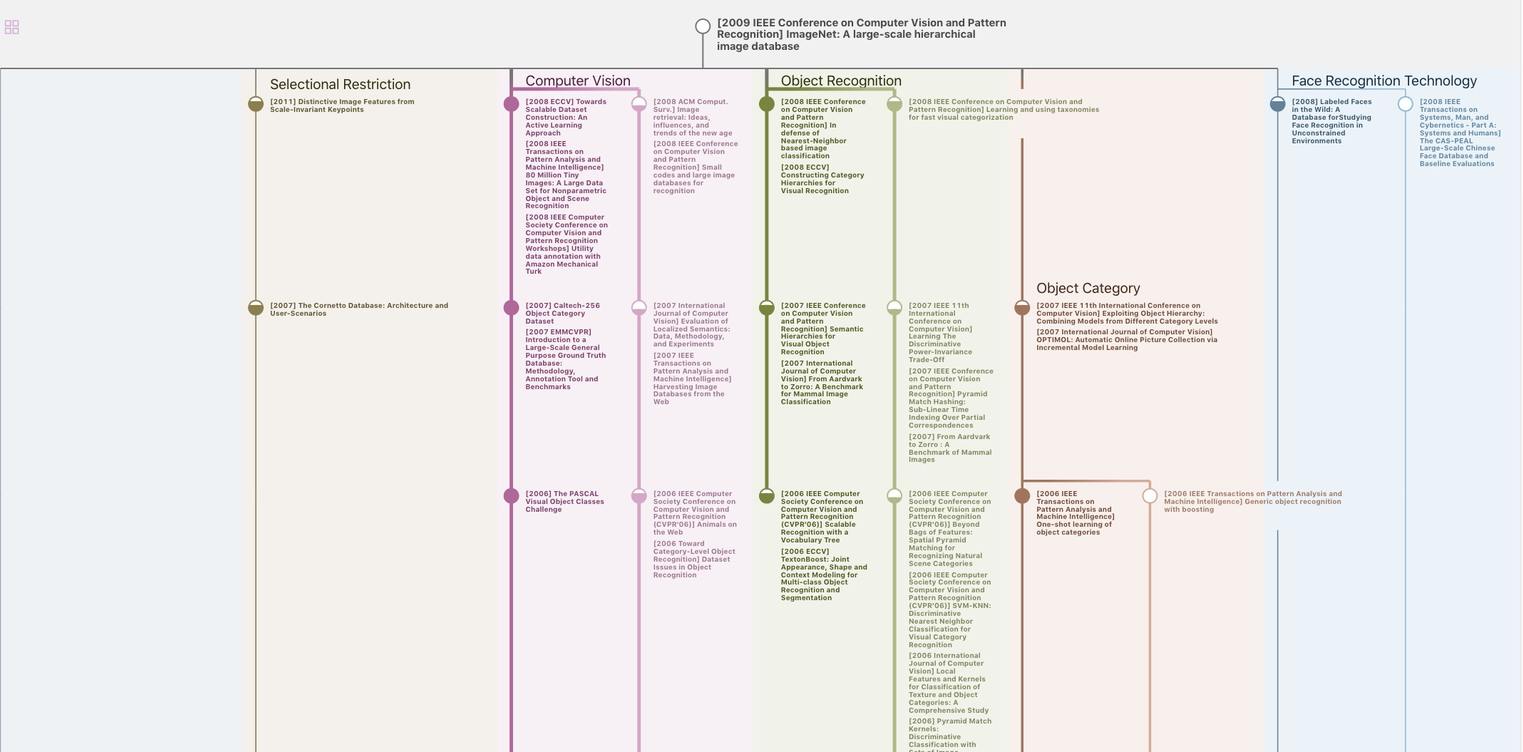
生成溯源树,研究论文发展脉络
Chat Paper
正在生成论文摘要