The Effect of IMU Sensor Location, Number of Features, and Window Size on a Random Forest Models Accuracy in Predicting Joint Kinematics and Kinetics During Gait
IEEE SENSORS JOURNAL(2023)
摘要
Using inertial measurement units (IMUs) in combination with machine learning (ML) techniques has become a common approach for predicting specific gait time series, such as lower limb joint kinematics and kinetics. However, the optimal number of IMUs, sensor placement, number of features, and window size applicable for predicting all gait time series has not been previously determined. This study aimed to investigate how a random forest (RF) model's performance can be affected by different combinations of IMU location, the number of features, and window sizes used for feature extraction. We recorded optical motion capture marker trajectories, ground reaction forces (GRFs), and seven IMUs data from 20 participants during gait. Joint angles and moments were calculated using OpenSim inverse kinematics (IKs) and dynamics tools from the marker's trajectory and GRFs. For different combinations of IMU numbers and locations, the most important features to predict each target (joint kinematics and kinetics) were extracted and used to build RF models. We showed that using only one pair of IMUs placed on the feet was as accurate as using seven IMUs when combined with an RF model to predict gait time series. Once the best IMU combination was determined, we explored the effect of the number of features and window size on RF model performance. A multioutput RF model with ten features per target and a window size of 0.75 s outperformed other models by providing lower prediction error for predicting most gait time series.
更多查看译文
关键词
Feature engineering,gait,inertial measurement units (IMUs),machine learning (ML),random forest (RF)
AI 理解论文
溯源树
样例
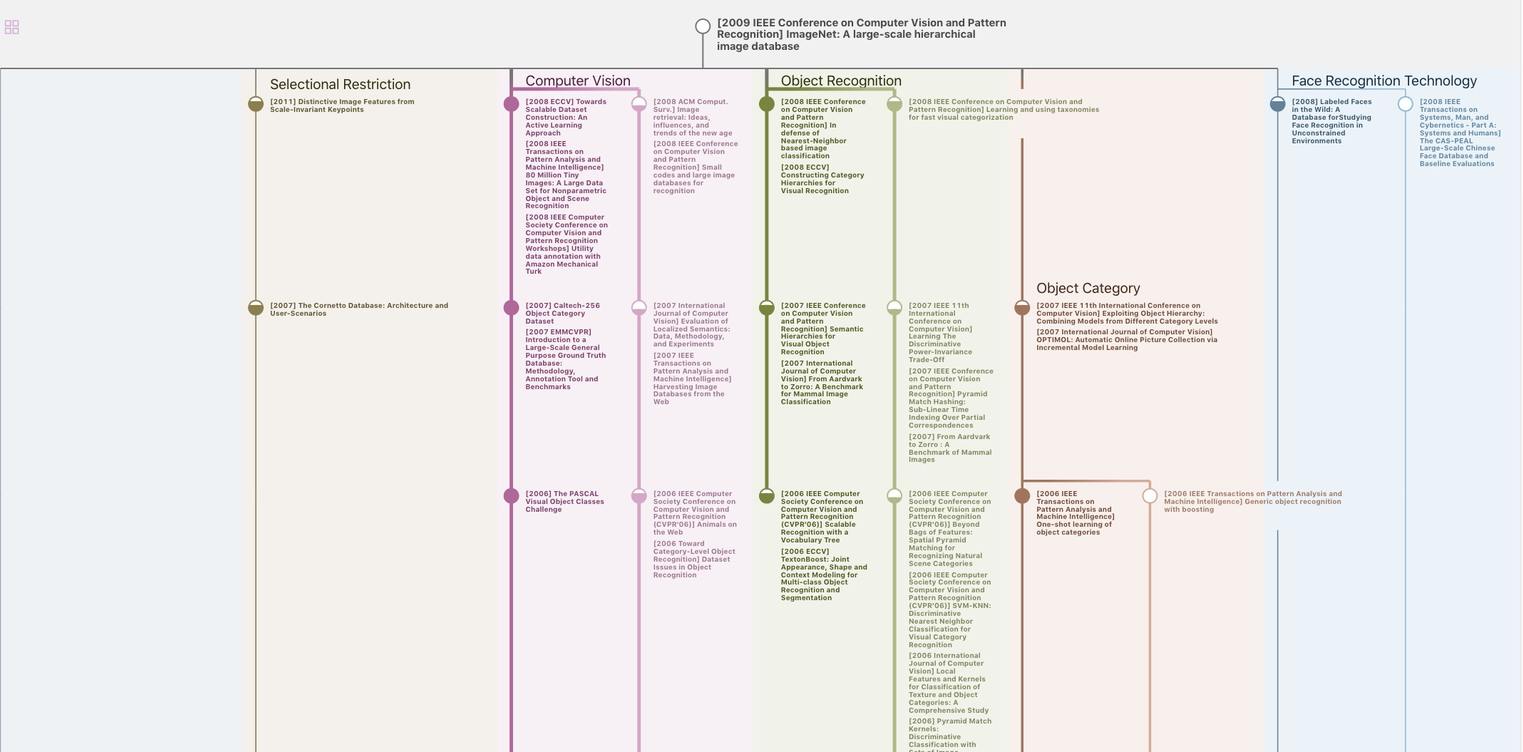
生成溯源树,研究论文发展脉络
Chat Paper
正在生成论文摘要