Learning Transferable Discriminative Knowledge From Attribute-Aligned Hyperspectral Images
IEEE TRANSACTIONS ON GEOSCIENCE AND REMOTE SENSING(2023)
摘要
Hyperspectral image (HSI) classification faces the inherent challenge of small sample learning, primarily due to the difficulty in labeling vast land covers. Meta-learning, with its ability to learn transferable meta-knowledge from existing HSIs, is seen as a promising solution. However, different HSIs usually have varying distributions manifested as differing spectral wavelengths and reflectance shifts, which is often neglected in existing methods, stalling the acquisition of transferable features. To address this issue, we introduce an attribute-driven spectral alignment (ADSA) method, which parameterizes and embeds spectral attributes (i.e., spectral wavelengths and reflectance shifts) into a domain-adaptation model, aiming to decouple the domain-specific attributes of different HSIs in an unsupervised manner. After training, the parameterized attributes of source-domain (SD) HSIs can be substituted with those of the target domain (TD), allowing the decoder of ADSA to rebuild new HSIs sharing identical spectral attributes. By this means, numerous distribution-consistent labeled samples preserving inherent spectral-spatial structures can be obtained. Then, a 3-D residual prototypical network (RPN) based on 3-D convolutions and metric learning is designed to model complex structures of HSIs, which in combination with the few-shot learning (FSL) framework can extract valuable discriminative knowledge from these auxiliary samples. Finally, by applying this learned knowledge to the TD HSI, only a small number of labeled samples are required to obtain satisfactory performance. Extensive experiments on four real-world HSIs demonstrate the effectiveness of our method, and the performance outperforms several state-of-the-art methods.
更多查看译文
关键词
Attribute-driven spectral alignment (ADSA),cross domain,few-shot learning (FSL),hyperspectral image (HSI) classification,meta-learning
AI 理解论文
溯源树
样例
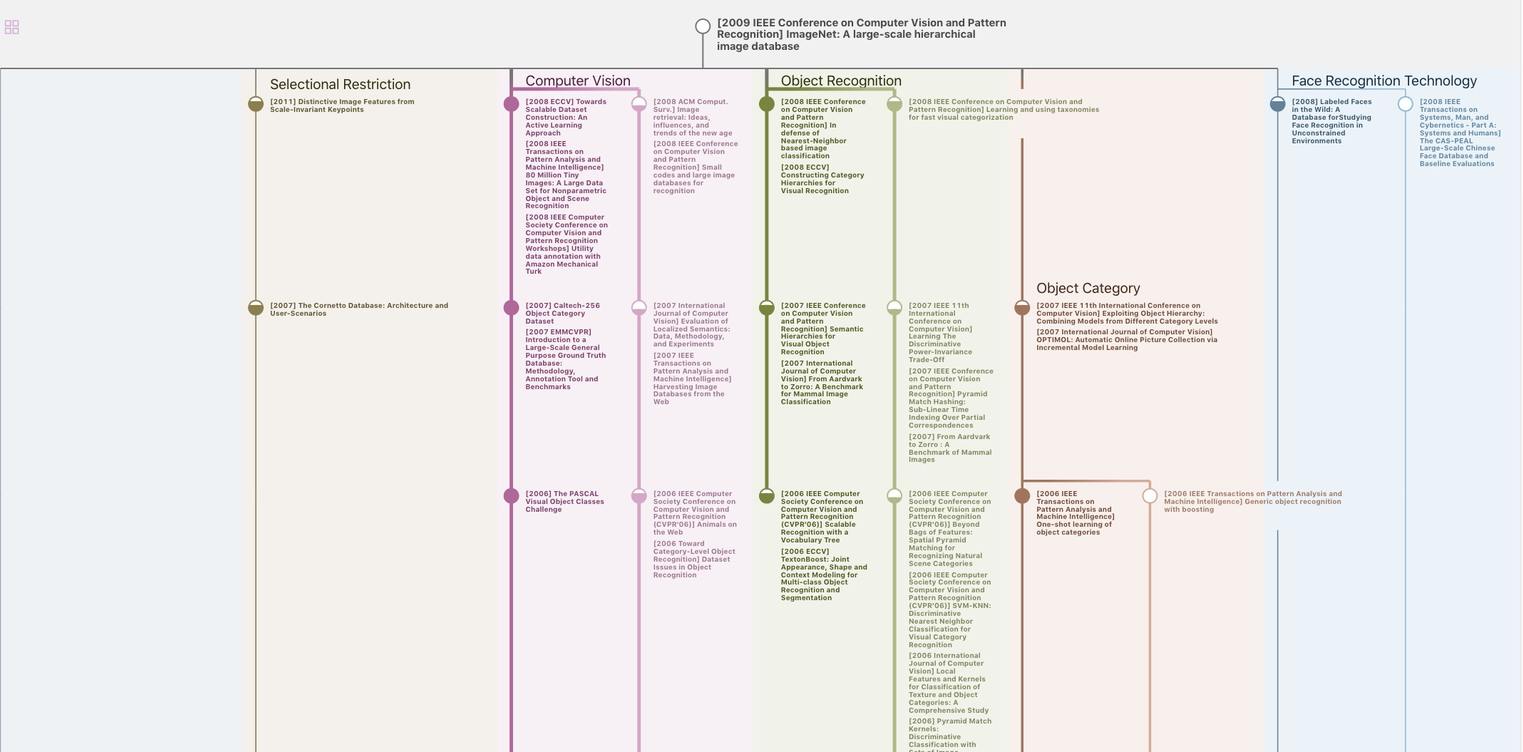
生成溯源树,研究论文发展脉络
Chat Paper
正在生成论文摘要