Frequency Domain Joint-Normalized Stochastic Gradient Projection-Based Algorithm for Widely Linear Quaternion-Valued Adaptive Filtering
IEEE TRANSACTIONS ON SIGNAL PROCESSING(2023)
摘要
The widely linear quaternion-valued least-mean-squares (WLQ-LMS) algorithm in the time domain tends to slow down its convergence when dealing with highly correlated or non-circular input signals. To address such a problem while reducing the computational complexity, in this article, we develop an adaptive frequency domain widely linear quaternion-valued joint-normalized stochastic gradient projection-based (FDWLQ-NGP) algorithm, which comprises gradient update and projection steps. In the first step, our joint-normalization design using joint second-order statistical information of ortho-split representation of quaternion aids the algorithm's convergence speed. As a comparison, an algebraic real translation-based algorithm for widely linear quaternion-valued adaptive filtering is also implemented in the frequency domain using the joint-normalization approach. Next, mean and mean-square analysis of a periodic update FDWLQ-NGP (P-FDWLQ-NGP) algorithm is conducted, which reveals the effect of the update interval and regularization parameters on the algorithm performance. By introducing a variable regularization mechanism for the P-FDWLQ-NGP algorithm, the steady-state errors are further reduced, along with attaining fast convergence. Finally, computer simulation results demonstrate that the proposed approaches outperform the WLQ-LMS, quaternion augmented affine projection and subband widely linear quaternion algorithms in terms of convergence speed, steady-state error, and computational complexity.
更多查看译文
关键词
Frequency domain,quaternion adaptive filter,widely linear,gradient projection,variable regularization,mean-square analysis
AI 理解论文
溯源树
样例
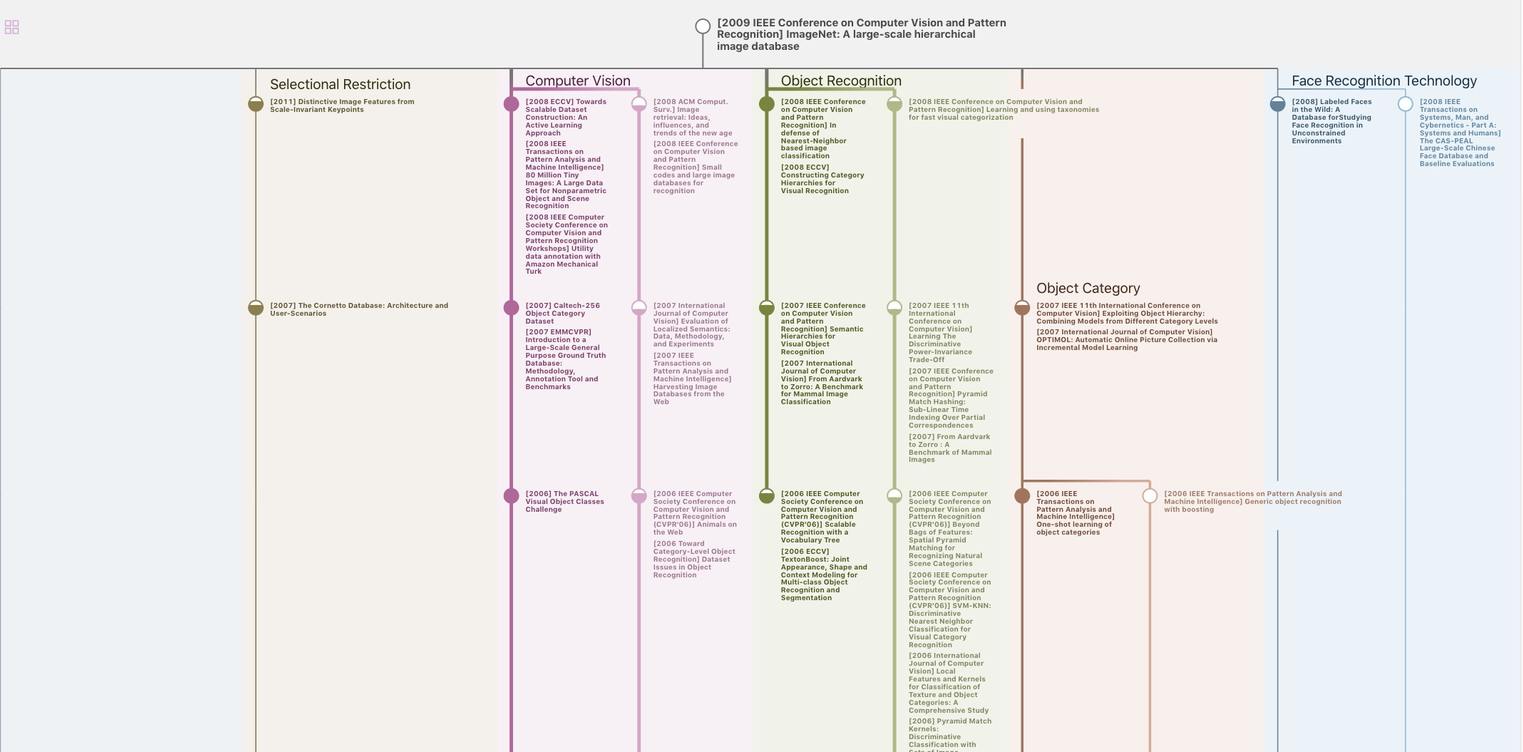
生成溯源树,研究论文发展脉络
Chat Paper
正在生成论文摘要