Few-Shot Hyperspectral Image Classification Using Meta Learning and Regularized Finetuning
IEEE TRANSACTIONS ON GEOSCIENCE AND REMOTE SENSING(2023)
Abstract
The use of deep learning (DL)-based hyperspectral image (HSI) classification has been made remarkable progress in recent years. However, obtaining sufficient labeled samples for training DL models remains a challenge. Transfer learning is effective in addressing the problem of HSI classification with limited labeled samples. However, cross-domain HSI classification using transfer learning remain difficult, as differences in ground object categories between two datasets make it challenging to transfer and learn accurate. To address this issue, we propose a simple yet effective method for HSI classification using model-agnostic meta-learning (MAML) and Regularized Fine-tuning (MRFSL). Our method uses optimized 3-D convolutional neural networks (3D-CNNs) model, aided by MAML and cutout data augmentation to enable cross-domain transfer learning and carry out the HSI classification with limited target samples. Experiments conducted on three HSI datasets demonstrate that the MRFSL method achieves excellent results compared to existing methods. Specifically, the overall accuracy (OA) of our proposed MRFSL method reached 91.81%, 71.04%, and 88.35%, when only five labeled samples for each category were randomly extracted from the Salinas, Indian Pines (IPs), and University of Pavia (UP) datasets, respectively.
MoreTranslated text
Key words
Cross-domain,few-shot learning (FSL),hyperspectral image (HSI) classification,meta-learning,regularized finetuning
AI Read Science
Must-Reading Tree
Example
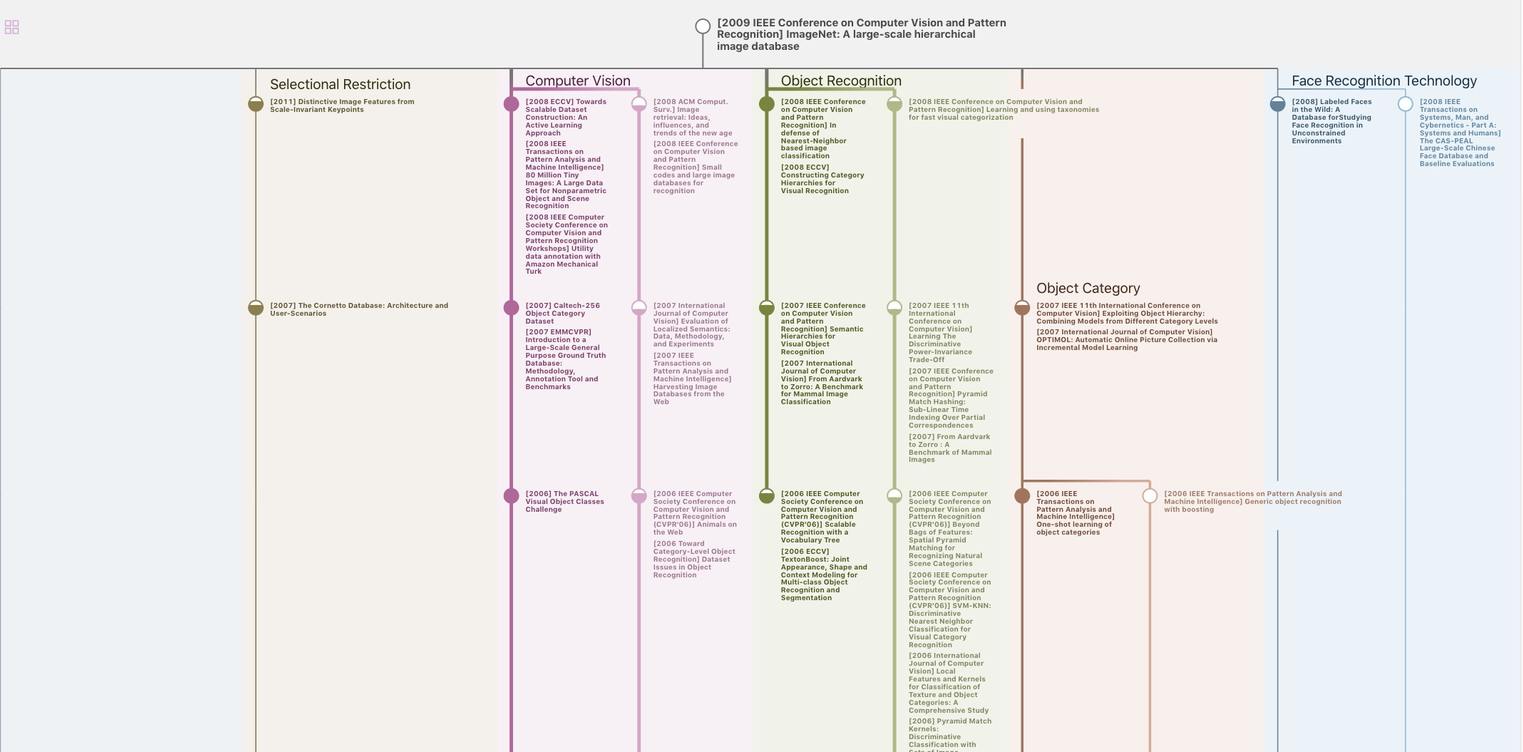
Generate MRT to find the research sequence of this paper
Chat Paper
Summary is being generated by the instructions you defined