SSENet: A Multiscale 3-D Convolutional Neural Network for InSAR Shift Estimation
IEEE TRANSACTIONS ON GEOSCIENCE AND REMOTE SENSING(2023)
摘要
The interferometric synthetic aperture radar (InSAR) image shift measurement technique is of great significance in processing high-precision digital elevation model (DEM) generation and deformation measurements. It can be used in steps such as image fine coregistration, interferometric phase unwrapping (PU), and absolute phase calibration in the InSAR processing flow without an external DEM. However, the shifts estimated by current methods are of low resolution and have high measurement noise, which may have adverse impacts on subsequent applications. In this article, a lightweight, high-resolution, and low-noise interferometric stereo-radargrammetric shift estimation network (SSENet) is proposed to solve the aforementioned problems. It introduces deep-learning technology to the InSAR shift estimation task for the first time. We propose forming multiscale 3-D coherence coefficient cubes (CC-Cubes) by projecting the shift values of the images onto the third dimension and then using a 3-D convolutional network for multiscale fusion and encoding, followed by decoding with linear layers. In addition, a dataset generation and augmentation scheme based on real data is designed for model training and evaluation. Several sets of real SAR images from different regions of the world were used to evaluate SSENet. Compared with the typical coherent cross correlation (CCC) approach, SSENet reduces the mean absolute error (MAE) of the estimated shifts by approximately 79% while improving the resolution by a factor of 4 x 4 , making it possible to restore the absolute interferometric phase. Finally, we demonstrate a stitching strategy for processing large-scale SAR images and discuss the multiple potential uses of SSENet in the InSAR processing chain.
更多查看译文
关键词
Estimation,Azimuth,Radar polarimetry,Image resolution,Deformation,Three-dimensional displays,Task analysis,Deep learning,image coregistration,interferometric synthetic aperture radar (InSAR) processing,stereo-radargrammetry
AI 理解论文
溯源树
样例
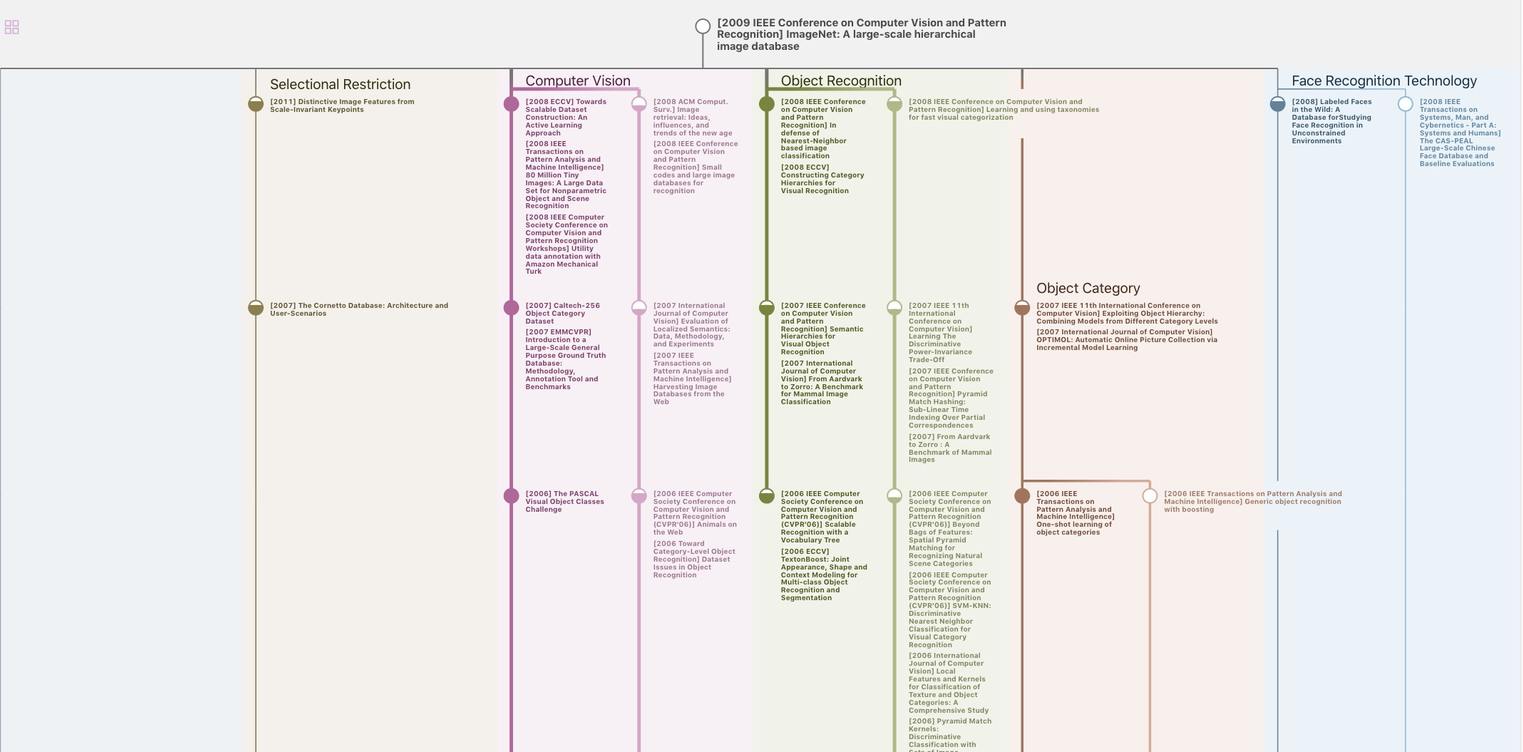
生成溯源树,研究论文发展脉络
Chat Paper
正在生成论文摘要