Digital Twin-Enabled Decision-Making Framework for Multi-UAV Mission Planning: A Multiagent Deep Reinforcement Learning Perspective
IECON 2023- 49th Annual Conference of the IEEE Industrial Electronics Society(2023)
Abstract
The utilization of multiple unmanned aerial vehicles (multi-UAVs) greatly enhances the quality of public services, whereas presents challenges to effective mission planning. Existing research predominantly concentrates on task allocation and path planning, with limited emphasis on integrating UAV deployment and task allocation. Despite the promise of learning-based approaches for joint optimization, their applicability to sim-to-real problems remains restricted. In this paper, we propose a hierarchical decision-making framework empowered by digital twin (DT) technology to enhance resource-efficient utilization and facilitate realtime mission planning. In the upper layer, a swarm of UAVs is divided into subgroups using a K-means method and strategically deployed to corresponding task regions. In the lower layer, a DT-enabled reinforcement learning approach is adopted to leverage real-world experiences. Specifically, we employ a multiagent deep Q learning algorithm to optimize the training process, where each agent interacts with the DT environment through original and target networks to acquire an optimal strategy. The proposed approach is evaluated using simulations that demonstrate its effectiveness in achieving high-quality plans.
MoreTranslated text
Key words
Multi-UAV,decision-making,mission planning,multiagent deep Q learning,digital twin
AI Read Science
Must-Reading Tree
Example
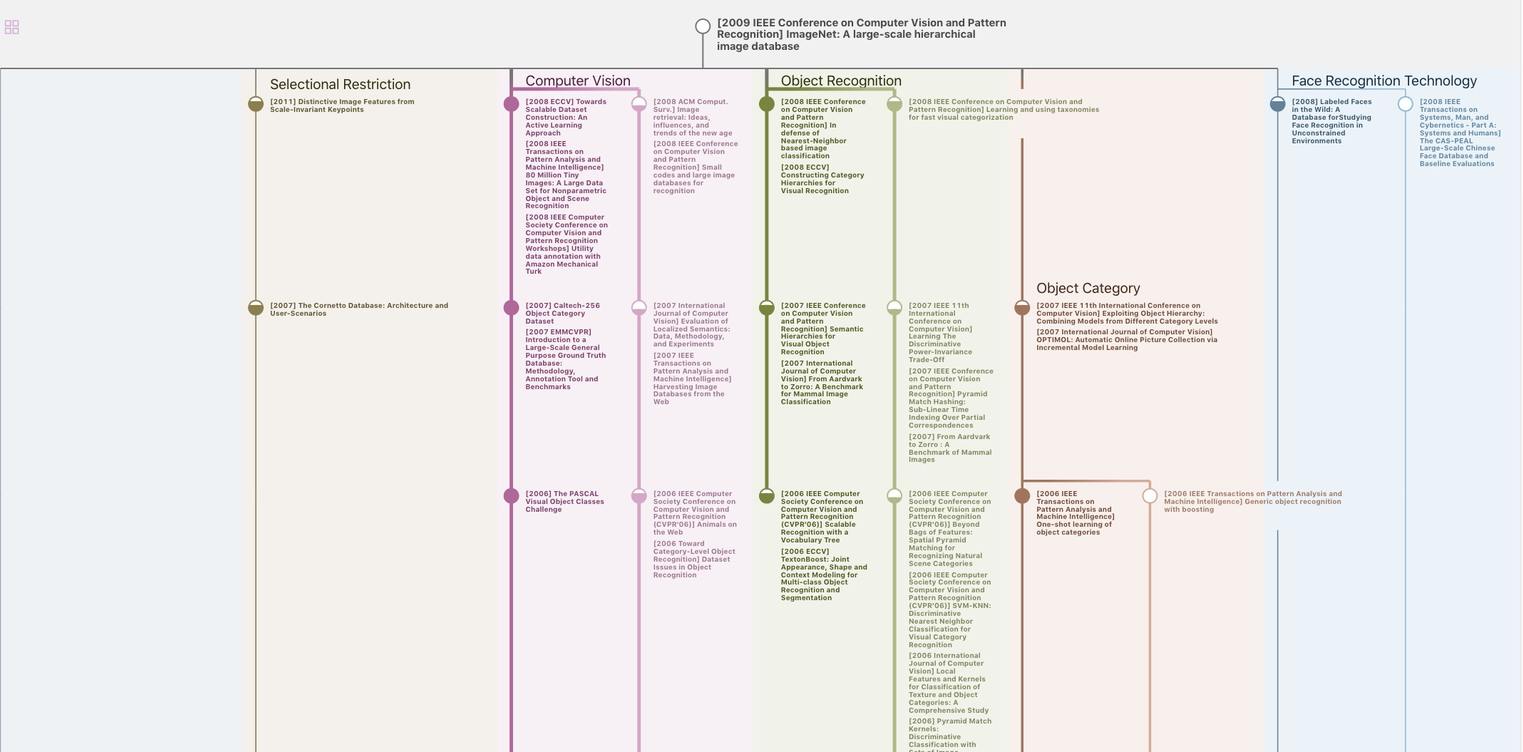
Generate MRT to find the research sequence of this paper
Chat Paper
Summary is being generated by the instructions you defined