POLO: Learning Exp licit Cro ss-Modal ity Fusio n for Temporal Action Localization
IEEE Signal Processing Letters(2021)
摘要
Temporal action localization aims at discovering action instances in untrimmed videos, where RGB and flow are two widely used feature modalities. Specifically, RGB chiefly reveals appearance and flow mainly depicts motion. Given RGB and flow features, previous methods employ the early fusion or late fusion paradigm to mine the complementarity between them. By concatenating raw RGB and flow features, the early fusion implicitly achieved complementarity by the network, but it partly discards the particularity of each modality. The late fusion independently maintains two branches to explore the particularity of each modality, but it only fuses the localization results, which is insufficient to mine the complementarity. In this work, we propose ex
p
licit cr
o
ss-moda
l
ity fusi
o
n (POLO) to effectively utilize the complementarity between two modalities and thoroughly explore the particularity of each modality. POLO performs cross-modality fusion via estimating the attention weight from RGB modality and employing it to flow modality (vice versa). This bridges the complementarity of one modality to supply the other. Assisted with the attention weight, POLO independently learns from RGB and flow features and explores the particularity of each modality. Extensive experiments on two benchmarks demonstrate the preferable performance of POLO.
更多查看译文
关键词
Feature fusion,frame-wise attention,mutual attention,temporal action localization
AI 理解论文
溯源树
样例
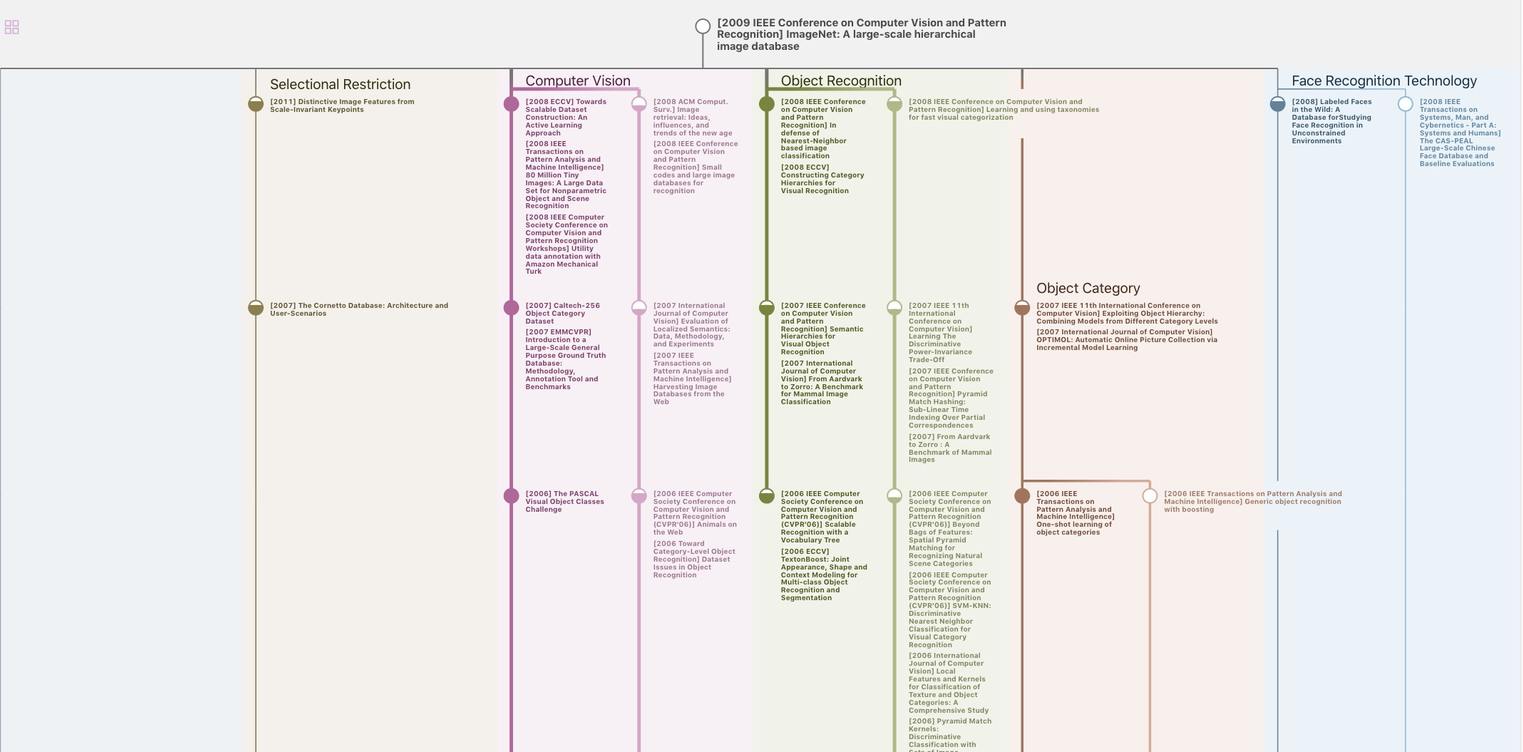
生成溯源树,研究论文发展脉络
Chat Paper
正在生成论文摘要