LPT-QPN: A Lightweight Physics-Informed Transformer for Quantitative Precipitation Nowcasting
IEEE TRANSACTIONS ON GEOSCIENCE AND REMOTE SENSING(2023)
Abstract
Quantitative precipitation nowcasting (QPN) is a highly challenging task in weather forecasting. The ability to provide precise, immediate, and detailed QPN products is necessary for a variety of situations, including storm warnings, air travel, and large gatherings. To address this challenge, this article proposes a new transformer lightweight physics-informed transformer (LPT)-QPN for QPN tasks, utilizing vertical cumulative liquid water content (VIL) products. This model adopts novel transformer modules to model the long-term evolution of precipitation and incorporates multihead squared attention (MHSA) to model its highly nonlinear relationships while reducing computational complexity. The results of experimental evaluations demonstrate the superiority of LPT-QPN when compared to existing state-of-the-art QPN models. In particular, the LPT-QPN model demonstrates greater accuracy for long lead time and in high-intensity areas, confirmed in both quantitative and qualitative evaluations. In addition, through three customized fine-tuning schemes, we are able to further improve the predictability of the LPT-QPN model for specific precipitation events. By incorporating the physical constraints of the convection-diffusion equation, our approach offers novel perspectives for future explorations that combine physical prior knowledge and deep-learning (DL) techniques.
MoreTranslated text
Key words
Computational modeling,Precipitation,Task analysis,Predictive models,Data models,Mathematical models,Transformers,Deep learning (DL),fine-tuning,physical constraints,quantitative precipitation nowcasting (QPN),transformer
AI Read Science
Must-Reading Tree
Example
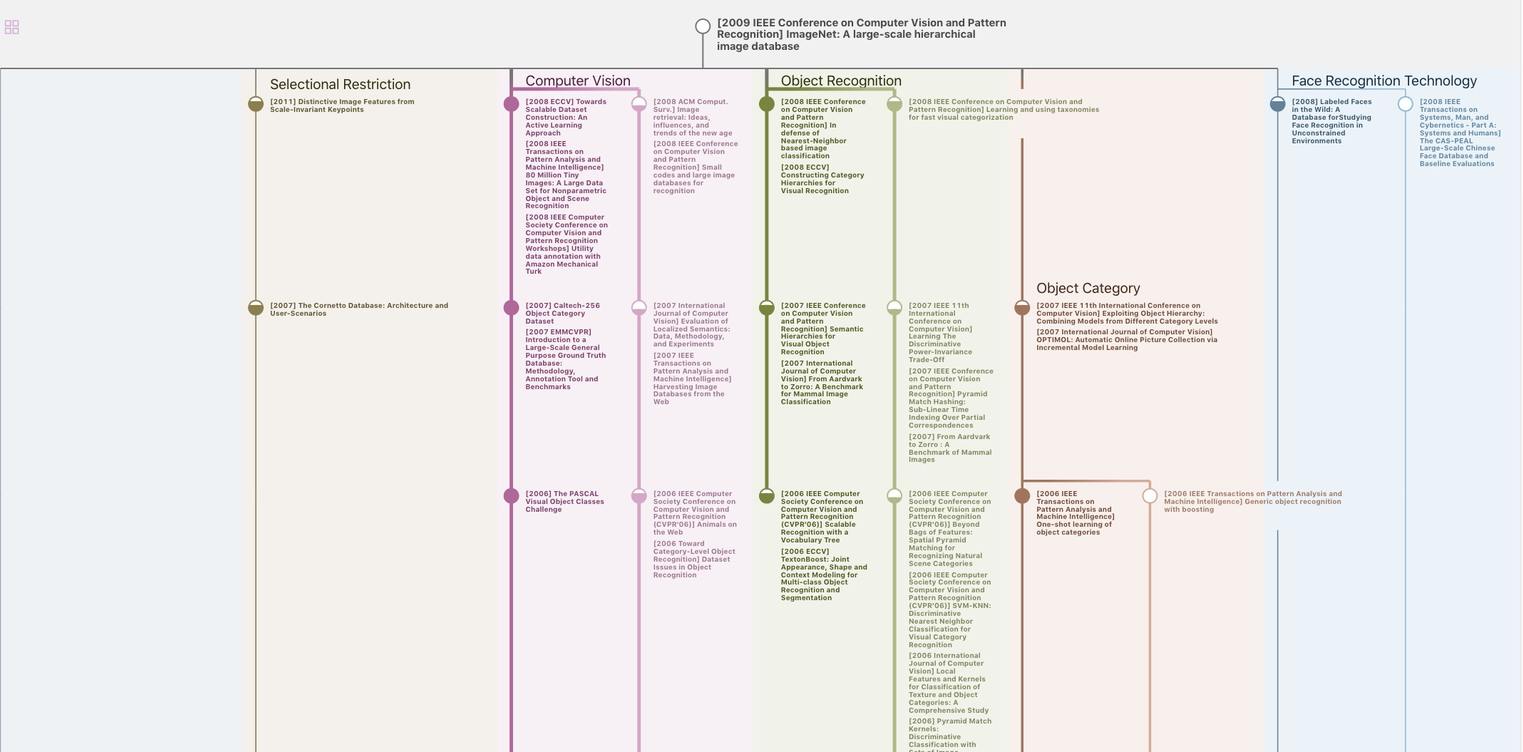
Generate MRT to find the research sequence of this paper
Chat Paper
Summary is being generated by the instructions you defined