BAS$^{4}$ Net: Boundary-Aware Semi-Supervised Semantic Segmentation Network for Very High Resolution Remote Sensing Images
IEEE Journal of Selected Topics in Applied Earth Observations and Remote Sensing(2020)
关键词
Boundary-aware,fully convolutional networks (FCNs),remote sensing images,semantic segmentation,semisupervised learning
AI 理解论文
溯源树
样例
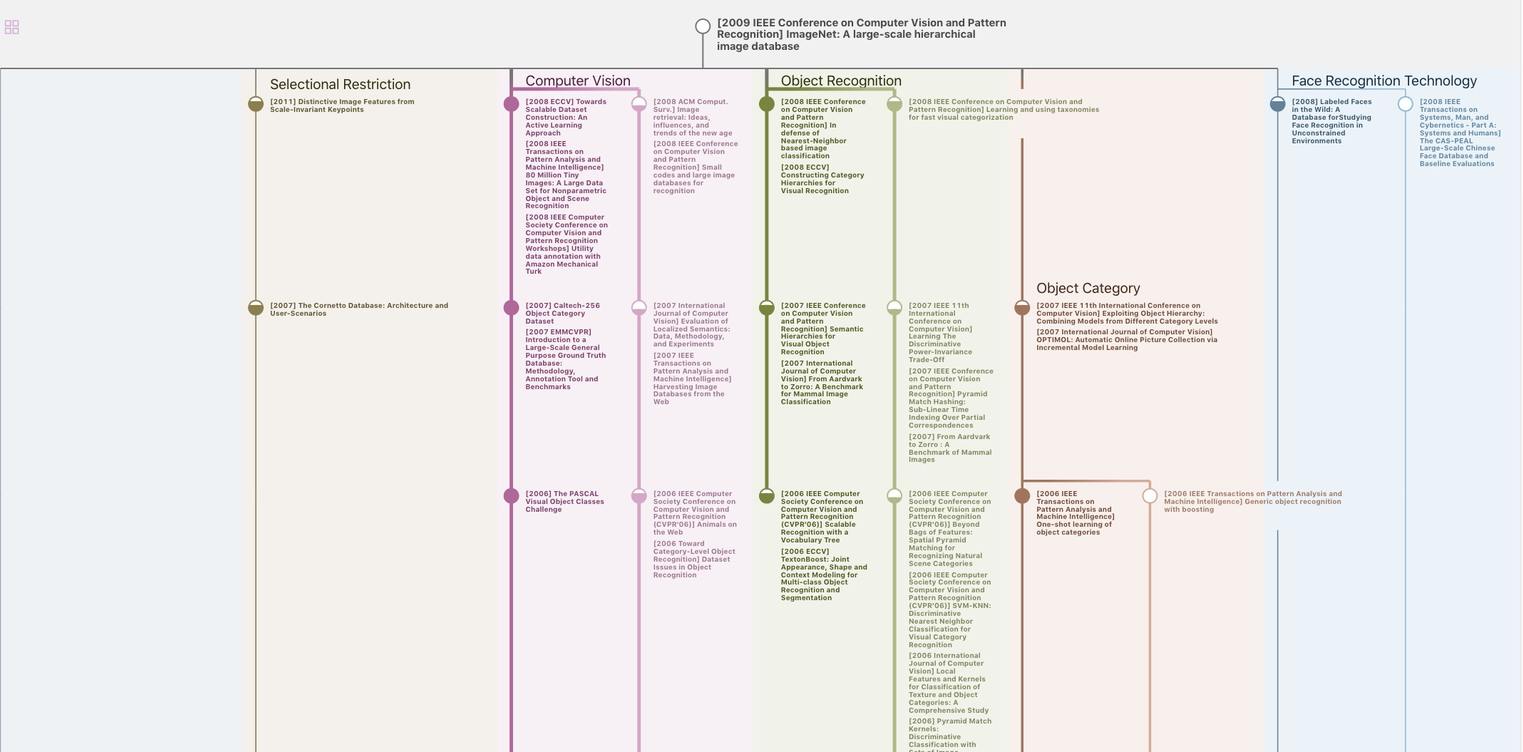
生成溯源树,研究论文发展脉络
Chat Paper
正在生成论文摘要