FDNet: Lightweight Train Image Fault Detection Network in Edge Computing Environments
IEEE Sensors Journal(2023)
摘要
The current train image fault detection model does not consider detection accuracy and speed, and its parameters and calculation amount are large. Consequently, the model cannot easily satisfy the requirements of the actual edge computing scenario. Therefore, this study proposes a lightweight train image fault detection network (FDNet) under an edge computing environment. FDNet makes three improvements based on YOLOX. First, FDNet designs dual-path enhanced channel attention (DECA) module and fuses the path aggregation network (PAN) to form a DECA-PAN module to suppress background interference, highlight the object, and improve the feature fusion ability of the model. Second, focal loss and alpha-complete intersection over union (Alpha-CIoU) loss are used to optimize the loss function to alleviate the problem of small image samples and uneven fault categories. Third, the receptive field fusion block is added to the FDNet detection head, and three groups of detection heads are devised to detect objects at different scales to improve the detection ability of the model on multiscale objects. Experimental results on the train image fault dataset demonstrate that the mean average precision (mAP) and speed of FDNet reach 94.82% and 103.4 frames per second (FPS), respectively, whereas its parameters and floating-point operations are less than one-sixth those of the well-known YOLOv4. The experimental results of FDNet, YOLOv5-s, YOLOX-s, YOLOv6-T, and YOLOv7-tiny in the public dataset COCO2017 show that FDNet achieves the highest mAP of 60.60% while maintaining a high detection speed among all lightweight models. The noise and illumination robustness tests show that FDNet has good anti-interference ability.
更多查看译文
关键词
Edge computing, fault detection, lightweight model, object detection
AI 理解论文
溯源树
样例
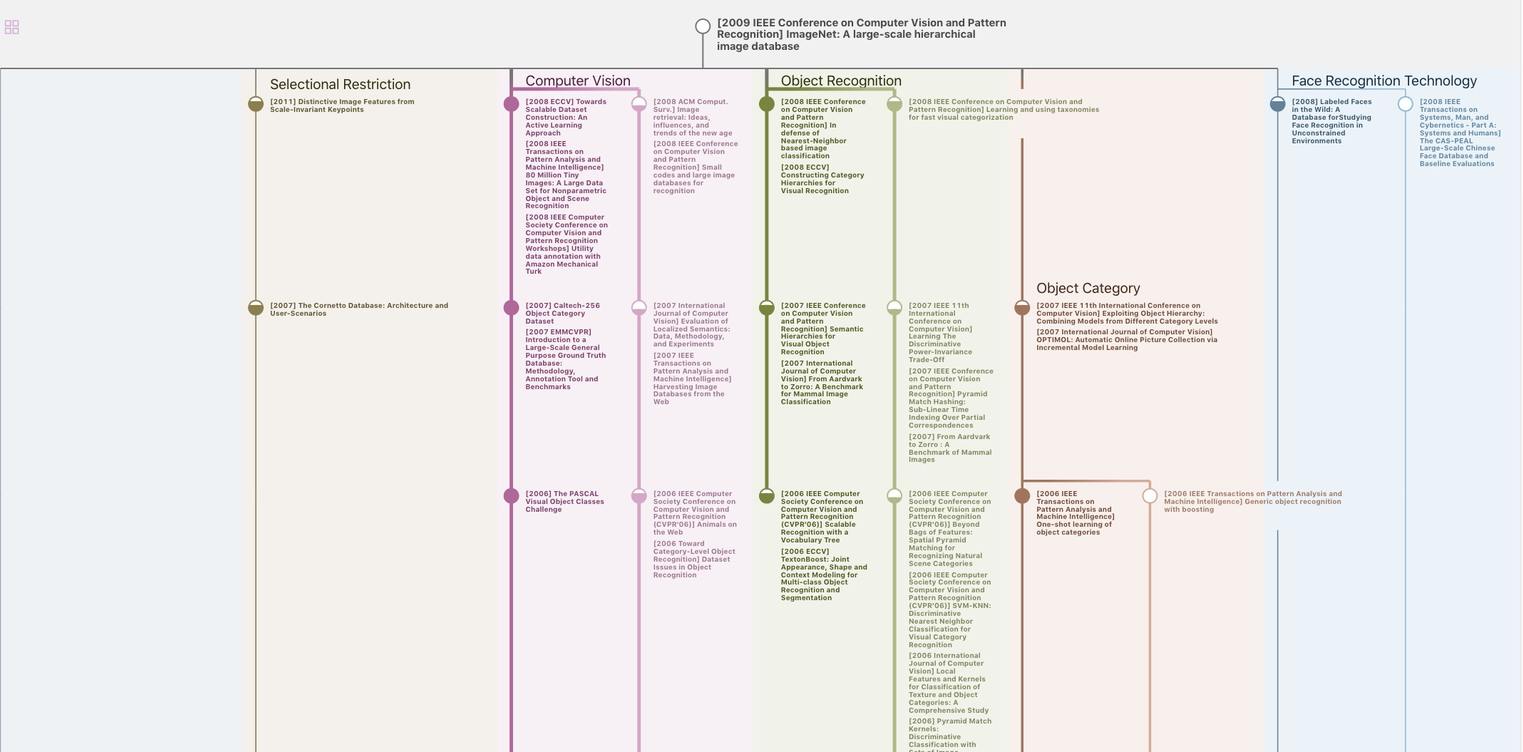
生成溯源树,研究论文发展脉络
Chat Paper
正在生成论文摘要