Adaptive Two-Stage Multisensor Convolutional Autoencoder Model for Lossy Compression of Hyperspectral Data
IEEE TRANSACTIONS ON GEOSCIENCE AND REMOTE SENSING(2023)
摘要
The growing availability of hyperspectral remote sensing data, specifically from the new hyperspectral satellite missions, requires efficient data compression due to limitations in bandwidth and available storage space while simultaneously preserving the spectral characteristics. Machine learning approaches are a powerful way to address this challenge, but they are usually tailored to only work on one specific sensor. This work addresses the challenge of transferability of autoencoder (AE) models for lossy compression of spatially independent and unknown hyperspectral datasets acquired from different sensor platforms. We propose the CompNext1D, an advanced multistage adaptive network based on the architecture of the adaptive 1-D convolutional AE (A1D-CAE). The characteristic of the A1D-CAE allows pretraining on a large dataset with wide spectral variability and transferability to other sensor data, e.g., with potentially limited data availability. The compression performance of the CompNext1D is enhanced by using image statistics and shows a high degree of transferability to unknown spectral signatures. We evaluate the reconstruction accuracy with three experiments of increasing complexity. The evaluation is based on the reconstruction accuracy using the spectral angle (SA), signal-to-noise ratio (SNR), peak SNR (PSNR), and structural similarity index measure (SSIM) metrics, and the results are compared to other learning-based lossy compression techniques. We demonstrate the high transferability and generalizability of our A1D-CAE and CompNext1D for compression rates from c(g) = 4 to c(g )approximate to 100 on hyperspectral data from different sensors and carrier platforms. The CompNext1D architecture performs well in compressing hyperspectral data from multiple sensor sources with different characteristics while achieving higher reconstruction accuracy compared to state-of-the-art methods.
更多查看译文
关键词
Autoencoder (AE),dimensionality reduction,entropy coding,feature extraction,hyperspectral imaging (HSI) compression,spectral analysis,spectral compression
AI 理解论文
溯源树
样例
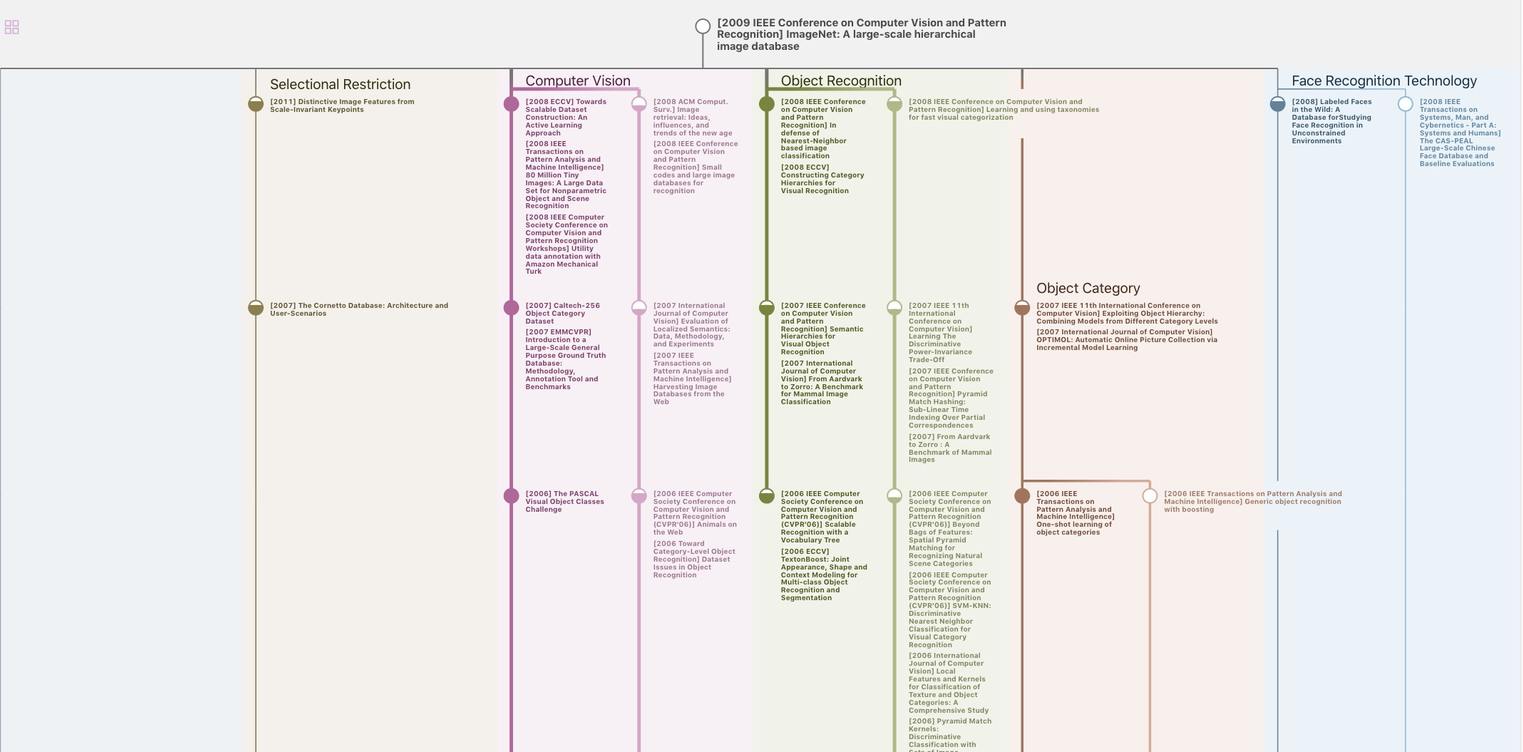
生成溯源树,研究论文发展脉络
Chat Paper
正在生成论文摘要