LTReID: Factorizable Feature Generation With Independent Components for Long-Tailed Person Re-Identification
IEEE Transactions on Multimedia(2023)
Abstract
With the rapid increase of large-scale and real-world person datasets, it is crucial to address the problem of long-tailed data distributions,
i
.
e
., head classes have large number of images while tail classes occupy extremely few samples. We observe that the imbalanced data distribution is likely to distort the overall feature space and impair the generalization capability of trained models. Nevertheless, this long-tailed problem has been rarely investigated in previous person Re-Identification (ReID) works. In this paper, we propose a novel
Long-Tailed Re-Identification
(LTReID) framework to simultaneously alleviate class-imbalance and hard-imbalance problems. Specifically, each real feature is decomposed into multiple independent components with two decorrelation losses. Then these components are randomly aggregated to generate more fake features for tail classes than head ones, resulting in the class-balance between head and tail classes. For the hard-balance between easy and hard samples, we utilize adversarial learning to generate more hard features than easy ones. The proposed framework can be trained in an end-to-end manner and avoids increasing the space and time complexity of inference models. Moreover, comprehensive experiments are conducted on the four ReID datasets so as to validate the effectiveness of the overall framework and the advantage of each module. Our results show that when trained with either balanced or imbalanced datasets, the LTReID achieves superior performance over the state-of-the-art methods.
MoreTranslated text
Key words
factorizable feature generation,independent components,person,long-tailed,re-identification
AI Read Science
Must-Reading Tree
Example
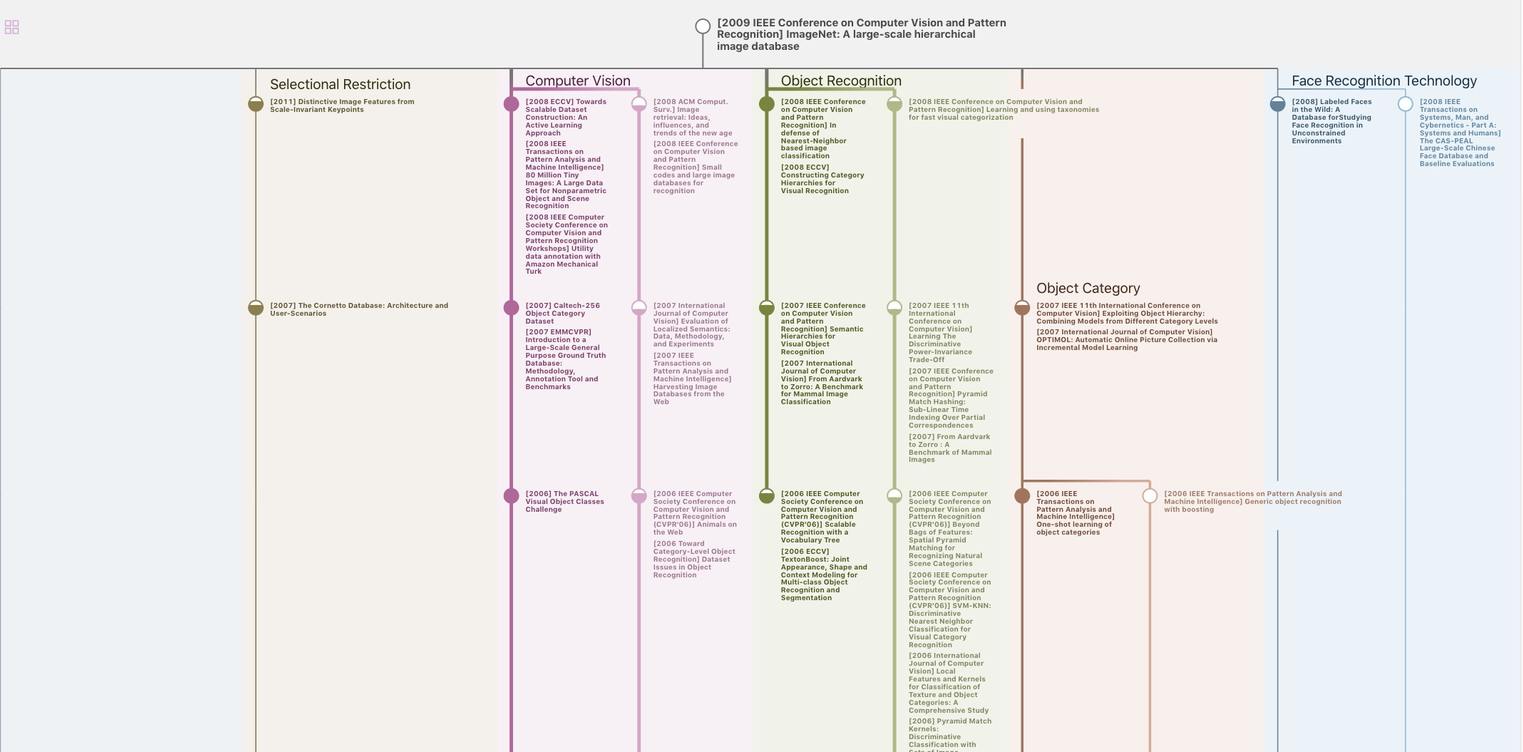
Generate MRT to find the research sequence of this paper
Chat Paper
Summary is being generated by the instructions you defined