NU$^{2}$ P-Based Reversible Data Hiding in the Multi-Histogram Modification Framework
IEEE Signal Processing Letters(2023)
摘要
This letter aims to propose an advanced predictor called NU
$^{2}$
P in the multiple histogram modification (MHM)-based framework by combining deep learning techniques that take the local characteristics of pixels into account. NU
$^{2}$
P is firstly designed for reversible data hiding by incorporating U
$^{2}$
P (an improved version of U
$^{2}$
-Net) equipped with convolutional block attention module (CBAM). The purpose of U
$^{2}$
P is to integrate the feature maps with different sizes and receptive fields through U-Net-like residual U-blocks (RSUs), which can make full of strong correlations between adjacent pixels while reducing the computational cost by means of the pooling layers of RSUs. CBAM pays different attention to feature maps and elements of feature maps from the perspectives of channel and spatial attention, thereby helping NU
$^{2}$
P further enhance the prediction performance. In the MHM-based framework, for multiple categories generated using fuzzy C-means with multiple deliberately-designed features, NU
$^{2}$
P is conductive to constructing a sharp prediction error histogram (PEH) for each category and the improved discrete particle swarm optimization without significantly increasing the computational cost is used to adaptively select the optimal bins for each PEH. The experimental results show that the proposed method significantly outperforms several state-of-the-art RDH methods in terms of image quality and payload.
更多查看译文
关键词
Reversible data hiding (RDH),fuzzy C-means (FCM),multiple features,NU $^{2}$ P,CBAM,IDPSO
AI 理解论文
溯源树
样例
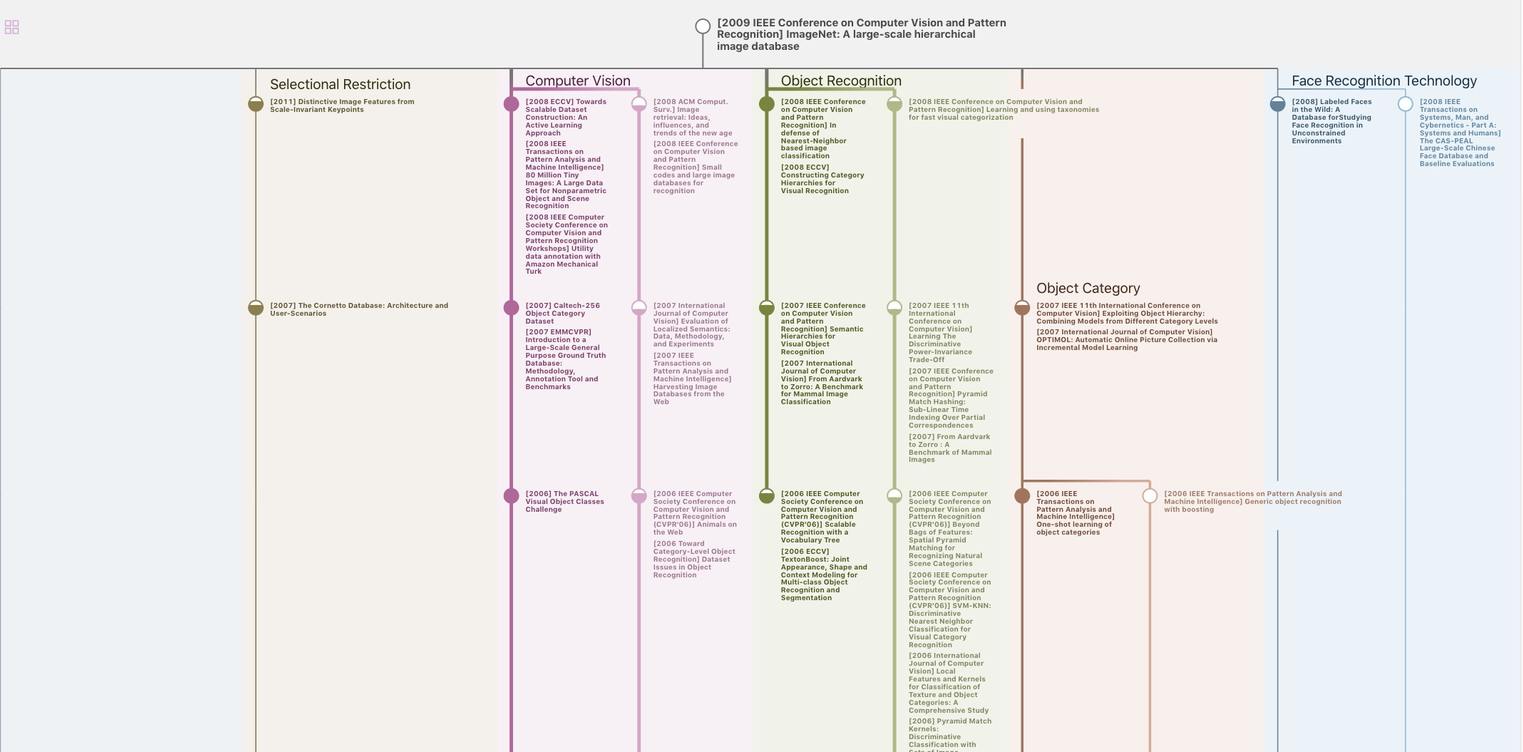
生成溯源树,研究论文发展脉络
Chat Paper
正在生成论文摘要