Hybrid Model-Driven Spectroscopic Network for Rapid Retrieval of Turbine Exhaust Temperature
IEEE TRANSACTIONS ON INSTRUMENTATION AND MEASUREMENT(2023)
Abstract
Exhaust gas temperature (EGT) is a key parameter in diagnosing the health of gas turbine engines (GTEs). In this article, we propose a model-driven spectroscopic network with strong generalizability to monitor the EGT rapidly and accurately. The proposed network relies on data obtained from a well-proven temperature measurement technique, i.e., wavelength modulation spectroscopy (WMS), with the novelty of introducing an underlying physical absorption model and building a hybrid dataset from simulation and experiment. This hybrid model-driven (HMD) network enables strong noise resistance of the neural network against real-world experimental data. The proposed network is assessed by in situ measurements of EGT on an aero-GTE at millisecond-level temporal response. Experimental results indicate that the proposed network substantially outperforms previous neural-network methods in terms of accuracy and precision of the measured EGT when the GTE is steadily loaded.
MoreTranslated text
Key words
Deep neural network (DNN),exhaust gas temperature (EGT),gas turbine engine (GTE),signal processing,wavelength modulation spectroscopy (WMS)
AI Read Science
Must-Reading Tree
Example
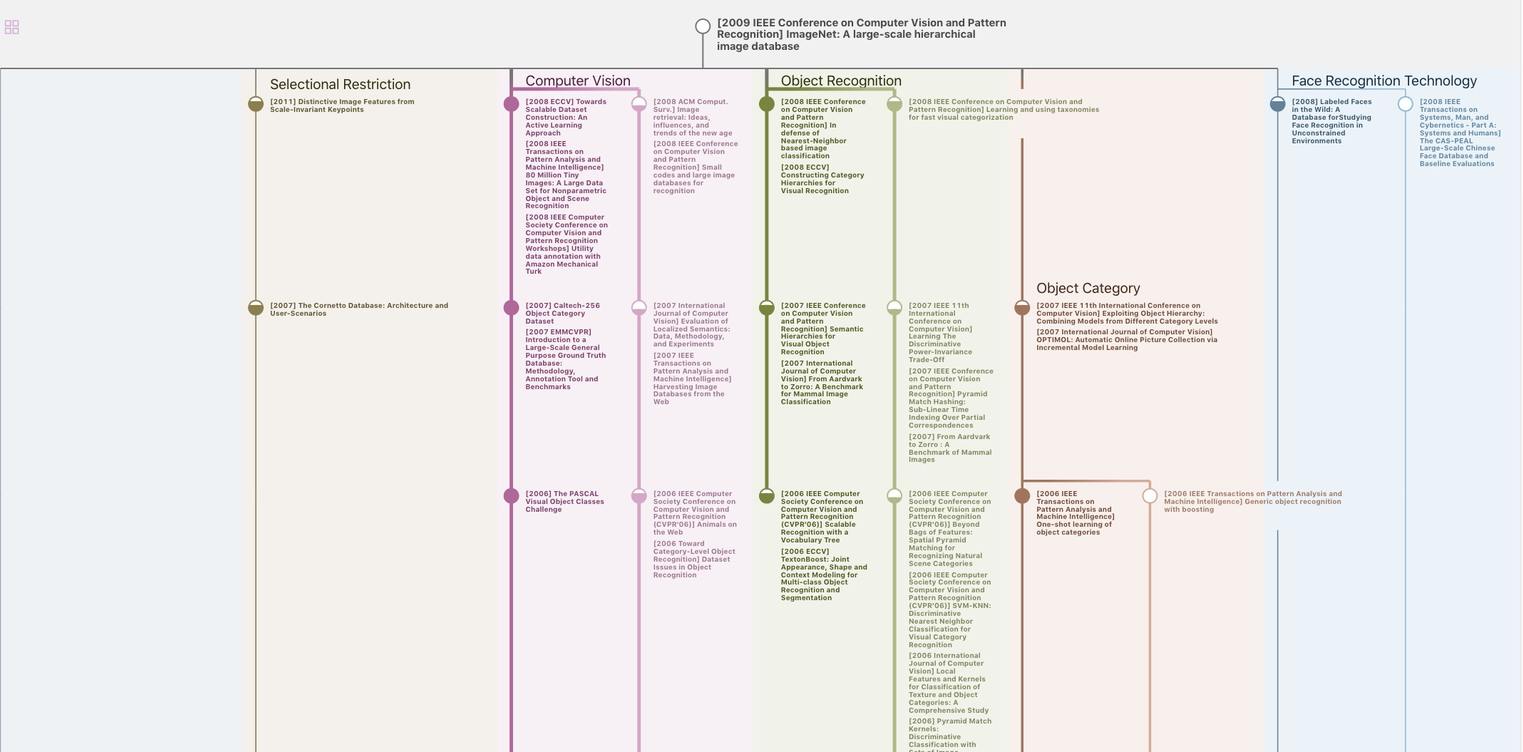
Generate MRT to find the research sequence of this paper
Chat Paper
Summary is being generated by the instructions you defined