A Deep Learning Solution for Height Inversion on Forested Areas Using Single and Dual Polarimetric TomoSAR
IEEE GEOSCIENCE AND REMOTE SENSING LETTERS(2023)
摘要
Forest characterization and monitoring are highly important for tracking climate change, using ecology resources, and biodiversity applications. Synthetic aperture radar tomography (TomoSAR) provides the opportunity to reconstruct 3-D structures of the penetrable media relying on multibaseline image acquisition. In forest applications, TomoSAR serves as a powerful technical tool for reconstructing forest height and underlying topography. Presently, a number of reconstruction methods are based on fully polarimetric (FP) TomoSAR (Pol-TomoSAR) datasets which require costly data acquisition. The aim of this letter is to go beyond the limitation of the requirement for full polarization by extending tomographic SAR neural network (TSNN), a neural network for TomoSAR, to the case of single-polarimetric (SP) and dual-polarimetric (DP) TomoSAR data for retrieving forest height and underlying topography. Experimental results indicate that TSNN trained by SP or DP TomoSAR data is a powerful candidate to estimate forest height and underlying topography with high accuracy.
更多查看译文
关键词
Forestry,Surfaces,Laser radar,Testing,Synthetic aperture radar,Image reconstruction,Geoscience and remote sensing,Climate change,Tracking,Deep learning (DL),forest height,polarimetry,synthetic aperture radar (SAR),tomography,underlying topography
AI 理解论文
溯源树
样例
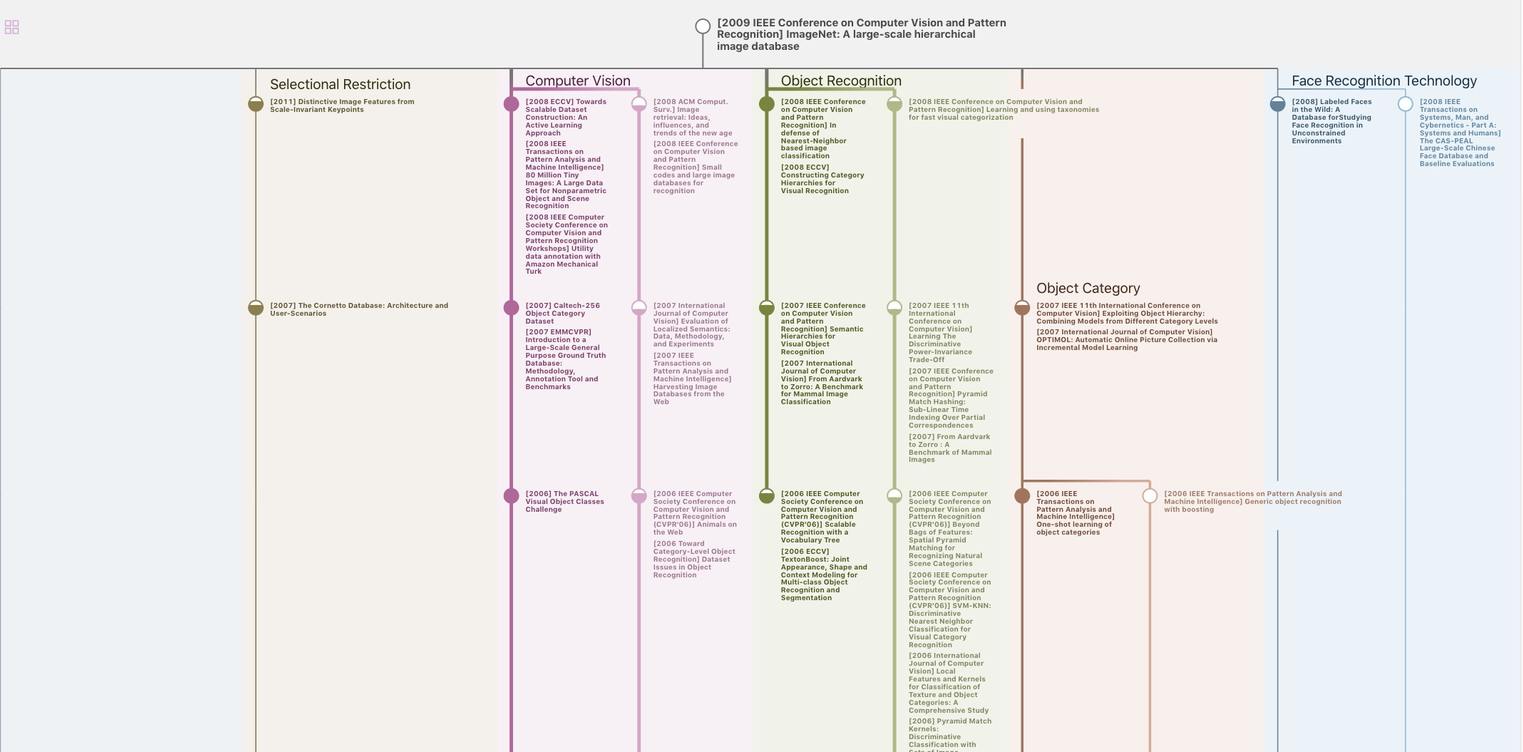
生成溯源树,研究论文发展脉络
Chat Paper
正在生成论文摘要