MHGAN: A Multi-Headed Generative Adversarial Network for Underwater Sonar Image Super-Resolution
IEEE TRANSACTIONS ON GEOSCIENCE AND REMOTE SENSING(2023)
摘要
Super-resolution (SR) is a technique for recovering image details based on available information, avoiding image quality degradation by increasing an image's resolution. Despite the great success of deep-learning-based SR models, they are rarely applied to underwater sonar scenarios due to the lack of underwater sonar datasets and the difficulty in recovering texture details. To address these challenges, we propose a multi-headed generative adversarial network (MHGAN) for the SR of underwater sonar images. The main body of the generator is designed with the introduced residual simple-dense self-calibrated block (RSSB), which combines self-calibrated attention and pixel attention to improve generalization capability. In addition, the discriminator employs a novel multi-headed U-net structure to acquire detailed texture information at multiple patch sizes of different scales, along with the generated image and high-resolution (HR) image details. Moreover, we introduce the correction loss, which acts as a pixel-level correction to help improve the quality of the output images, and multiperceptual loss based on VGG19 to extract multiscale features for comparison. Meanwhile, we also introduce the underwater sonar dataset for SR (USDSR). We perform the visual and quantitative comparison, ablation experiments, and model analysis with state-of-the-art methods on both the public KLSG dataset and the built USDSR dataset. Experimental results demonstrate the effectiveness and superiority of our proposed MHGAN model.
更多查看译文
关键词
Generative adversarial network (GAN),single-image super-resolution (SISR),underwater sonar image
AI 理解论文
溯源树
样例
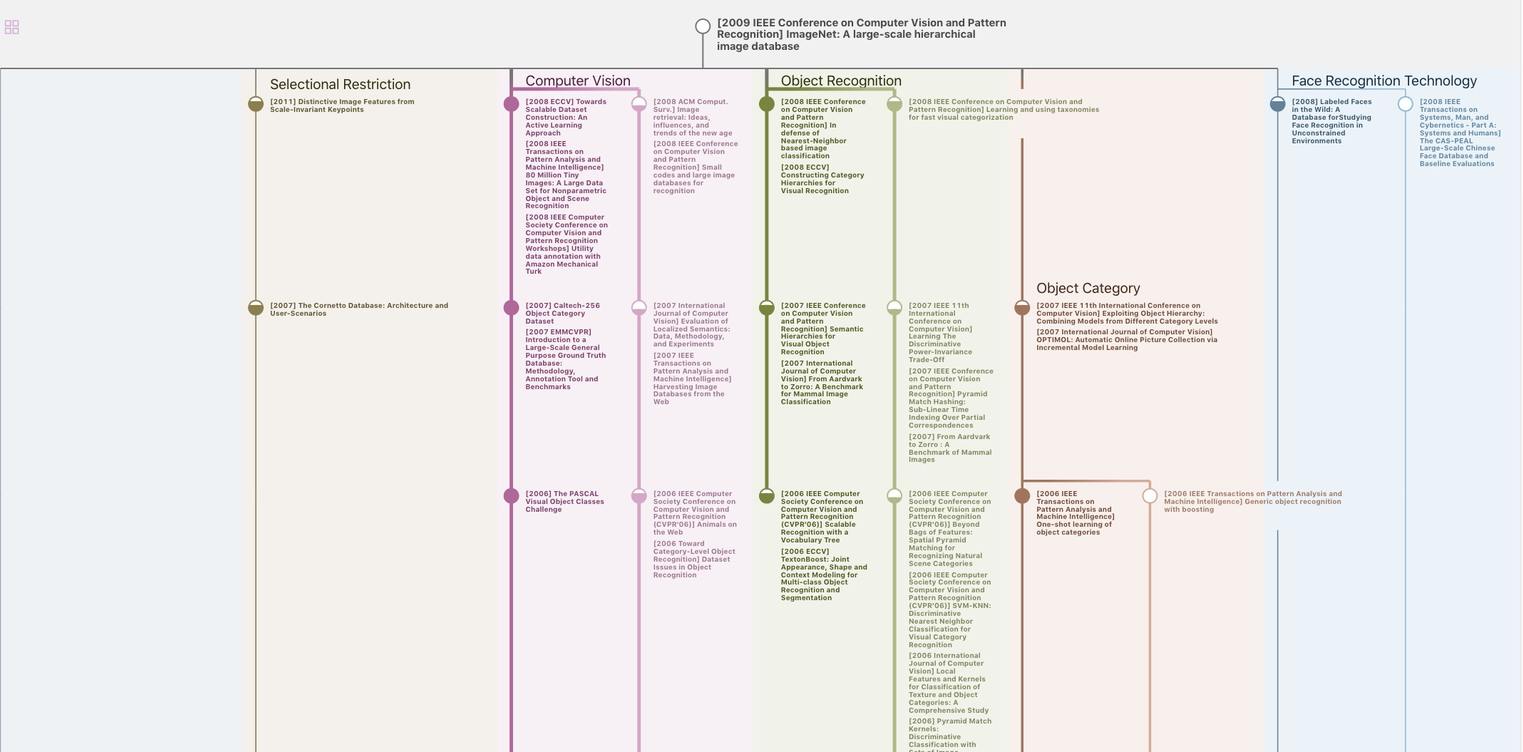
生成溯源树,研究论文发展脉络
Chat Paper
正在生成论文摘要